Road Obstacle Detection Method Based on an Autoencoder with Semantic Segmentation
Toshiaki Ohgushi (TOYOTA), Kenji Horiguchi (TOYOTA), Masao Yamanaka (TOYOTA)*
Keywords: Applications of Computer Vision, Vision for X
Abstract:
Accurate detection of road obstacles is vital for ensuring safe autonomous driving, particularly on highways.However, existing methods tend to perform poorly when analyzing road scenes with complex backgrounds, because supervised approaches cannot detect unknown objects that are not included in the training dataset.Hence, in this study, we propose a road obstacle detection method using an autoencoder with semantic segmentation that was trained with only data from normal road scenes.The proposed method requires only a color image captured by a common in-vehicle camera as input. It then creates a resynthesized image using an autoencoder consisting of a semantic image generator as the encoder and a photographic image generator as the decoder.Extensive experiments demonstrate that the performance of the proposed method is comparable to that of existing methods, even without postprocessing. The proposed method with postprocessing outperformed state-of-the-art methods on the Lost and Found dataset.Further, in evaluations using our Highway Anomaly Dataset, which includes actual and synthetic road obstacles, the proposed method significantly outperformed a supervised method that explicitly learns road obstacles.Thus, the proposed machine-learning-based road obstacle detection method is a practical solution that will advance the development of autonomous driving systems.
SlidesLive
Similar Papers
Low-level Sensor Fusion Network for 3D Vehicle Detection using Radar Range-Azimuth Heatmap and Monocular Image
Jinhyeong Kim ( Korea Advanced Institute of Science and Technology), Youngseok Kim (Korea Advanced Institute of Science and Technology (KAIST))*, Dongsuk Kum (Korea Advanced Institute of Science and Technology)
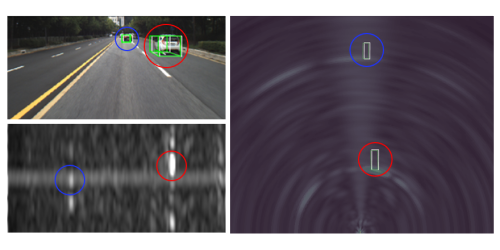
Semantic Synthesis of Pedestrian Locomotion
Maria Priisalu (Lund University)*, Ciprian Paduraru (IMAR), Aleksis Pirinen (Lund University), Cristian Sminchisescu (Lund University)
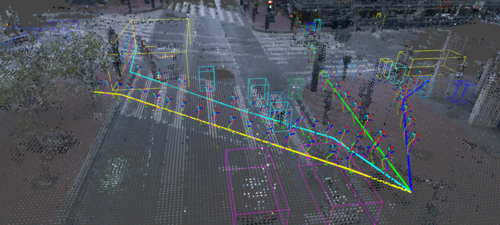
3D Object Detection from Consecutive Monocular Images
Chia-Chun Cheng (National Tsing Hua University)*, Shang-Hong Lai (Microsoft)
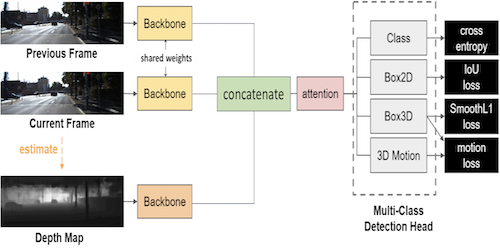