Show, Conceive and Tell: Image Captioning with Prospective Linguistic Information
Yiqing Huang (Tsinghua University), Jiansheng Chen (Tsinghua University)*
Keywords: Applications of Computer Vision, Vision for X
Abstract:
Attention based encoder-decoder models have achieved competitive performances in image captioning. However, these models usually follow the auto-regressive way during inference, meaning that only the previously generated words, namely the explored linguistic information, can be utilized for caption generation.Intuitively, enabling the model to conceive the prospective linguistic information contained in the words to be generated can be beneficial for further improving the captioning results. Consequently, we devise a novel Prospective information guided LSTM (Pro-LSTM) model, to exploit both prospective and explored information to boost captioning. For each image, we first draft a coarse caption which roughly describes the whole image contents. At each time step, we mine the prospective and explored information from the coarse caption. These two kinds of information are further utilized by a Prospective information guided Attention (ProA) module to guide our model to comprehensively utilize the visual feature from a semantically global perspective. We also propose an Attentive Attribute Detector (AAD) which refines the object features to predict the image attributes more precisely. This further improves the semantic quality of the generated caption. Thanks to the prospective information and more accurate attributes, the Pro-LSTM model achieves state-of-the-art performances on the MSCOCO dataset with a 129.5 CIDEr-D.
SlidesLive
Similar Papers
Image Captioning through Image Transformer
Sen He (University of Exeter)*, Wentong Liao (Leibniz University Hannover), Hamed R. Tavakoli (Nokia Technologies), Michael Yang (University of Twente), Bodo Rosenhahn (Leibniz University Hannover), Nicolas Pugeault (University of Glasgow)
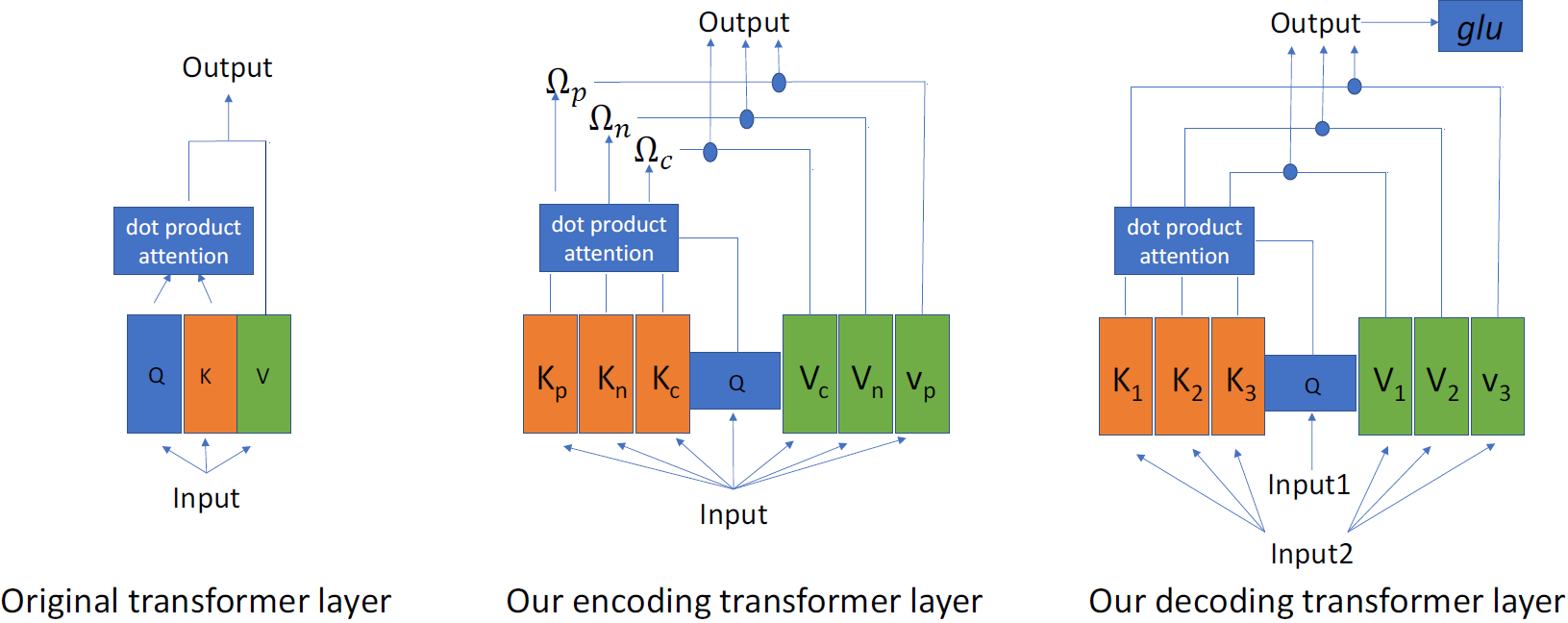
Query by Strings and Return Ranking Word Regions with Only One Look
Peng Zhao (Beijing Jiaotong University), Wenyuan Xue (Beijing Jiaotong University), Qingyong Li (Beijing Jiaotong University)*, Siqi Cai (Beijing Jiaotong University)
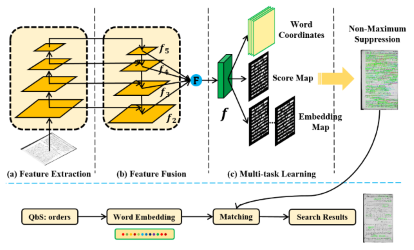
Multi-task Learning with Future States for Vision-based Autonomous Driving
Inhan Kim (POSTECH)*, Hyemin Lee (POSTECH), Joonyeong Lee (POSTECH), Eunseop Lee (POSTECH), Daijin Kim (Pohang University of Science and Technology)
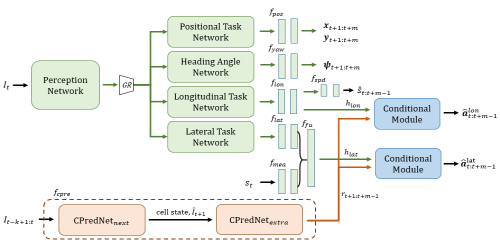