Audiovisual Transformer with Instance Attention for Audio-Visual Event Localization
Yan-Bo Lin (National Taiwan Unviersity)*, Yu-Chiang Frank Wang (National Taiwan University)
Keywords: Applications of Computer Vision, Vision for X
Abstract:
Audio-visual event localization requires one to identify the event label across video frames by jointly observing visual and audio information. To address this task, we propose a deep learning framework of cross-modality co-attention for video event localization. Our proposed audiovisual transformer (AV-transformer) is able to exploit intra and inter-frame visual information, with audio features jointly observed to perform co-attention over the above three modalities. With visual, temporal, and audio information observed across consecutive video frames, our model achieves promising capability in extracting informative spatial/temporal features for improved event localization. Moreover, our model is able to produce instance-level attention, which would identify image regions at the instance level which are associated with the sound/event of interest. Experiments on a benchmark dataset confirm the effectiveness of our proposed framework, with ablation studies performed to verify the design of our propose network model.
SlidesLive
Similar Papers
Second Order enhanced Multi-glimpse Attention in Visual Question Answering
Qiang Sun (Fudan University)*, Binghui Xie (Fudan University), Yanwei Fu (Fudan University)
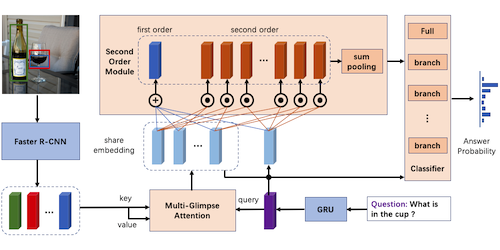
Play Fair: Frame Contributions in Video Models
Will Price (University of Bristol)*, Dima Damen (University of Bristol)
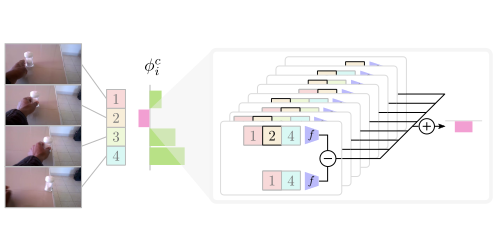
Sequential View Synthesis with Transformer
Phong Nguyen-Ha (University of Oulu)*, Lam Huynh ( University of Oulu), Esa Rahtu (Tampere University), Janne Heikkila (University of Oulu, Finland)
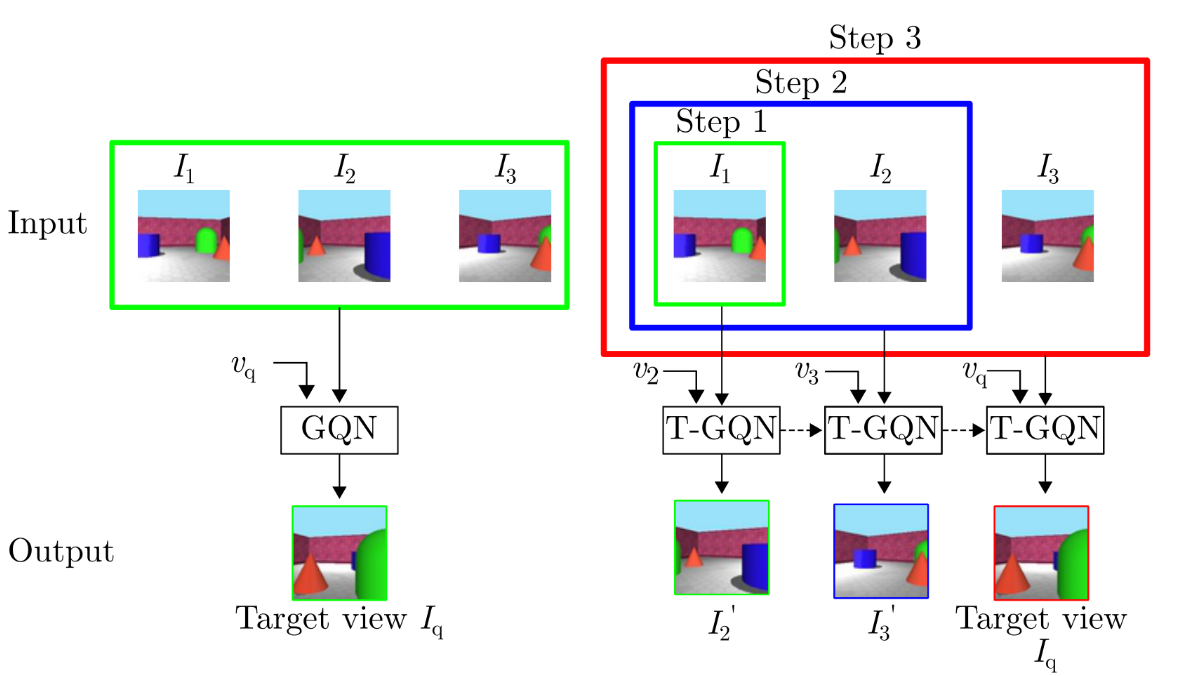