Efficient Large-Scale Semantic Visual Localization in 2D Maps
Tomas Vojir (CMP CTU)*, Ignas Budvytis (Department of Engineering, University of Cambridge), Roberto Cipolla (University of Cambridge)
Keywords: Recognition: Feature Detection, Indexing, Matching, and Shape Representation
Abstract:
With the emergence of autonomous navigation systems, image-based localization is one of the essential tasks to be tackled. However, most of the current algorithms struggle to scale to city-size environments mainly because of the need to collect large (semi-)annotated datasets for CNN training and create databases for test environment of images, keypoint level features or image embeddings. This data acquisition is not only expensive and time-consuming but also may cause privacy concerns. In this work, we propose a novel framework for semantic visual localization in city-scale environments which alleviates the aforementioned problem by using freely available 2D maps such as OpenStreetMap. Our method does not require any images or image-map pairs for training or test environment database collection. Instead, a robust embedding is learned from a depth and building instance label information of a particular location in the 2D map. At test time, this embedding is extracted from a panoramic building instance label and depth images. It is then used to retrieve the closest match in the database.We evaluate our localization framework on two large-scale datasets consisting of Cambridge and San Francisco cities with a total length of drivable roads spanning over 500 km and including approximately 110k unique locations. To the best of our knowledge, this is the first large-scale semantic localization method which works on par with approaches that require the availability of images at train time or for test environment database creation.
SlidesLive
Similar Papers
A Two-Stage Minimum Cost Multicut Approach to Self-Supervised Multiple Person Tracking
Kalun Ho (Fraunhofer ITWM)*, Amirhossein Kardoost (University of Mannheim), Franz-Josef Pfreundt (Fraunhofer ITWM), Janis Keuper (hs-offenburg), Margret Keuper (University of Mannheim)
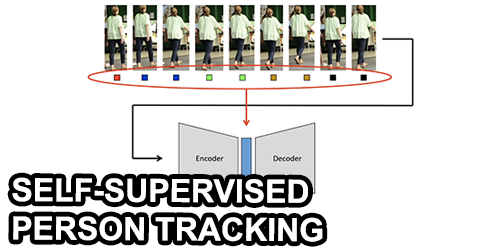
Semantic Synthesis of Pedestrian Locomotion
Maria Priisalu (Lund University)*, Ciprian Paduraru (IMAR), Aleksis Pirinen (Lund University), Cristian Sminchisescu (Lund University)
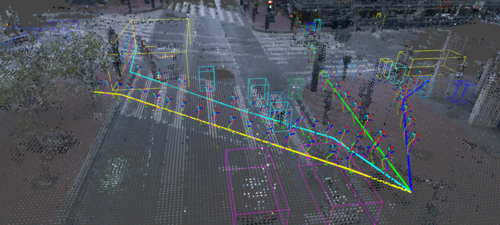
3D Object Detection from Consecutive Monocular Images
Chia-Chun Cheng (National Tsing Hua University)*, Shang-Hong Lai (Microsoft)
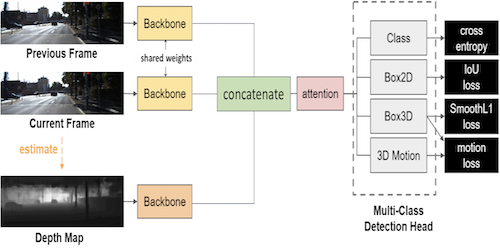