Visualizing Color-wise Saliency of Black-Box Image Classification Models
Yuhki Hatakeyama (SenseTime Japan)*, Hiroki Sakuma (SenseTime Japan), Yoshinori Konishi (SenseTime Japan), Kohei Suenaga (Kyoto University)
Keywords: Recognition: Feature Detection, Indexing, Matching, and Shape Representation
Abstract:
Image classification based on machine learning is being commonly used.However, a classification result given by an advanced method, including deep learning, is often hard to interpret.This problem of interpretability is one of the major obstacles in deploying a trained model in safety-critical systems.Several techniques have been proposed to address this problem;one of which is RISE, which explains a classification result by a heatmap, called a saliency map, that explains the significance of each pixel.We propose MC-RISE (Multi-Color RISE), which is an enhancement of RISE to take color information into account in an explanation.Our method not only shows the saliency of each pixel in a given image as the original RISE does, but the significance of color components of each pixel;a saliency map with color information is useful especially in the domain where the color information matters (e.g., traffic-sign recognition).We implemented MC-RISE and evaluate them using two datasets (GTSRB and ImageNet) to demonstrate the effectiveness of our methods in comparison with existing techniques for interpreting image classification results.
SlidesLive
Similar Papers
Project to Adapt: Domain Adaptation for Depth Completion from Noisy and Sparse Sensor Data
Adrian Lopez-Rodriguez (Imperial College London)*, Benjamin Busam (Technical University of Munich), Krystian Mikolajczyk (Imperial College London)
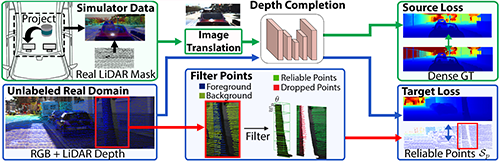
SGNet: Semantics Guided Deep Stereo Matching
Shuya Chen (Zhejiang University), Zhiyu Xiang (Zhejiang University)*, Chengyu Qiao (Zhejiang University), Yiman Chen (Zhejiang University), Tingming Bai (Zhejiang University)
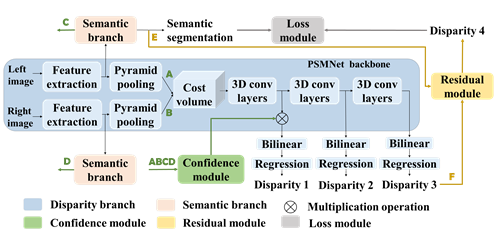
MLIFeat: Multi-level information fusion based deep local features
Yuyang Zhang (Institute of Automation, Chinese Academy of Sciences, University of Chinese Academy of Sciences), Jinge Wang (Megvii), Shibiao Xu (Institute of Automation, Chinese Academy of Sciences)*, Xiao Liu (Megvii Inc), Xiaopeng Zhang (Institute of Automation, Chinese Academy of Sciences)
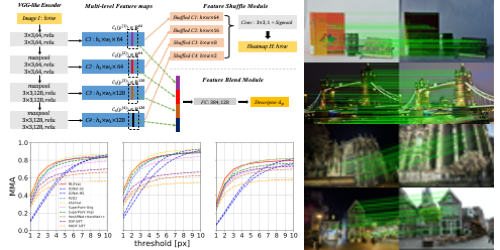