Over-exposure Correction via Exposure and Scene Information Disentanglement
Yuhui Cao (SECE, Shenzhen Graduate School, Peking University)*, Yurui Ren (Shenzhen Graduate School, Peking University), Thomas H. Li (Advanced Institute of Information Technology, Peking University), Ge Li (SECE, Shenzhen Graduate School, Peking University)
Keywords: Generative models for computer vision ; Illumination and Reflectance Modeling
Abstract:
Over-exposure correction is an important problem of great consequence to social media industries. In this paper, we propose a novel model to tackle this task. Considering that reasonable enhanced results can still vary in terms of exposure, we do not strictly enforce the model to generate identical results with ground-truth images. On the contrary, we train the network to recover the lost scene information according to the existing information of the over-exposure images and generate naturalness-preserved images. Experiments compared with several state-of-the-art methods show the superior performance of the proposed network. Besides, we also verify our hypothesis with ablation studies. Our source code is available at \url{https://github.com/0x437968/overexposure-correction-dise}.
SlidesLive
Similar Papers
A cost-effective method for improving and re-purposing large, pre-trained GANs by fine-tuning their class-embeddings
Qi Li (Auburn University), Long Mai (Adobe Research), Michael A. Alcorn (Auburn University), Anh Nguyen (Auburn University)*
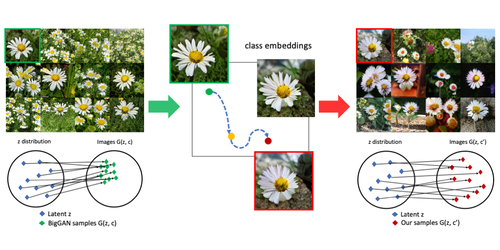
COG: COnsistent data auGmentation for object perception
Zewen He (Casia)*, Rui Wu (Horizon Robotics), Dingqian Zhang (Horizon Robotics)
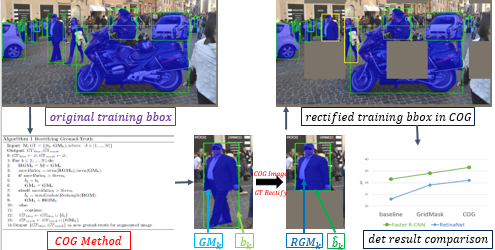
Attended-Auxiliary Supervision Representation for Face Anti-spoofing
Son Minh Nguyen (Teikyo University)*, Linh Duy Tran (Teikyo University), Masayuki Arai (Teikyo Univ.)
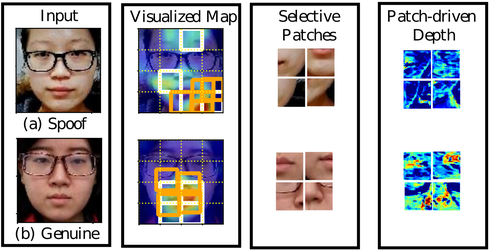