Second Order enhanced Multi-glimpse Attention in Visual Question Answering
Qiang Sun (Fudan University)*, Binghui Xie (Fudan University), Yanwei Fu (Fudan University)
Keywords: Deep Learning for Computer Vision
Abstract:
Visual Question Answering (VQA) is formulated as predicting the answer given an image and question pair. A successful VQA model relies on the information from both visual and textual modalities. Previous endeavours of VQA are made on the good attention mechanism, and multi-modal fusion strategies. For example, most models, till date, are proposed to fuse the multi-modal features based on implicit neural network through cross-modal interactions. To better explore and exploit the information of different modalities, the idea of second order interactions of different modalities, which is prevalent in recommendation system, is re-purposed to VQA in efficiently and explicitly modeling the second order interaction on both the visual and textual features, learned in a shared embedding space. To implement this idea, we propose a novel Second Order enhanced Multi-glimpse Attention model (SOMA) where each glimpse denotes an attention map. SOMA adopts multi-glimpse attention to focus on different contents in the image. With projected the multi-glimpse outputs and question feature into a shared embedding space, an explicit second order feature is constructed to model the interaction on both the intra-modality and cross-modality of features. Furthermore, we advocate a semantic deformation method as data augmentation to generate more training examples in Visual Question Answering. Experimental results on VQA v2.0 and VQA-CP v2.0 have demonstrated the effectiveness of our method. Extensive ablation studies are studied to evaluate the components of the proposed model.
SlidesLive
Similar Papers
Sequential View Synthesis with Transformer
Phong Nguyen-Ha (University of Oulu)*, Lam Huynh ( University of Oulu), Esa Rahtu (Tampere University), Janne Heikkila (University of Oulu, Finland)
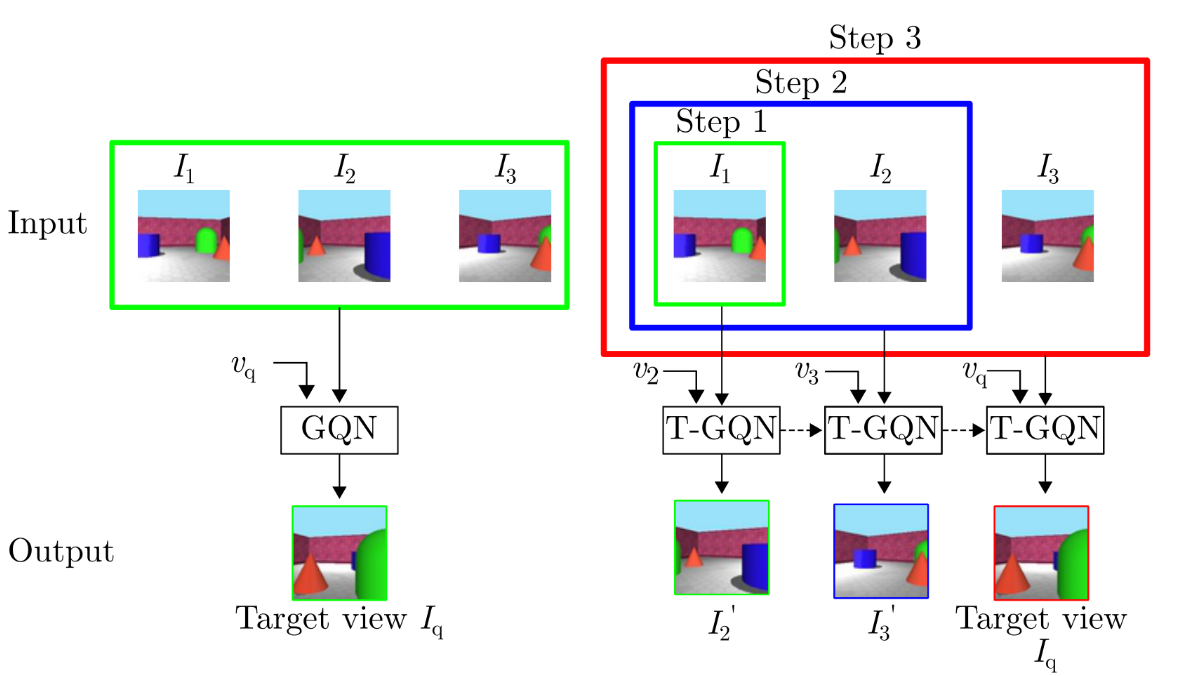
MTNAS: Search Multi-Task Networks for Autonomous Driving
Hao Liu (Beijing Institute of Technology)*, Dong Li (Xilinx), JinZhang Peng (Xilinx), Qingjie Zhao (Beijing Institute of Technology), Lu Tian (Xilinx,Inc.), Yi Shan (Xilinx)
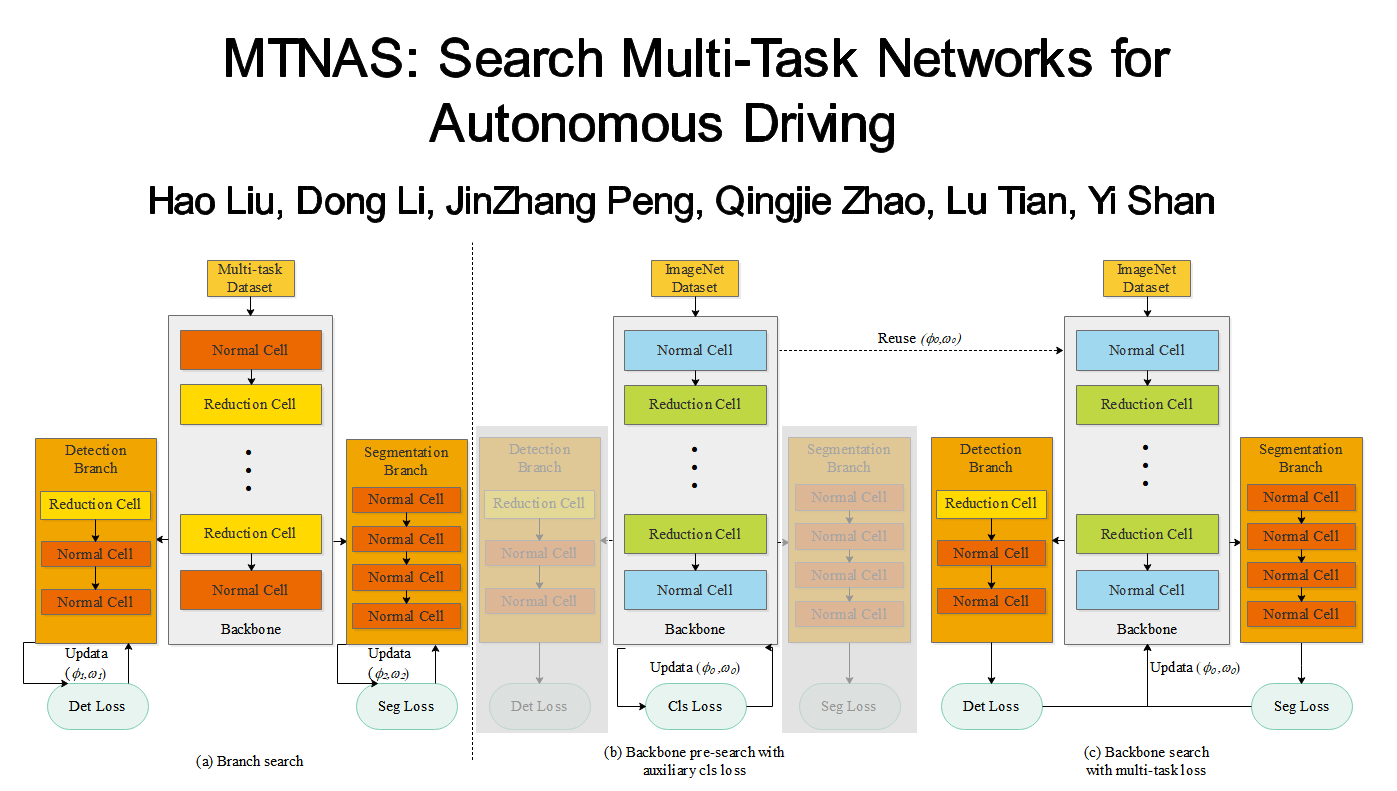
Audiovisual Transformer with Instance Attention for Audio-Visual Event Localization
Yan-Bo Lin (National Taiwan Unviersity)*, Yu-Chiang Frank Wang (National Taiwan University)
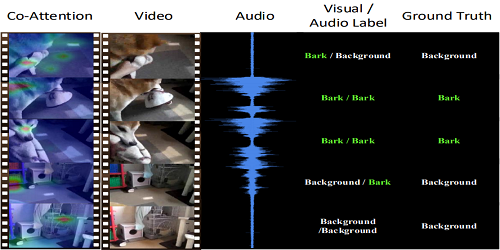