Motion Prediction Using Temporal Inception Module
Tim Lebailly (EPFL)*, Sena Kiciroglu (EPFL (École polytechnique fédérale de Lausanne)), Mathieu Salzmann (EPFL), Pascal Fua (EPFL, Switzerland), Wei Wang (EPFL)
Keywords: Motion and Tracking
Abstract:
Human motion prediction is a necessary component for many applications in robotics and autonomous driving. Recent methods propose using sequence-to-sequence deep learning models to tackle this problem. However, they do not focus on exploiting different temporal scales for different length inputs. We argue that the diverse temporal scales are important as they allow us to look at the past frames with different receptive fields, which can lead to better predictions. In this paper, we propose a Temporal Inception Module (TIM) to encode human motion. Making use of TIM, our framework produces input embeddings using convolutional layers, by using different kernel sizes for different input lengths. The experimental results on standard motion prediction benchmark datasets Human3.6M and CMU motion capture dataset show that our approach consistently outperforms the state of the art methods.
SlidesLive
Similar Papers
Adversarial Refinement Network for Human Motion Prediction
Xianjin Chao (The City University of Hong Kong)*, Yanrui Bin (HUST), Wenqing Chu (Tencent), Xuan Cao (Tencent), Yanhao Ge (Tencent), Chengjie Wang (Tencent), Jilin Li (Tencent), Feiyue Huang (Tencent), Howard Leung (City University of Hong Kong)
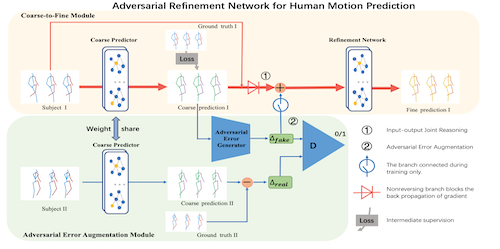
Multi-task Learning with Future States for Vision-based Autonomous Driving
Inhan Kim (POSTECH)*, Hyemin Lee (POSTECH), Joonyeong Lee (POSTECH), Eunseop Lee (POSTECH), Daijin Kim (Pohang University of Science and Technology)
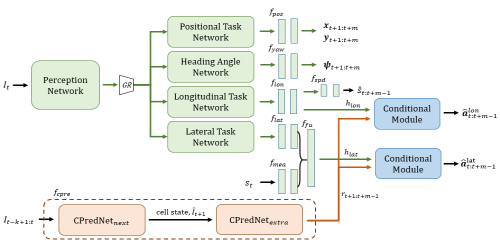
Reconstructing Human Body Mesh from Point Clouds by Adversarial GP Network
Boyao Zhou (Inria)*, Jean-Sebastien Franco (INRIA), Federica Bogo (Microsoft), Bugra Tekin (Microsoft), Edmond Boyer (Inria)
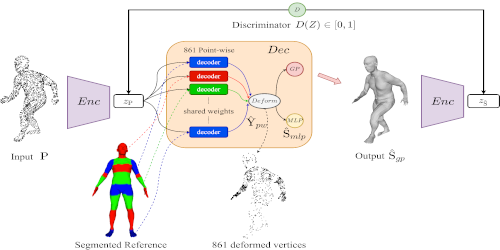