MLIFeat: Multi-level information fusion based deep local features
Yuyang Zhang (Institute of Automation, Chinese Academy of Sciences, University of Chinese Academy of Sciences), Jinge Wang (Megvii), Shibiao Xu (Institute of Automation, Chinese Academy of Sciences)*, Xiao Liu (Megvii Inc), Xiaopeng Zhang (Institute of Automation, Chinese Academy of Sciences)
Keywords: Recognition: Feature Detection, Indexing, Matching, and Shape Representation
Abstract:
Accurate image keypoints detection and description are of central importance in a wide range of applications. Although there are various studies proposed to address these challenging tasks, they are far from optimal. In this paper, we devise a model named MLIFeat with two novel light-weight modules for multi-level information fusion based deep local features learning, to cope with both the image keypoints detection and description. On the one hand, the image keypoints are robustly detected by our Feature Shuffle Module (FSM), which can efficiently utilize the multi-level convolutional feature maps with marginal computing cost. On the other hand, the corresponding feature descriptors are generated by our well-designed Feature Blend Module (FBM), which can collect and extract the most useful information from the multi-level convolutional feature vectors. To study in-depth about our MLIFeat and other state-of-the-art methods, we have conducted thorough experiments, including image matching on HPatches and FM-Bench, and visual localization on Aachen-Day-Night, which verifies the robustness and effectiveness of our proposed model. Code at:https://github.com/yyangzh/MLIFeat
SlidesLive
Similar Papers
Project to Adapt: Domain Adaptation for Depth Completion from Noisy and Sparse Sensor Data
Adrian Lopez-Rodriguez (Imperial College London)*, Benjamin Busam (Technical University of Munich), Krystian Mikolajczyk (Imperial College London)
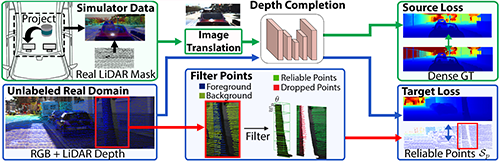
Multiple Exemplars-based Hallucination for Face Super-resolution and Editing
Kaili Wang (KU Leuven, UAntwerpen)*, Jose Oramas (UAntwerp, imec-IDLab), Tinne Tuytelaars (KU Leuven)
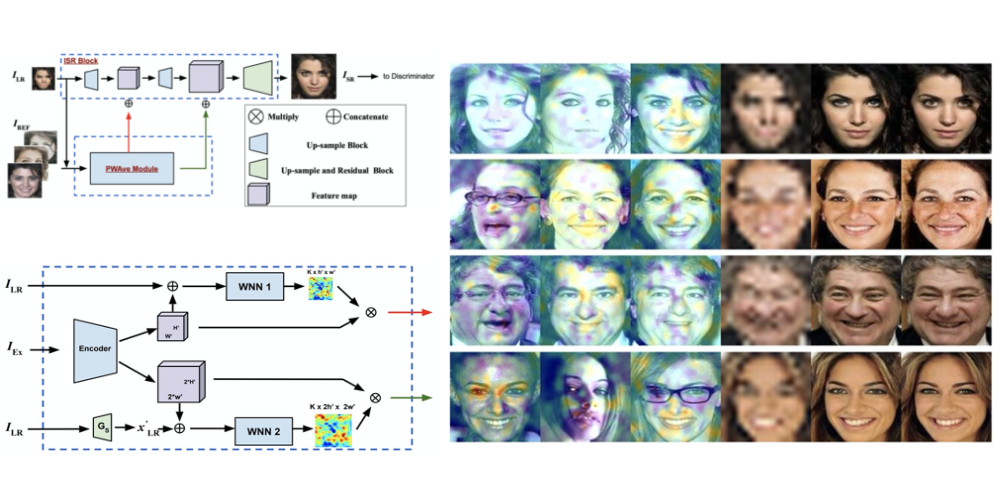
Visualizing Color-wise Saliency of Black-Box Image Classification Models
Yuhki Hatakeyama (SenseTime Japan)*, Hiroki Sakuma (SenseTime Japan), Yoshinori Konishi (SenseTime Japan), Kohei Suenaga (Kyoto University)
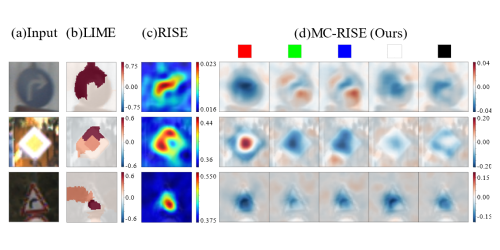