Explaining an image classifier's decisions using generative models
Chirag Agarwal (UIC), Anh Nguyen (Auburn University)*
Keywords: Applications of Computer Vision, Vision for X
Abstract:
Perturbation-based explanation methods often measure the contribution of an input feature to an image classifier's outputs by heuristically removing it via e.g. blurring, adding noise, or graying out, which often produce unrealistic, out-of-samples. Instead, we propose to integrate a generative inpainter into three representative attribution methods to remove an input feature. Our proposed change improved all three methods in (1) generating more plausible counterfactual samples under the true data distribution; (2) being more accurate according to three metrics: object localization, deletion, and saliency metrics; and (3) being more robust to hyperparameter changes. Our findings were consistent across both ImageNet and Places365 datasets and two different pairs of classifiers and inpainters.
SlidesLive
Similar Papers
RGB-D Co-attention Network for Semantic Segmentation
Hao Zhou (Harbin Engineering University)*, Lu Qi (The Chinese University of Hong Kong), Zhaoliang Wan (Harbin Engineering University), Hai Huang (Harbin Engineering University), Xu Yang (Chinese Academy of Sciences)
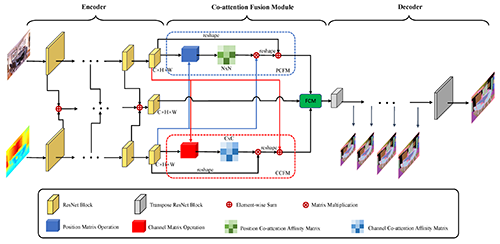
MIX'EM: Unsupervised Image Classification using a Mixture of Embeddings
Ali Varamesh (KU Leuven)*, Tinne Tuytelaars (KU Leuven)
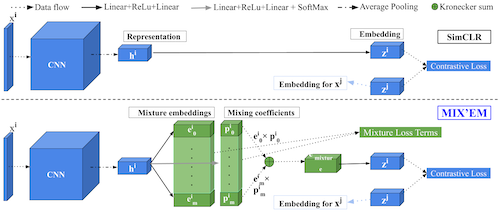
Robust High Dynamic Range (HDR) Imaging with Complex Motion and Parallax
Zhiyuan Pu (NanJing University), Peiyao Guo (Nanjing University), M. Salman Asif (University of California, Riverside), Zhan Ma (Nanjing University)*
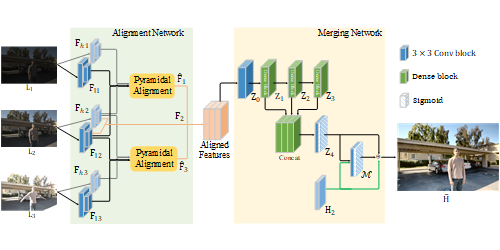