A cost-effective method for improving and re-purposing large, pre-trained GANs by fine-tuning their class-embeddings
Qi Li (Auburn University), Long Mai (Adobe Research), Michael A. Alcorn (Auburn University), Anh Nguyen (Auburn University)*
Keywords: Generative models for computer vision
Abstract:
Large, pre-trained generative models have been increasingly popular and useful to both the research and wider communities. Specifically, BigGANs a class-conditional Generative Adversarial Networks trained on ImageNet---achieved excellent, state-of-the-art capability in generating realistic photos. However, fine-tuning or training BigGANs from scratch is practically impossible for most researchers and engineers because (1) GAN training is often unstable and suffering from mode-collapse; and (2) the training requires a significant amount of computation, 256 Google TPUs for 2 days or 8xV100 GPUs for 15 days. Importantly, many pre-trained generative models both in NLP and image domains were found to contain biases that are harmful to society. Thus, we need computationally-feasible methods for modifying and re-purposing these huge, pre-trained models for downstream tasks. In this paper, we propose a cost-effective optimization method for improving and re-purposing BigGANs by fine-tuning only the class-embedding layer. We show the effectiveness of our model-editing approach in three tasks: (1) significantly improving the realism and diversity of samples of complete mode-collapse classes; (2) re-purposing ImageNet BigGANs for generating images for Places365; and (3) de-biasing or improving the sample diversity for selected ImageNet classes.
SlidesLive
Similar Papers
COG: COnsistent data auGmentation for object perception
Zewen He (Casia)*, Rui Wu (Horizon Robotics), Dingqian Zhang (Horizon Robotics)
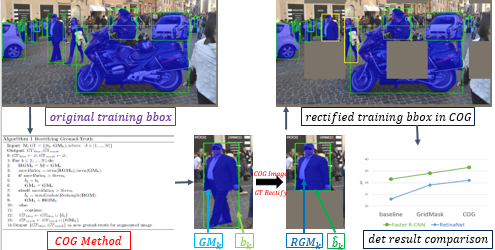
Over-exposure Correction via Exposure and Scene Information Disentanglement
Yuhui Cao (SECE, Shenzhen Graduate School, Peking University)*, Yurui Ren (Shenzhen Graduate School, Peking University), Thomas H. Li (Advanced Institute of Information Technology, Peking University), Ge Li (SECE, Shenzhen Graduate School, Peking University)
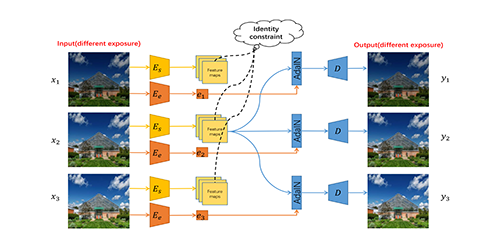
A Benchmark and Baseline for Language-Driven Image Editing
Jing Shi (University of Rochester)*, Ning Xu (Adobe Research), Trung Bui (Adobe Research), Franck Dernoncourt (Adobe Research), Zheng Wen (DeepMind), Chenliang Xu (University of Rochester)
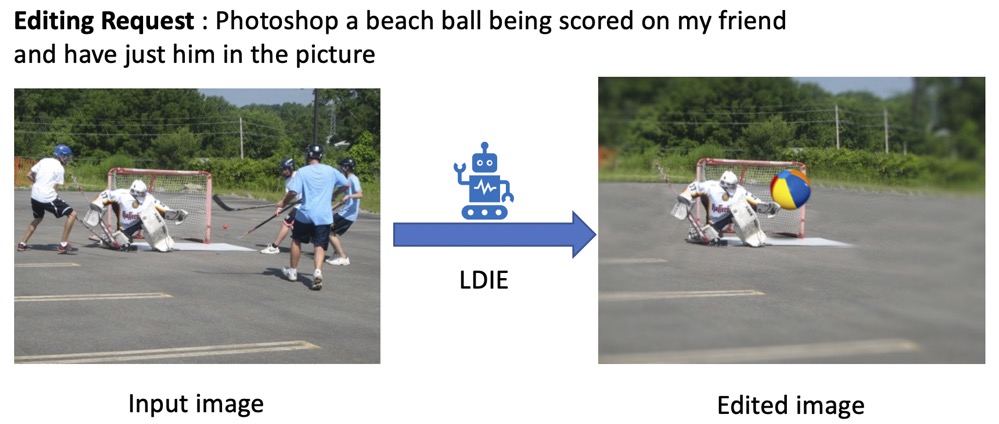