Faster, Better and More Detailed: 3D Face Reconstruction with Graph Convolutional Networks
Shiyang Cheng (Samsung)*, Georgios Tzimiropoulos (Samsung AI), Jie Shen (Imperial College London), Maja Pantic (Samsung AI Centre Cambridge/ Imperial College London )
Keywords: Face, Pose, Action, and Gesture
Abstract:
This paper addresses the problem of 3D face reconstruction from a single image. While available solutions for addressing this problem do exist, to our knowledge, we propose the very first approach which is robust, lightweight and detailed i.e. it can reconstruct fine facial details. Our system is extremely simple and consists of 3 key components: (a) a lightweight non-parametric decoder based on Graph Convolutional Networks (GCNs) trained in a supervised manner to reconstruct coarse facial geometry from image-based ResNet features. (b) An extremely lightweight (35K parameters) subnetwork -- also based on GCNs -- which is trained in an unsupervised manner to refine the output of the first network. (c) A novel feature-sampling mechanism and adaptation layer which injects fine details from the ResNet features of the first network into the second one. Overall, our system is the first one (to our knowledge) to reconstruct detailed facial geometry relying solely on GCNs. We exhaustively compare our method with 7 state-of-the-art methods on 3 datasets reporting state-of-the-art results for all of our experiments, both qualitatively and quantitatively, with our approach being, at the same time, significantly faster.
SlidesLive
Similar Papers
Sketch-to-Art: Synthesizing Stylized Art Images From Sketches
Bingchen Liu (Rutgers, The State University of New Jersey)*, Kunpeng Song (Rutgers University), Yizhe Zhu (Rutgers University ), Ahmed Elgammal (-)
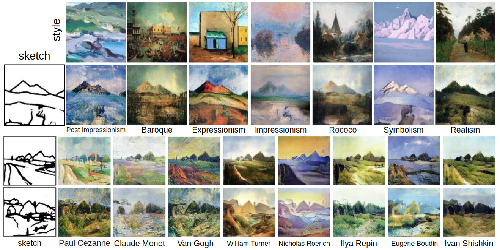
3D Object Detection and Pose Estimation of Unseen Objects in Color Images with Local Surface Embeddings
Giorgia Pitteri (Université de Bordeaux, LaBRI)*, Aureélie Bugeau (University of Bordeaux), Slobodan Ilic (Siemens AG), Vincent Lepetit (Ecole des Ponts ParisTech)
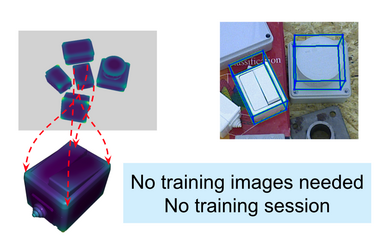
Human Motion Deblurring using Localized Body Prior
Jonathan Samuel Lumentut (Inha University), Joshua Santoso (Inha University), In Kyu Park (Inha University)*
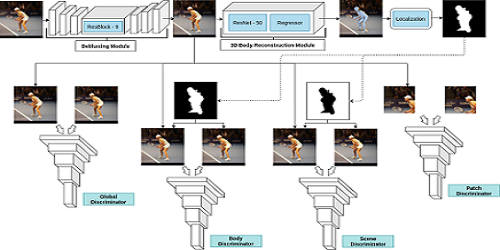