3D Object Detection and Pose Estimation of Unseen Objects in Color Images with Local Surface Embeddings
Giorgia Pitteri (Université de Bordeaux, LaBRI)*, Aureélie Bugeau (University of Bordeaux), Slobodan Ilic (Siemens AG), Vincent Lepetit (Ecole des Ponts ParisTech)
Keywords: 3D Computer Vision
Abstract:
We present an approach for detecting and estimating the 3D poses of objects in images that requires only an untextured CAD model and no training phase for new objects. Our approach combines Deep Learning and 3D geometry: It relies on an embedding of local 3D geometry to match the CAD models to the input images. For points at the surface of objects, this embedding can be computed directly from the CAD model; for image locations, we learn to predict it from the image itself. This establishes correspondences between 3D points on the CAD model and 2D locations of the input images. However, many of these correspondences are ambiguous as many points may have similar local geometries. We show that we can use Mask-RCNN in a class-agnostic way to detect the new objects without retraining and thus drastically limit the number of possible correspondences. We can then robustly estimate a 3D pose from these discriminative correspondences using a RANSAC-like algorithm. We demonstrate the performance of this approach on the T-LESS dataset, by using a small number of objects to learn the embedding and testing it on the other objects. Our experiments show that our method is on par or better than previous methods.
SlidesLive
Similar Papers
Learning 3D Face Reconstruction with a Pose Guidance Network
Pengpeng Liu (The Chinese University of Hong Kong)*, Xintong Han (Malong Technologies), Michael Lyu (The Chinese University of Hong Kong), Irwin King (The Chinese University of Hong Kong), Jia Xu (Huya AI)
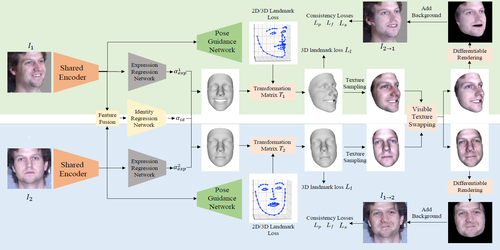
3D Guided Weakly Supervised Semantic Segmentation
Weixuan Sun (Australian National University, Data61 )*, Jing Zhang (Australian National University), Nick Barnes (ANU)
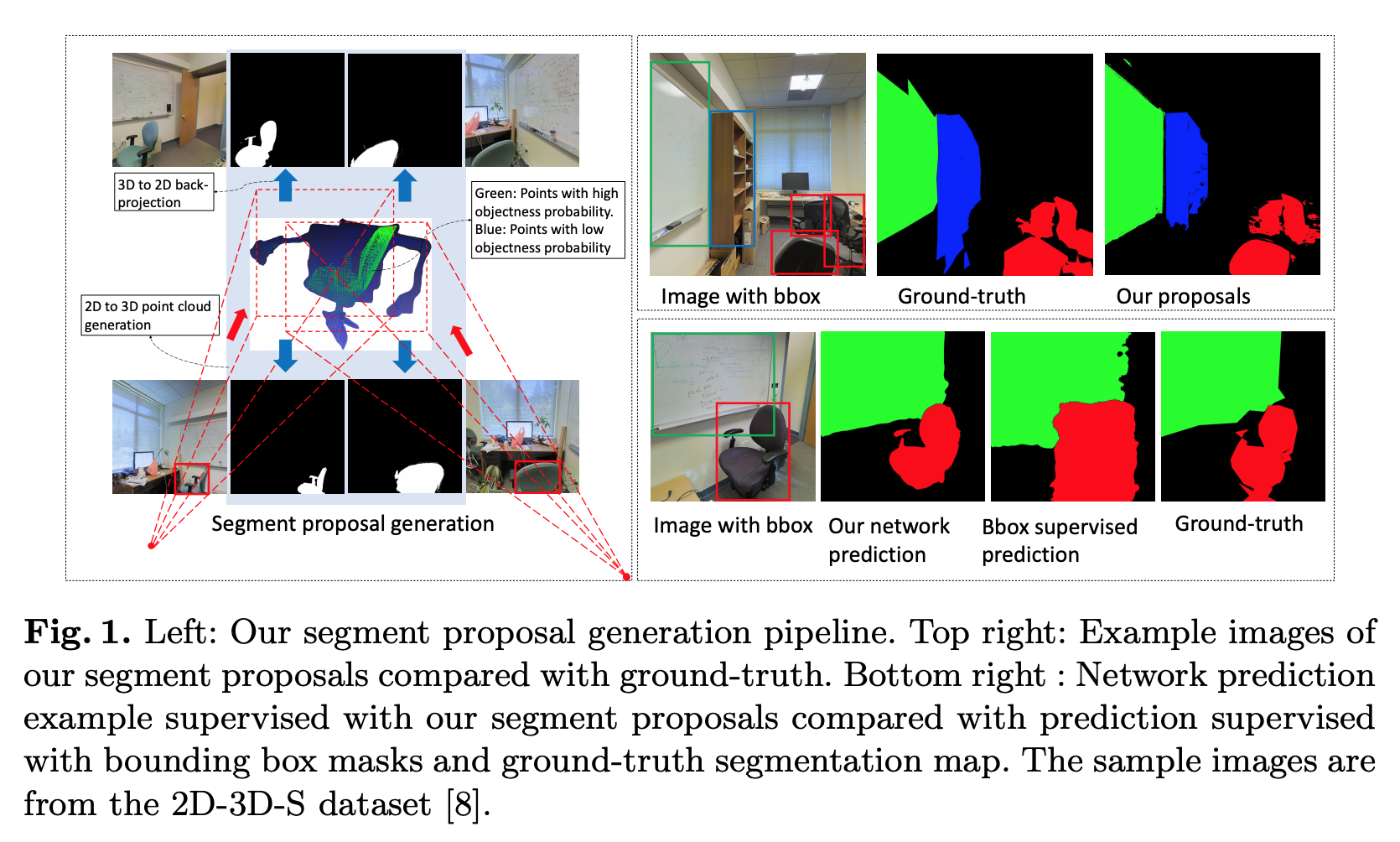
Adaptive Spotting: Deep Reinforcement Object Search in 3D Point Clouds
Onkar Krishna (NTT Corporation, Japan)*, Go Irie (NTT Corporation), Xiaomeng Wu (NTT Corporation), Takahito Kawanishi (NTT Corporation), Kunio Kashino (NTT Communication Science Laboratories)
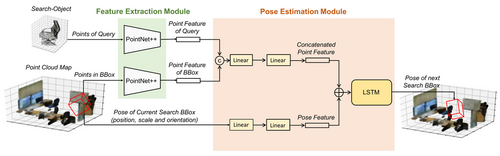