Bi-Directional Attention for Joint Instance and Semantic Segmentation in Point Clouds
guangnan wu (Shandong university)*, Zhiyi Pan (Shandong University), Peng Jiang (Shandong University), Changhe Tu (Shandong University)
Keywords: 3D Computer Vision
Abstract:
Instance segmentation in point clouds is one of the most fine-grained ways to understand the 3D scene. Due to its close relationship to semantic segmentation, many works approach these two tasks simultaneously and leverage the benefits of multi-task learning. However, most of them only considered simple strategies such as element-wise feature fusion, which may not lead to mutual promotion. In this work, we build a Bi-Directional Attention module on backbone neural networks for 3D point cloud perception, which uses similarity matrix measured from features for one task to help aggregate non-local information for the other task, avoiding the potential feature exclusion and task conflict. From comprehensive experiments, ablation studies and efficiency studies on the S3DIS dataset and the PartNet dataset, the superiority of our method is verified. Moreover, the mechanism of how bi-directional attention module helps joint instance and semantic segmentation is also analyzed.
SlidesLive
Similar Papers
SDP-Net: Scene Flow Based Real-time Object Detection and Prediction from Sequential 3D Point Clouds
Yi Zhang (Zhejiang University), Yuwen Ye (Zhejiang University), Zhiyu Xiang (Zhejiang University)*, Jiaqi Gu (Zhejiang University)
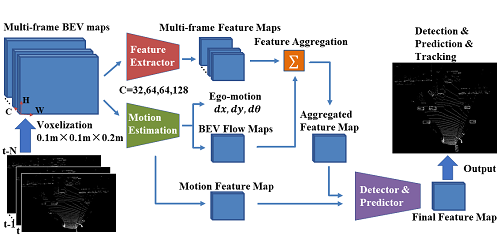
IAFA: Instance-Aware Feature Aggregation for 3D Object Detection from a Single Image
Dingfu Zhou (Baidu)*, Xibin Song (Baidu), Yuchao Dai (Northwestern Polytechnical University), Junbo Yin (Beijing Institute of Technology), Feixiang Lu (Baidu), Miao Liao (Baidu), Jin Fang (Baidu ), Liangjun Zhang (Baidu)
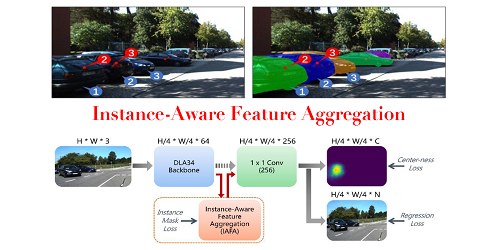
Recursive Bayesian Filtering for Multiple Human Pose Tracking from Multiple Cameras
Oh-Hun Kwon (University of Bonn), Julian Tanke (University of Bonn)*, Jürgen Gall (University of Bonn)
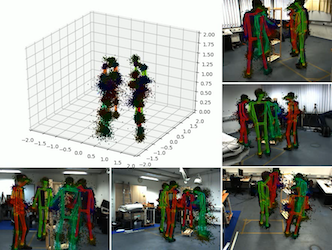