Query by Strings and Return Ranking Word Regions with Only One Look
Peng Zhao (Beijing Jiaotong University), Wenyuan Xue (Beijing Jiaotong University), Qingyong Li (Beijing Jiaotong University)*, Siqi Cai (Beijing Jiaotong University)
Keywords: Document image analysis
Abstract:
Word spotting helps people like archaeologists, historian and internet censors to retrieve regions of interest from document images according to the queries defined by them. However, words in handwritten historical document images are generally densely distributed and have many overlapping strokes, which make it challenging to apply word spotting in such scenarios. Recently, deep learning based methods have achieved significant performance improvement, which usually adopt two-stage object detectors to produce word segmentation results and then embed cropped word regions into a word embedding space. Different from these multi-stage methods, this paper presents an effective end-to-end trainable method for segmentation-free query-by-string word spotting. To the best of our knowledge, this is the first work that uses a single network to simultaneously predict word bounding box and word embedding in only one stage by adopting feature sharing and multi-task learning strategy. Experiments on several benchmarks demonstrate that the proposed method surpasses the previous state-of-the-art segmentation-free methods.
SlidesLive
Similar Papers
Image Captioning through Image Transformer
Sen He (University of Exeter)*, Wentong Liao (Leibniz University Hannover), Hamed R. Tavakoli (Nokia Technologies), Michael Yang (University of Twente), Bodo Rosenhahn (Leibniz University Hannover), Nicolas Pugeault (University of Glasgow)
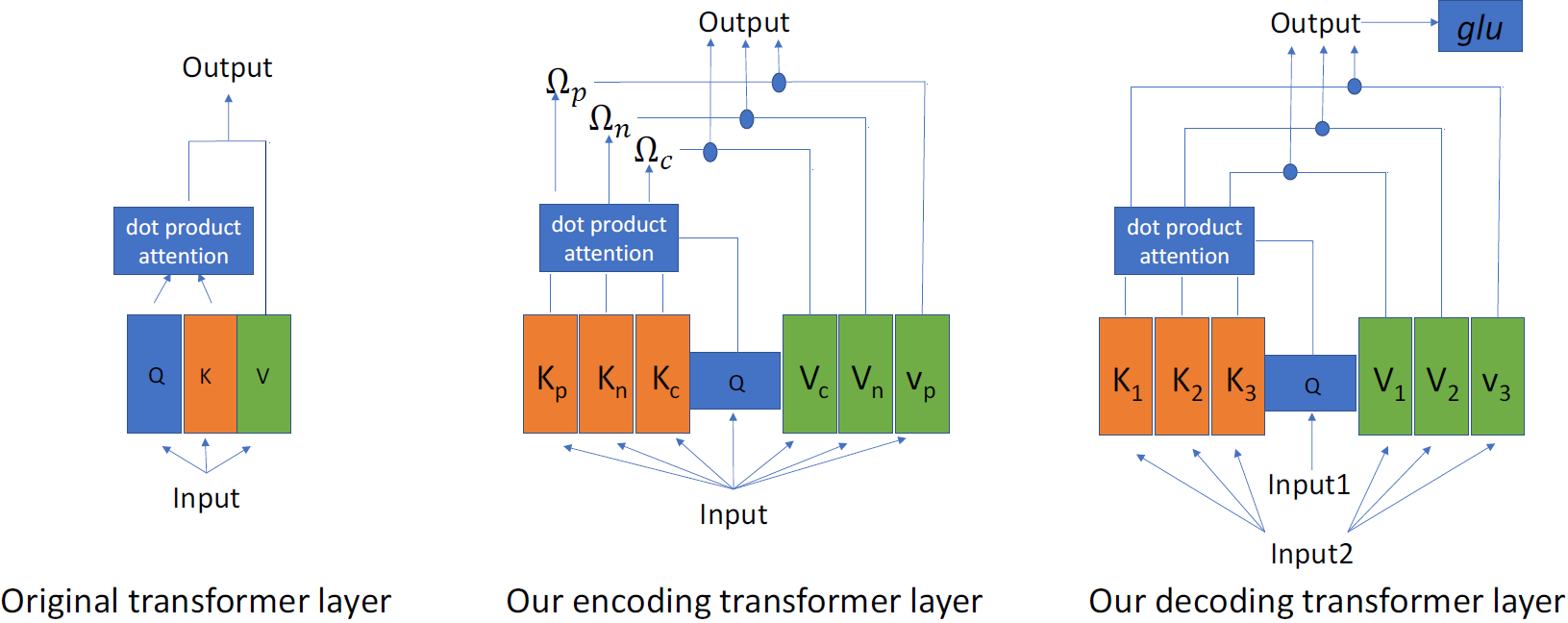
Show, Conceive and Tell: Image Captioning with Prospective Linguistic Information
Yiqing Huang (Tsinghua University), Jiansheng Chen (Tsinghua University)*
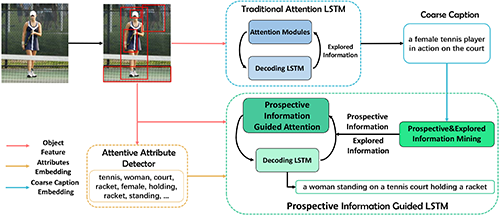
Rotation Axis Focused Attention Network (RAFA-Net) for Estimating Head Pose
Ardhendu Behera (Edge Hill University)*, Zachary Wharton (Edge Hill University), Pradeep Hewage (Edge Hill University), Swagat Kumar (Edge Hill University)
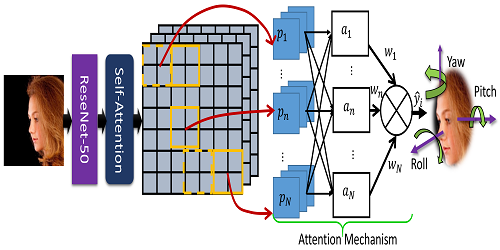