Mask-Ranking Network for Semi-Supervised Video Object Segmentation
Wenjing Li (University of Electronic Science & Technology of China)*, Xiang Zhang (University of Electronic Science & Technology of China), Yujie Hu (University of Electronic Science & Technology of China), Yingqi Tang (University of Electronic Science & Technology of China)
Keywords: Segmentation and Grouping
Abstract:
Video object segmentation is the fundamental problem of video analysis and many methods based on mask propagation and matching have been proposed in recent years. However, the two strategies are highly dependent on the last mask or the fixed mask given in the first frame and hence cannot adapt well to high deformation and rapid motion of objects. In this paper, we proposed a novel architecture named Mask-Ranking Network(MRNet), which takes advantage of both the propagation-based method and the matching-based method, to address the above problem. Specifically, in order to make better use of the long-term previous masks, we propose a novel propagation mechanism to make the network comprehensively consider the previous information. Under a unified encoder-decoder framework, we track the pixel-wise similarity of the object activation area in a long-term manner and explore the correlation between frames. In contrast to propagation-based only or matching-based only techniques, our method reduces the accumulation of errors in the propagation process and effectively uses the long-term previous frame information. In the video object segmentation task, MRNet can better handle the deformation of the objects, and make the segmentation result more accurate. We validate the effectiveness of the proposed method on the DAVIS 2016 and DAVIS 2017 dataset. Experiment results show that our method achieve state-of-the-art performance without using online fine-tuning and is robust to long-term propagation.
SlidesLive
Similar Papers
Few-Shot Object Detection by Second-order Pooling
Shan Zhang (ANU, Beijing Union University)*, Dawei Luo (Beijing Key Laboratory of Information Service Engineering, Beijing Union University ), Lei Wang ("University of Wollongong, Australia"), Piotr Koniusz (Data61/CSIRO, ANU)
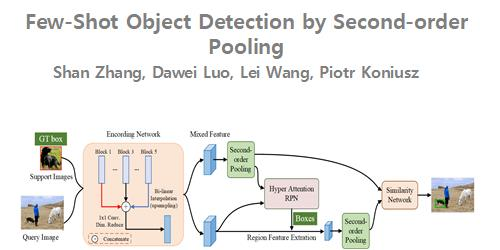
Point Proposal based Instance Segmentation with Rectangular Masks for Robot Picking Task
Satoshi Ito (Toshiba Corporation)*, Susumu Kubota (Toshiba Corporation)
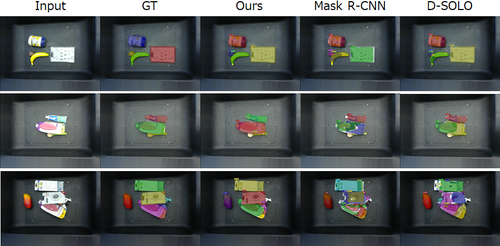
RF-GAN: A Light and Reconfigurable Network for Unpaired Image-to-Image Translation
Ali Koksal (Nanyang Technological University), Shijian Lu (Nanyang Technological University)*
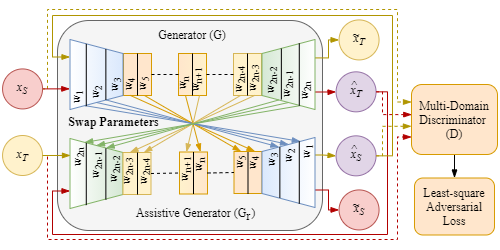