Few-Shot Object Detection by Second-order Pooling
Shan Zhang (ANU, Beijing Union University)*, Dawei Luo (Beijing Key Laboratory of Information Service Engineering, Beijing Union University ), Lei Wang ("University of Wollongong, Australia"), Piotr Koniusz (Data61/CSIRO, ANU)
Keywords: Applications of Computer Vision, Vision for X; Recognition: Feature Detection, Indexing, Matching, and Shape Representation; Statistical Methods and Learning
Abstract:
In this paper, we tackle a challenging problem of Few-shot Object Detection rather than recognition. We propose Power Normalizing Second-order Detector consisting of the Encoding Network (EN), the Multi-scale Feature Fusion (MFF), Second-order Pooling (SOP) with Power Normalization (PN), the Hyper Attention Region Proposal Network (HARPN) and Similarity Network (SN). EN takes support image crops and a query image per episode to produce covolutional feature maps across several layers while MFF combines them into multi-scale feature maps. SOP aggregates them per support image while PN detects the presence of visual feature instead of counting its frequency of occurrence. HARPN cross-correlates the PN pooled support features against the query feature map to match regions and produce query region proposals that are then aggregated with SOP/PN. Finally, support and query second-order descriptors are passed to SN. Our approach performs well because: (i) HARPN leverages SOP/PN for cross-correlation of detected rather than counted support features with query features which improves region proposals, (ii) SOP/PN capture second-order statistics per region proposal and factor out spatial locations, and (iii) PN limits the complexity of the space of functions over which HARPN and SN learn. These properties lead to the state of the art on the PASCAL VOC 2007/12, MS COCO and the FSOD datasets.
SlidesLive
Similar Papers
Mask-Ranking Network for Semi-Supervised Video Object Segmentation
Wenjing Li (University of Electronic Science & Technology of China)*, Xiang Zhang (University of Electronic Science & Technology of China), Yujie Hu (University of Electronic Science & Technology of China), Yingqi Tang (University of Electronic Science & Technology of China)
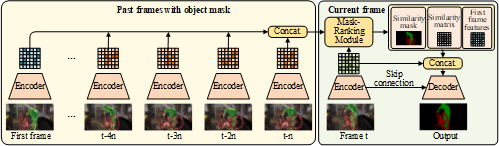
RF-GAN: A Light and Reconfigurable Network for Unpaired Image-to-Image Translation
Ali Koksal (Nanyang Technological University), Shijian Lu (Nanyang Technological University)*
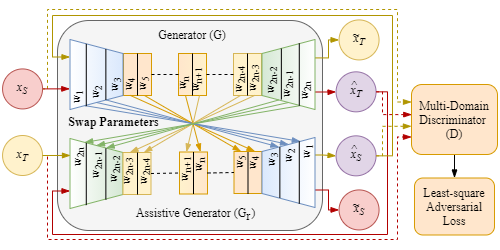
Jointly Discriminating and Frequent Visual Representation Mining
Qiannan Wang (Xidian university), Ying Zhou (Xidian University), ZhaoYan Zhu (Xidian university), Xuefeng Liang (Xidian University)*, Yu Gu (School of Artificial Intelligence, Xi'dian University)
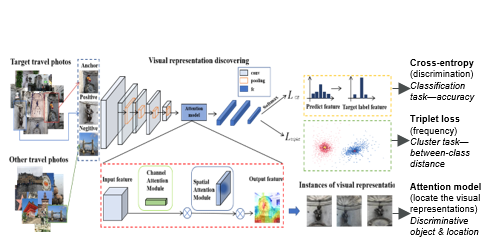