A Benchmark and Baseline for Language-Driven Image Editing
Jing Shi (University of Rochester)*, Ning Xu (Adobe Research), Trung Bui (Adobe Research), Franck Dernoncourt (Adobe Research), Zheng Wen (DeepMind), Chenliang Xu (University of Rochester)
Keywords: Applications of Computer Vision, Vision for X; Datasets and Performance Analysis
Abstract:
Language-driven image editing can significantly save the laborious image editing work and be friendly to the photography novice. However, most similar work can only deal with a specific image domain or can only do global retouching. To solve this new task, we first present a new language-driven image editing dataset that supports both local and global editing with editing operation and mask annotations. Besides, we also propose a baseline method that fully utilizes the annotation to solve this problem. Our new method treats each editing operation as a sub-module and can automatically predict operation parameters. Not only performing well on challenging user data, but such an approach is also highly interpretable. We believe our work, including both the benchmark and the baseline, will advance the image editing area towards a more general and free-form level.
SlidesLive
Similar Papers
FAN: Feature Adaptation Network for Surveillance Face Recognition and Normalization
Xi Yin (Microsoft Cloud & AI)*, Ying Tai (Tencent YouTu), Yuge Huang (Tencent YouTu), Xiaoming Liu (Michigan State University)
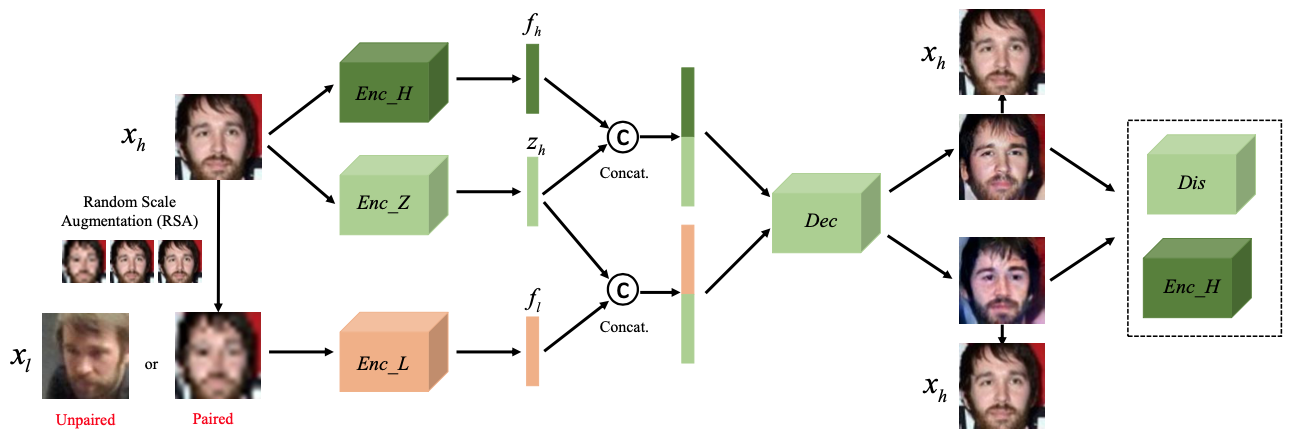
Second-order Camera-aware Color Transformation for Cross-domain Person Re-identification
Wangmeng Xiang (The Hong Kong Polytechnic University), Hongwei Yong (The Hong Kong Polytechnic University), Jianqiang Huang (Damo Academy, Alibaba Group), Xian-Sheng Hua (Alibaba Group), Lei Zhang ("Hong Kong Polytechnic University, Hong Kong, China")*
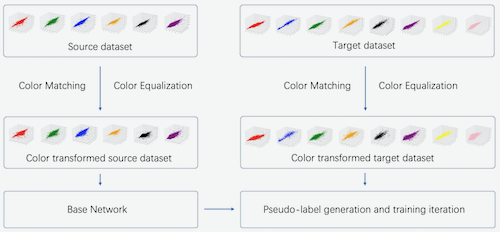
SGNet: Semantics Guided Deep Stereo Matching
Shuya Chen (Zhejiang University), Zhiyu Xiang (Zhejiang University)*, Chengyu Qiao (Zhejiang University), Yiman Chen (Zhejiang University), Tingming Bai (Zhejiang University)
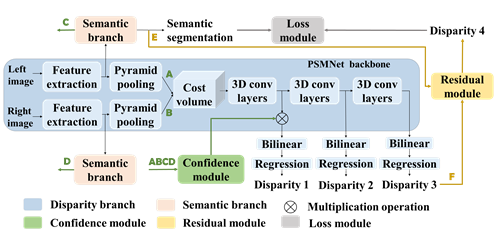