Multi-task Learning with Future States for Vision-based Autonomous Driving
Inhan Kim (POSTECH)*, Hyemin Lee (POSTECH), Joonyeong Lee (POSTECH), Eunseop Lee (POSTECH), Daijin Kim (Pohang University of Science and Technology)
Keywords: Applications of Computer Vision, Vision for X
Abstract:
Human drivers consider past and future driving environments to maintain stable control of a vehicle. To adopt a human driver's behavior, we propose a vision-based autonomous driving model, called Future Actions and States Network (FASNet), which uses predicted future actions and generated future states in multi-task learning manner. Future states are generated using an enhanced deep predictive-coding network and motion equations dened by the kinematic vehicle model. The nal control values are determined by the weighted average of thepredicted actions for a stable decision. With these methods, the proposed FASNet has a high generalization ability in unseen environments. To validate the proposed FASNet, we conducted several experiments, including ablation studies in realistic three-dimensional simulations. FASNet achieves a higher Success Rate (SR) on the recent CARLA benchmarks under several conditions as compared to state-of-the-art models.
SlidesLive
Similar Papers
Motion Prediction Using Temporal Inception Module
Tim Lebailly (EPFL)*, Sena Kiciroglu (EPFL (École polytechnique fédérale de Lausanne)), Mathieu Salzmann (EPFL), Pascal Fua (EPFL, Switzerland), Wei Wang (EPFL)
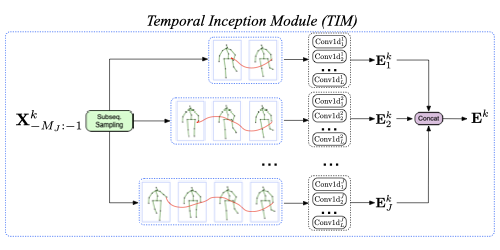
Adversarial Refinement Network for Human Motion Prediction
Xianjin Chao (The City University of Hong Kong)*, Yanrui Bin (HUST), Wenqing Chu (Tencent), Xuan Cao (Tencent), Yanhao Ge (Tencent), Chengjie Wang (Tencent), Jilin Li (Tencent), Feiyue Huang (Tencent), Howard Leung (City University of Hong Kong)
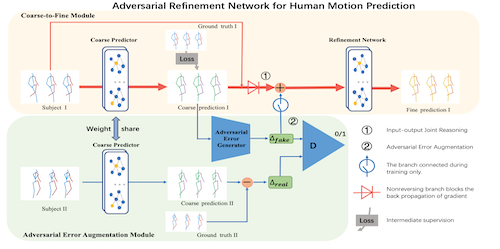
Image Captioning through Image Transformer
Sen He (University of Exeter)*, Wentong Liao (Leibniz University Hannover), Hamed R. Tavakoli (Nokia Technologies), Michael Yang (University of Twente), Bodo Rosenhahn (Leibniz University Hannover), Nicolas Pugeault (University of Glasgow)
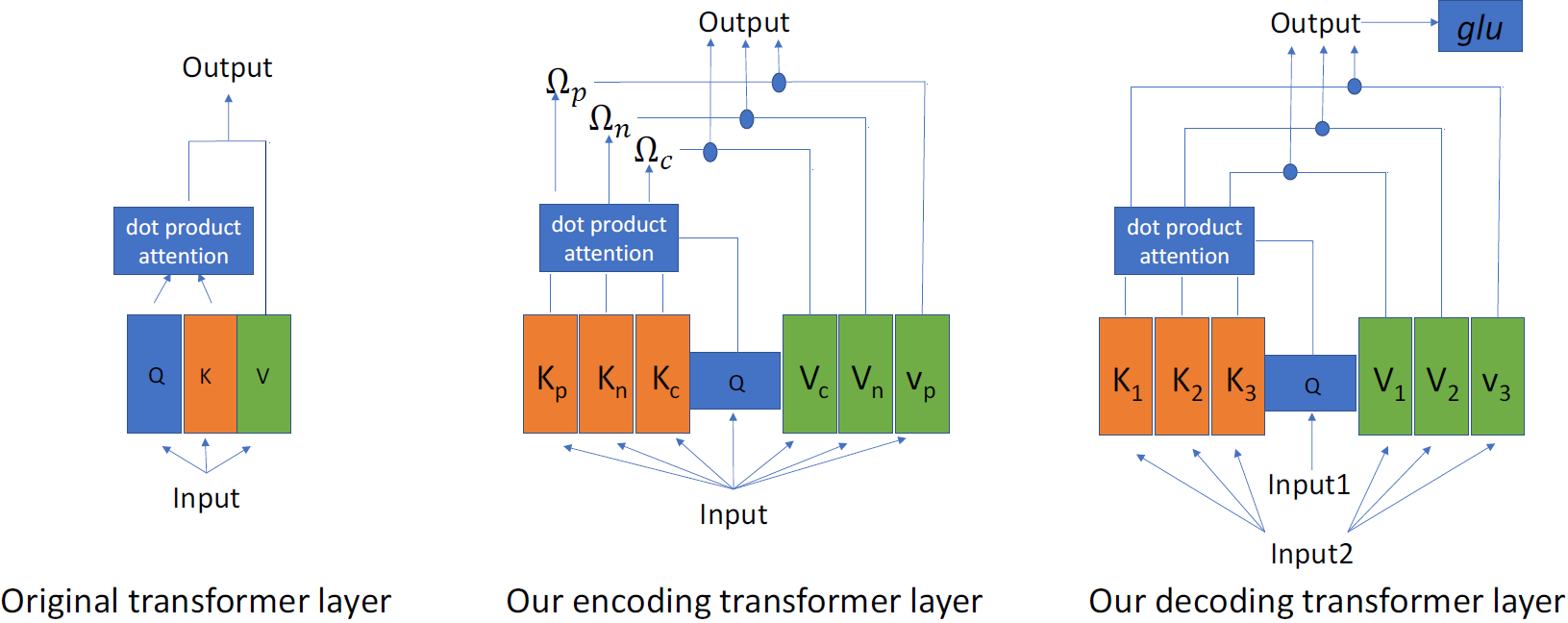