Reconstructing Human Body Mesh from Point Clouds by Adversarial GP Network
Boyao Zhou (Inria)*, Jean-Sebastien Franco (INRIA), Federica Bogo (Microsoft), Bugra Tekin (Microsoft), Edmond Boyer (Inria)
Keywords: 3D Computer Vision
Abstract:
We study the problem of reconstructing the template-aligned mesh for human body estimation from unstructured point cloud data. Recent studies of the shape matching problem using DNN methodologies have shown state-of-the-art results with generic point-wise architectures, but in so doing exploit much weaker human shape and surface priors in the inference than previous methods with explicit shape surface models. Since they are bound to improve the performance even more, we investigate the impact of adding back such constraints by proposing a new dedicated human template matching process with a point-based deep-autoencoder architecture, where surface consistency of surface points is enforced and parameterized with a specialized Gaussian Process layer, and whose global consistency and generalization abilities are enforced with adversarial training. The choice of these elements is grounded in a detailed review of failure cases in standard datasets SURREAL and FAUST. We validate and evaluate the impact of these components on this data with measured improvement over state of the art DNN methods, which also show through a leap in the visual quality of the results.
SlidesLive
Similar Papers
Adaptive Spotting: Deep Reinforcement Object Search in 3D Point Clouds
Onkar Krishna (NTT Corporation, Japan)*, Go Irie (NTT Corporation), Xiaomeng Wu (NTT Corporation), Takahito Kawanishi (NTT Corporation), Kunio Kashino (NTT Communication Science Laboratories)
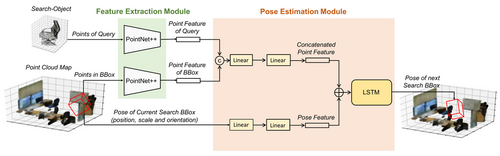
3D Guided Weakly Supervised Semantic Segmentation
Weixuan Sun (Australian National University, Data61 )*, Jing Zhang (Australian National University), Nick Barnes (ANU)
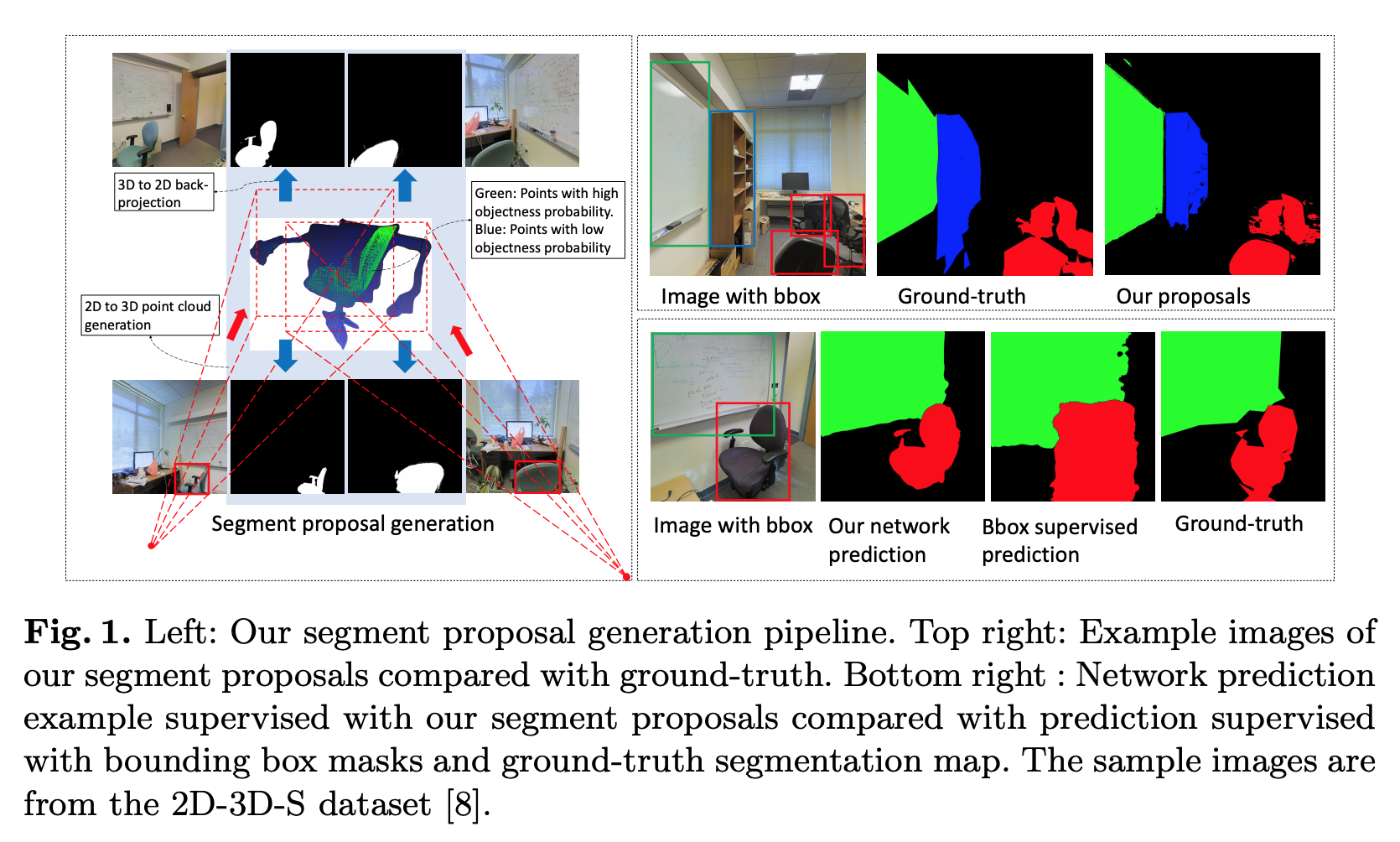
Weakly-supervised Reconstruction of 3D Objects with Large Shape Variation from Single In-the-Wild Images
Shichen Sun (Sichuan University), Zhengbang Zhu (Sichuan University), Xiaowei Dai (Sichuan University), Qijun Zhao (Sichuan University)*, Jing Li (Sichuan University)
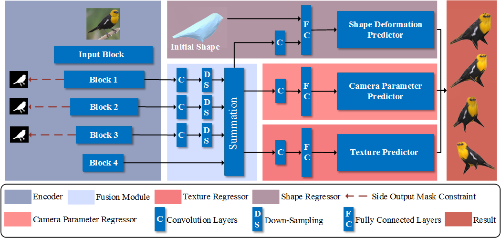