A Sparse Gaussian Approach to Region-Based 6DoF Object Tracking
Manuel Stoiber (German Aerospace Center (DLR))*, Martin Pfanne (German Aerospace Center), Klaus H. Strobl (DLR), Rudolph Triebel (German Aerospace Center (DLR)), Alin Albu-Schaeffer (Robotics and Mechatronics Center (RMC), German Aerospace Center (DLR))
Keywords: Motion and Tracking
Abstract:
We propose a novel, highly efficient sparse approach to region-based 6DoF object tracking that requires only a monocular RGB camera and the 3D object model. The key contribution of our work is a probabilistic model that considers image information sparsely along correspondence lines. For the implementation, we provide a highly efficient discrete scale-space formulation. In addition, we derive a novel mathematical proof that shows that our proposed likelihood function follows a Gaussian distribution. Based on this information, we develop robust approximations for the derivatives of the log-likelihood that are used in a regularized Newton optimization. In multiple experiments, we show that our approach outperforms state-of-the-art region-based methods in terms of tracking success while being about one order of magnitude faster. The source code of our tracker is publicly available.
SlidesLive
Similar Papers
Homography-based Egomotion Estimation Using Gravity and SIFT Features
Yaqing Ding (Nanjing University of Science and Technology)*, Daniel Barath (MTA SZTAKI, CMP Prague), Zuzana Kukelova (Czech Technical University in Prague)
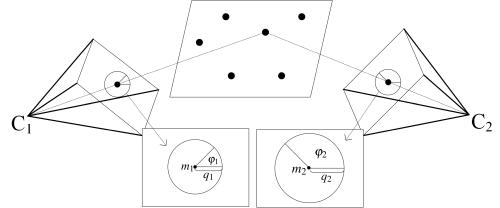
Recursive Bayesian Filtering for Multiple Human Pose Tracking from Multiple Cameras
Oh-Hun Kwon (University of Bonn), Julian Tanke (University of Bonn)*, Jürgen Gall (University of Bonn)
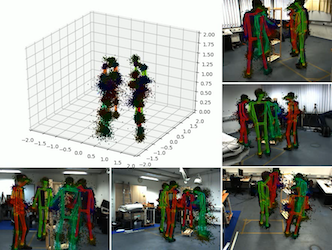
IAFA: Instance-Aware Feature Aggregation for 3D Object Detection from a Single Image
Dingfu Zhou (Baidu)*, Xibin Song (Baidu), Yuchao Dai (Northwestern Polytechnical University), Junbo Yin (Beijing Institute of Technology), Feixiang Lu (Baidu), Miao Liao (Baidu), Jin Fang (Baidu ), Liangjun Zhang (Baidu)
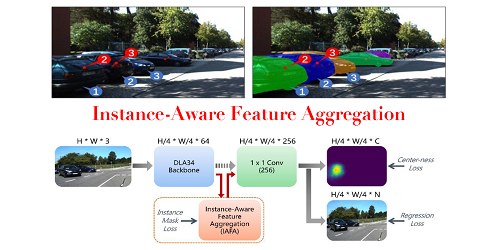