Project to Adapt: Domain Adaptation for Depth Completion from Noisy and Sparse Sensor Data
Adrian Lopez-Rodriguez (Imperial College London)*, Benjamin Busam (Technical University of Munich), Krystian Mikolajczyk (Imperial College London)
Keywords: 3D Computer Vision
Abstract:
Depth completion aims to predict a dense depth map from a sparse depth input. The acquisition of dense ground truth annotations for depth completion settings can be difficult and, at the same time, a significant domain gap between real LiDAR measurements and synthetic data has prevented from successful training of models in virtual settings. We propose a domain adaptation approach for sparse-to-dense depth completion that is trained from synthetic data, without annotations in the real domain or additional sensors. Our approach simulates the real sensor noise in an RGB~+~LiDAR set-up, and consists of three modules: simulating the real LiDAR input in the synthetic domain via projections, filtering the real noisy LiDAR for supervision and adapting the synthetic RGB image using a CycleGAN approach. We extensively evaluate these modules against the state-of-the-art in the KITTI depth completion benchmark, showing significant improvements.
SlidesLive
Similar Papers
MLIFeat: Multi-level information fusion based deep local features
Yuyang Zhang (Institute of Automation, Chinese Academy of Sciences, University of Chinese Academy of Sciences), Jinge Wang (Megvii), Shibiao Xu (Institute of Automation, Chinese Academy of Sciences)*, Xiao Liu (Megvii Inc), Xiaopeng Zhang (Institute of Automation, Chinese Academy of Sciences)
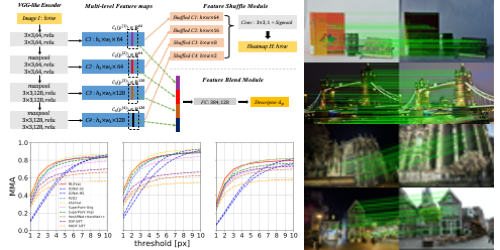
Visualizing Color-wise Saliency of Black-Box Image Classification Models
Yuhki Hatakeyama (SenseTime Japan)*, Hiroki Sakuma (SenseTime Japan), Yoshinori Konishi (SenseTime Japan), Kohei Suenaga (Kyoto University)
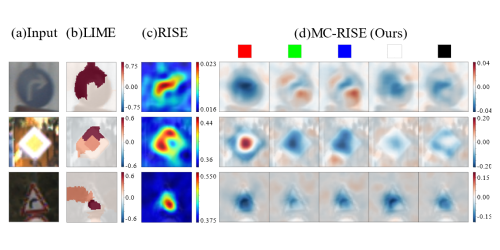
Multiple Exemplars-based Hallucination for Face Super-resolution and Editing
Kaili Wang (KU Leuven, UAntwerpen)*, Jose Oramas (UAntwerp, imec-IDLab), Tinne Tuytelaars (KU Leuven)
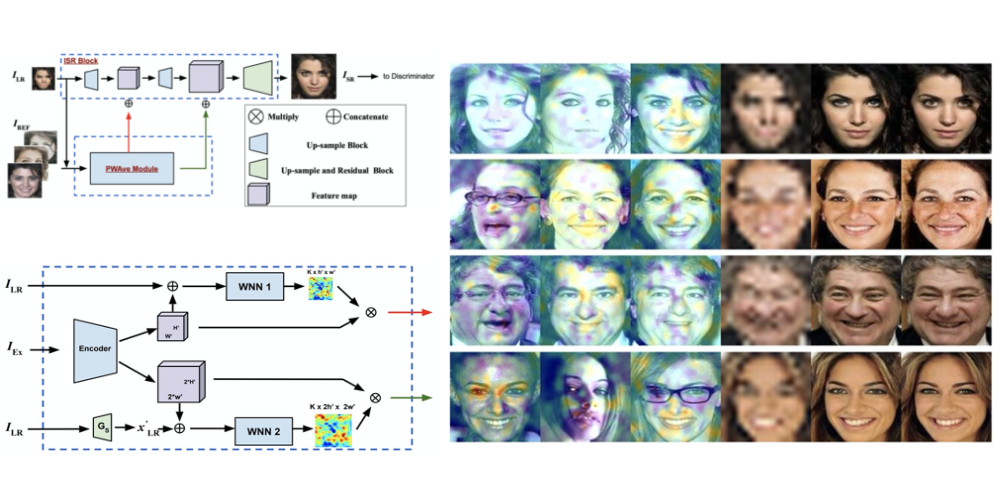