IAFA: Instance-Aware Feature Aggregation for 3D Object Detection from a Single Image
Dingfu Zhou (Baidu)*, Xibin Song (Baidu), Yuchao Dai (Northwestern Polytechnical University), Junbo Yin (Beijing Institute of Technology), Feixiang Lu (Baidu), Miao Liao (Baidu), Jin Fang (Baidu ), Liangjun Zhang (Baidu)
Keywords: 3D Computer Vision; Applications of Computer Vision, Vision for X
Abstract:
3D object detection from a single image is an important task in Autonomous Driving (AD), where various approaches have been proposed. However, the task is intrinsically ambiguous and challenging as single image depth estimation is already an ill-posed problem. In this paper, we propose an instance-aware approach to aggregate useful information for improving the accuracy of 3D object detection with the following contributions. First, an instance-aware feature aggregation (IAFA) module is proposed to collect local and global features for 3D bounding boxes regression. Second, we empirically find that the spatial attention module can be well learned by taking coarse-level instance annotations as a supervision signal. The proposed module has significantly boosted the performance of the baseline method on both 3D detection and 2D bird-eye's view of vehicle detection among all three categories. Third, our proposed method outperforms all single image-based approaches (even these methods trained with depth as auxiliary inputs) and achieves state-of-the-art 3D detection performance on the KITTI benchmark.
SlidesLive
Similar Papers
SDP-Net: Scene Flow Based Real-time Object Detection and Prediction from Sequential 3D Point Clouds
Yi Zhang (Zhejiang University), Yuwen Ye (Zhejiang University), Zhiyu Xiang (Zhejiang University)*, Jiaqi Gu (Zhejiang University)
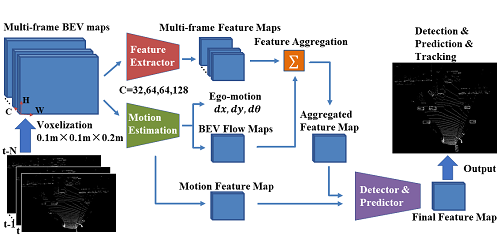
Adaptive Spatio-Temporal Regularized Correlation Filters for UAV-based Tracking
Libin Xu (Shandong University of Technology), Qilei Li (Sichuan University), Jun Jiang ( Southwest Petroleum University;Sichuan University of Science & Engineering), Guofeng Zou (Shandong University of Technology), Zheng Liu (University of British Columbia), Mingliang Gao (Shandong University of Technology)*
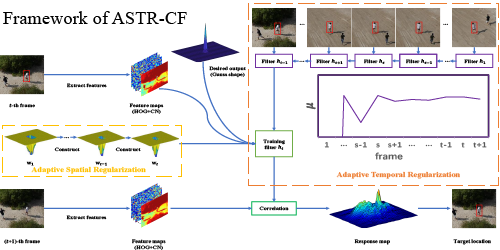
Bi-Directional Attention for Joint Instance and Semantic Segmentation in Point Clouds
guangnan wu (Shandong university)*, Zhiyi Pan (Shandong University), Peng Jiang (Shandong University), Changhe Tu (Shandong University)
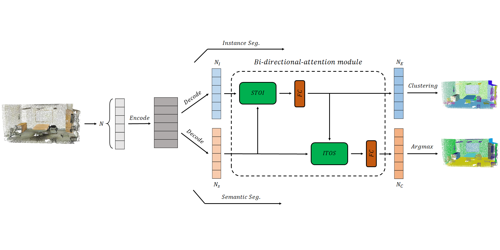