DeepSEE: Deep Disentangled Semantic Explorative Extreme Super-Resolution
Marcel C. Bühler (ETH Zürich)*, Andrés Romero (ETH Zürich), Radu Timofte (ETH Zurich)
Keywords: Generative models for computer vision
Abstract:
Super-resolution (SR) is by definition ill-posed. There are infinitely many plausible high-resolution variants for a given low-resolution natural image. Most of the current literature aims at a single deterministic solution of either high reconstruction fidelity or photo-realistic perceptual quality. In this work, we propose an explorative facial super-resolution framework, DeepSEE, for Deep disentangled Semantic Explorative Extreme super-resolution. To the best of our knowledge, DeepSEE is the first method to leverage semantic maps for explorative super-resolution. In particular, it provides control of the semantic regions, their disentangled appearance and it allows a broad range of image manipulations. We validate DeepSEE on faces, for up to 32x magnification and exploration of the space of super-resolution. Our code and models are available at: https://mcbuehler.github.io/DeepSEE/
SlidesLive
Similar Papers
Multiple Exemplars-based Hallucination for Face Super-resolution and Editing
Kaili Wang (KU Leuven, UAntwerpen)*, Jose Oramas (UAntwerp, imec-IDLab), Tinne Tuytelaars (KU Leuven)
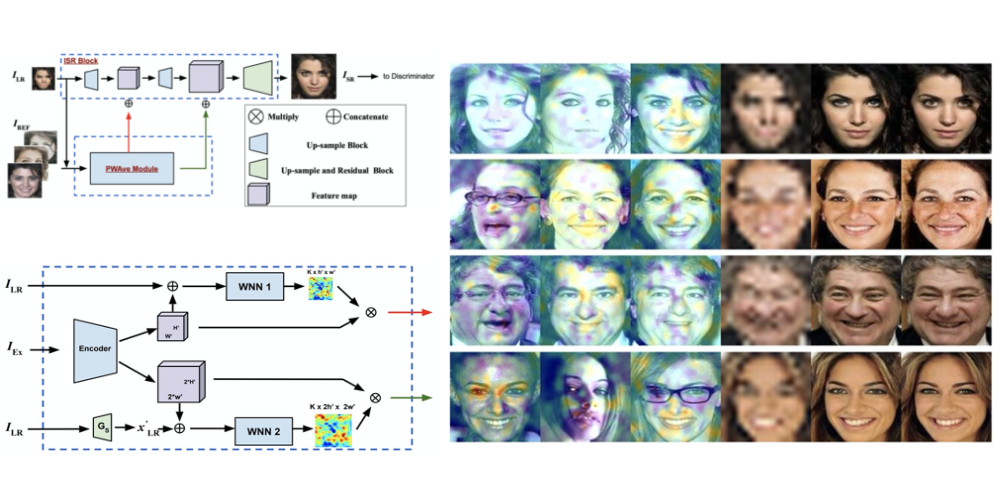
Point Proposal based Instance Segmentation with Rectangular Masks for Robot Picking Task
Satoshi Ito (Toshiba Corporation)*, Susumu Kubota (Toshiba Corporation)
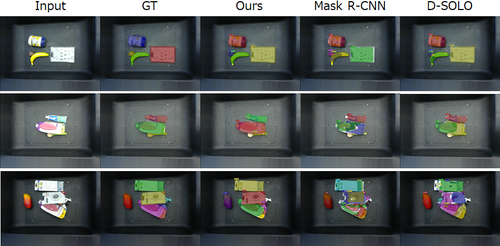
Over-exposure Correction via Exposure and Scene Information Disentanglement
Yuhui Cao (SECE, Shenzhen Graduate School, Peking University)*, Yurui Ren (Shenzhen Graduate School, Peking University), Thomas H. Li (Advanced Institute of Information Technology, Peking University), Ge Li (SECE, Shenzhen Graduate School, Peking University)
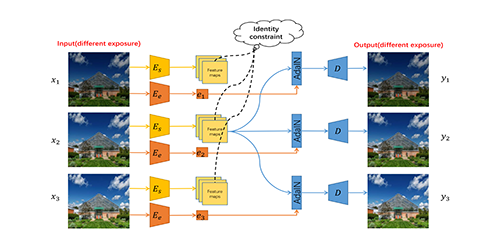