RGB-D Co-attention Network for Semantic Segmentation
Hao Zhou (Harbin Engineering University)*, Lu Qi (The Chinese University of Hong Kong), Zhaoliang Wan (Harbin Engineering University), Hai Huang (Harbin Engineering University), Xu Yang (Chinese Academy of Sciences)
Keywords: RGBD and Depth Image Processing
Abstract:
Incorporating the depth (D) information for RGB images has proven the effectiveness and robustness in semantic segmentation. However, the fusion between them is still a challenge due to their meaning discrepancy, in which RGB represents the color but D depth information. In this paper, we propose a co-attention Network (CANet) to capture the fine-grained interplay between RGB�_and D�_ features. The key part in our CANet is co-attention fusion part. It includes three modules. At first, the position and channel co-attention fusion modules adaptively fuse color and depth features in spatial and channel dimension. Finally, a final fusion module integrates the outputs of the two co-attention fusion modules for forming a more representative feature. Our extensive experiments validate the effectiveness of CANet in fusing RGB and D features, achieving the state-of-the-art performance on two challenging RGB-D semantic segmentation datasets, i.e., NYUDv2, SUN-RGBD.
SlidesLive
Similar Papers
Jointly Discriminating and Frequent Visual Representation Mining
Qiannan Wang (Xidian university), Ying Zhou (Xidian University), ZhaoYan Zhu (Xidian university), Xuefeng Liang (Xidian University)*, Yu Gu (School of Artificial Intelligence, Xi'dian University)
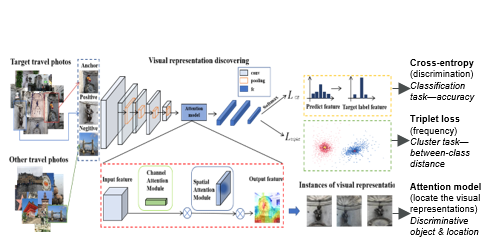
Explaining an image classifier's decisions using generative models
Chirag Agarwal (UIC), Anh Nguyen (Auburn University)*
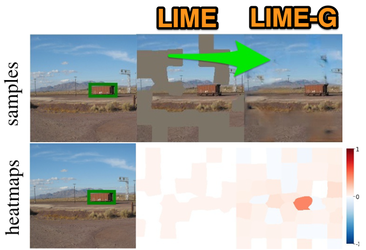
RF-GAN: A Light and Reconfigurable Network for Unpaired Image-to-Image Translation
Ali Koksal (Nanyang Technological University), Shijian Lu (Nanyang Technological University)*
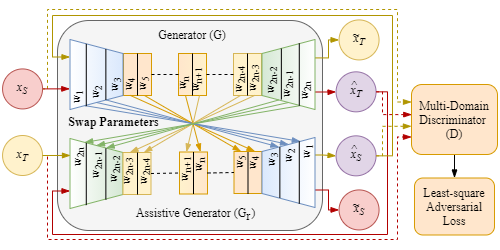