RF-GAN: A Light and Reconfigurable Network for Unpaired Image-to-Image Translation
Ali Koksal (Nanyang Technological University), Shijian Lu (Nanyang Technological University)*
Keywords: Generative models for computer vision
Abstract:
Generative adversarial networks (GANs) have been widely studied for unpaired image-to-image translation in recent years. On the other hand, state-of-the-art translation GANs are often constrained by large model sizes and inflexibility in translating across various domains. Inspired by the observation that the mappings between two domains are often approximately invertible, we design an innovative reconfigurable GAN (RF-GAN) that has a small size but is versatile in high-fidelity image translation either across two domains or among multiple domains. One unique feature of RF-GAN lies with its single generator which is reconfigurable and can perform bidirectional image translations by swapping its parameters. In addition, a multi-domain discriminator is designed which allows joint discrimination of original and translated samples in multiple domains. Experiments over eight unpaired image translation datasets (on various tasks such as object transfiguration, season transfer, and painters' style transfer, etc.) show that RF-GAN reduces the model size by up to 75% as compared with state-of-the-art translation GANs but produces superior image translation performance with lower Fréchet Inception Distance consistently.
SlidesLive
Similar Papers
Jointly Discriminating and Frequent Visual Representation Mining
Qiannan Wang (Xidian university), Ying Zhou (Xidian University), ZhaoYan Zhu (Xidian university), Xuefeng Liang (Xidian University)*, Yu Gu (School of Artificial Intelligence, Xi'dian University)
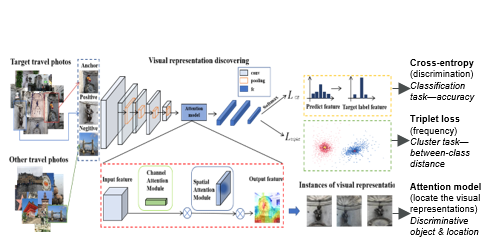
Few-Shot Object Detection by Second-order Pooling
Shan Zhang (ANU, Beijing Union University)*, Dawei Luo (Beijing Key Laboratory of Information Service Engineering, Beijing Union University ), Lei Wang ("University of Wollongong, Australia"), Piotr Koniusz (Data61/CSIRO, ANU)
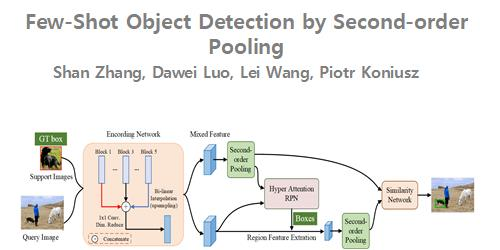
Low-light Color Imaging via Dual Camera Acquisition
Peiyao Guo (Nanjing University), Zhan Ma (Nanjing University)*
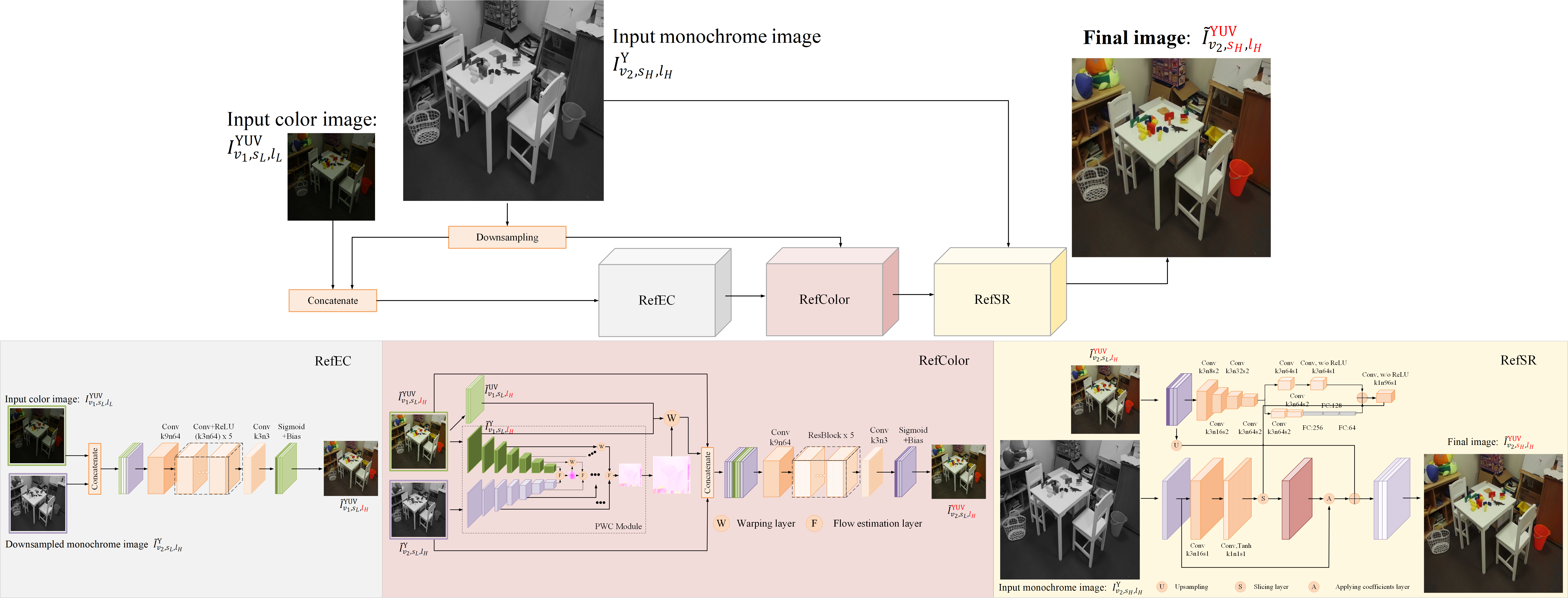