Video-Based Crowd Counting Using a Multi-Scale Optical Flow Pyramid Network
Mohammad Asiful Hossain (HUAWEI Technologies Co, LTD.)*, Kevin Cannons (Huawei Technologies Canada Co., Ltd ), Daesik Jang (Personal Research), Fabio Cuzzolin (Oxford Brookes University), Zhan Xu (Huawei Canada)
Keywords: Motion and Tracking; Video Analysis and Event Recognition
Abstract:
This paper presents a novel approach to the task of video-based crowd counting, which can be formalized as the regression problem of learning a mapping from an input image to an output crowd density map. Convolutional neural networks (CNNs) have demonstrated striking accuracy gains in a range of computer vision tasks, including crowd counting. However, the dominant focus within the crowd counting literature has been on the single-frame case or applying CNNs to videos in a frame-by-frame fashion without leveraging motion information. This paper proposes a novel architecture that exploits the spatiotemporal information captured in a video stream by combining an optical flow pyramid with an appearance-based CNN. Extensive empirical evaluation on five public datasets comparing against numerous state-of-the-art approaches demonstrates the efficacy of the proposed architecture, with our methods reporting best results on all datasets. Finally, a set of transfer learning experiments shows that, once the proposed model is trained on one dataset, it can be transferred to another using a limited number of training examples and still exhibit high accuracy.
SlidesLive
Similar Papers
Channel Recurrent Attention Networks for Video Pedestrian Retrieval
Pengfei Fang (The Australian National University)*, Pan Ji (OPPO US Research Center), Jieming Zhou (The Australian National University), Lars Petersson (Data61/CSIRO), Mehrtash Harandi (Monash University)
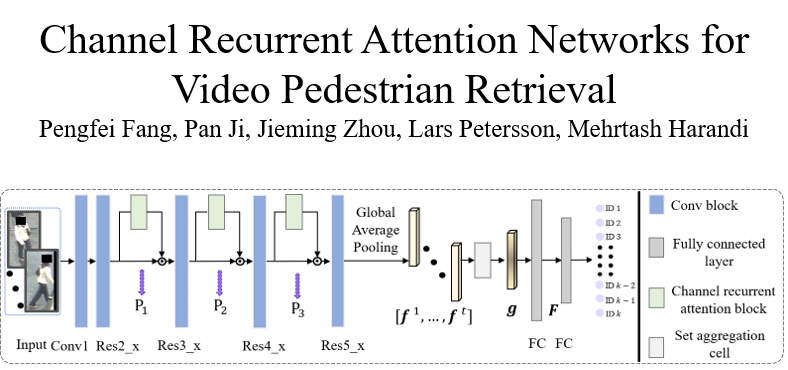
Transforming Multi-Concept Attention into Video Summarization
Yen-Ting Liu (National Taiwan University)*, Yu-Jhe Li (Carnegie Mellon University), Yu-Chiang Frank Wang (National Taiwan University)
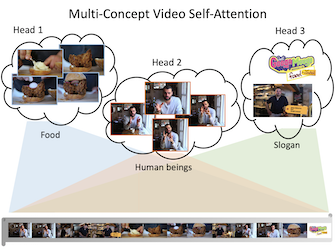
Feedback Recurrent Autoencoder for Video Compression
Adam Golinski (University of Oxford)*, Reza Pourreza (Qualcomm), Yang Yang (Qualcomm Inc.), Guillaume Sautiere (Qualcomm AI Research), Taco S. Cohen (Qualcomm)
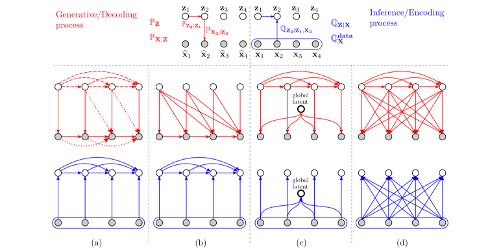