Semantic Synthesis of Pedestrian Locomotion
Maria Priisalu (Lund University)*, Ciprian Paduraru (IMAR), Aleksis Pirinen (Lund University), Cristian Sminchisescu (Lund University)
Keywords: Motion and Tracking
Abstract:
We present a model for generating 3d articulated pedestrian locomotion in urban scenarios, with synthesis capabilities informed by the 3d scene semantics and geometry. We reformulate pedestrian trajectory forecasting as a structured reinforcement learning (RL) problem. This allows us to naturally combine prior knowledge on collision avoidance, 3d human motion capture and the motion of pedestrians as observed e.g. in Cityscapes, Waymo or simulation environments like Carla. Our proposed RL-based model allows pedestrians to accelerate and slow down to avoid imminent danger (e.g. cars), while obeying human dynamics learnt from in-lab motion capture datasets. Specifically, we propose a hierarchical model consisting of a semantic trajectory policy network that provides a distribution over possible movements, and a human locomotion network that generates 3d human poses in each step. The RL-formulation allows the model to learn even from states that are seldom exhibited in the dataset, utilizing all of the available prior and scene information. Extensive evaluations using both real and simulated data illustrate that the proposed model is on par with recent models such as S-GAN, ST-GAT and S-STGCNN in pedestrian forecasting, while outperforming these in collision avoidance. We also show that our model can be used to plan goal reaching trajectories in urban scenes with dynamic actors.
SlidesLive
Similar Papers
3D Object Detection from Consecutive Monocular Images
Chia-Chun Cheng (National Tsing Hua University)*, Shang-Hong Lai (Microsoft)
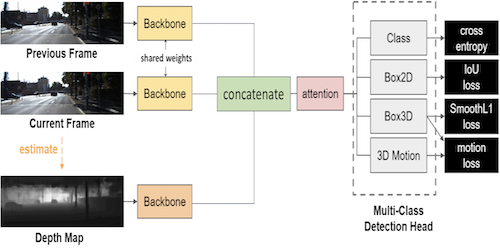
A Two-Stage Minimum Cost Multicut Approach to Self-Supervised Multiple Person Tracking
Kalun Ho (Fraunhofer ITWM)*, Amirhossein Kardoost (University of Mannheim), Franz-Josef Pfreundt (Fraunhofer ITWM), Janis Keuper (hs-offenburg), Margret Keuper (University of Mannheim)
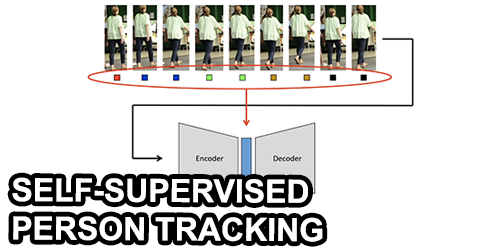
Efficient Large-Scale Semantic Visual Localization in 2D Maps
Tomas Vojir (CMP CTU)*, Ignas Budvytis (Department of Engineering, University of Cambridge), Roberto Cipolla (University of Cambridge)
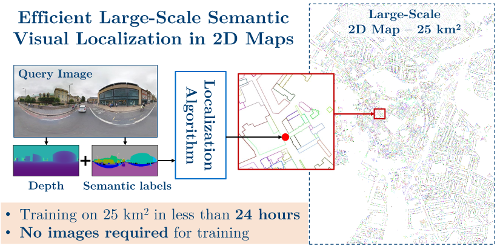