COG: COnsistent data auGmentation for object perception
Zewen He (Casia)*, Rui Wu (Horizon Robotics), Dingqian Zhang (Horizon Robotics)
Keywords: Recognition: Feature Detection, Indexing, Matching, and Shape Representation
Abstract:
Recently, data augmentation techniques for training conv-nets emerge one after another, especially focusing on image classification. They're always applied to object detection without further careful design. In this paper we propose COG, a general domain migration scheme for augmentation. Specifically, based on a particular augmentation, we first analyze its inherent inconsistency, and then adopt an adaptive strategy to rectify ground-truths of the augmented input images. Next, deep detection networks are trained on the rectified data to achieve better performance. Our extensive experiments show that our method COG's performance is superior to its competitor on detection and instance segmentation tasks. In addition, the results manifest the robustness of COG when faced with hyper-parameter variations, etc.
SlidesLive
Similar Papers
A cost-effective method for improving and re-purposing large, pre-trained GANs by fine-tuning their class-embeddings
Qi Li (Auburn University), Long Mai (Adobe Research), Michael A. Alcorn (Auburn University), Anh Nguyen (Auburn University)*
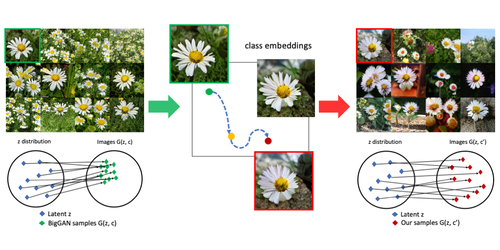
Attended-Auxiliary Supervision Representation for Face Anti-spoofing
Son Minh Nguyen (Teikyo University)*, Linh Duy Tran (Teikyo University), Masayuki Arai (Teikyo Univ.)
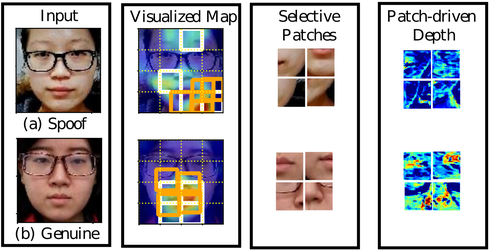
Towards Robust Fine-grained Recognition by Maximal Separation of Discriminative Features
Krishna Kanth Nakka (EPFL)*, Mathieu Salzmann (EPFL)
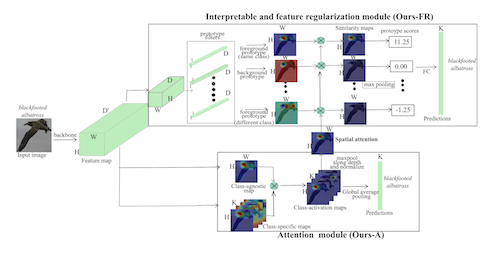