Sketch-to-Art: Synthesizing Stylized Art Images From Sketches
Bingchen Liu (Rutgers, The State University of New Jersey)*, Kunpeng Song (Rutgers University), Yizhe Zhu (Rutgers University ), Ahmed Elgammal (-)
Keywords: Applications of Computer Vision, Vision for X
Abstract:
We propose a new approach for synthesizing fully detailed art-stylized images from sketches. Given a sketch, with no semantic tagging, and a reference image of a specific style, the model can synthesize meaningful details with colors and textures. Based on the GAN framework, the model consists of three novel modules designed explicitly for better artistic style capturing and generation. To enforce the content faithfulness, we introduce the dual-masked mechanism which directly shapes the feature maps according to sketch. To capture more artistic style aspects, we design feature-map transformation for a better style consistency to the reference image. Finally, an inverse process of instance-normalization disentangles the style and content information and further improves the synthesis quality. Experiments demonstrate a significant qualitative and quantitative boost over baseline models based on previous state-of-the-art techniques, modified for the proposed task (17% better Frechet Inception distance and 18% better style classification score). Moreover, the lightweight design of the proposed modules enables the high-quality synthesis at 512 * 512 resolution.
SlidesLive
Similar Papers
Faster, Better and More Detailed: 3D Face Reconstruction with Graph Convolutional Networks
Shiyang Cheng (Samsung)*, Georgios Tzimiropoulos (Samsung AI), Jie Shen (Imperial College London), Maja Pantic (Samsung AI Centre Cambridge/ Imperial College London )
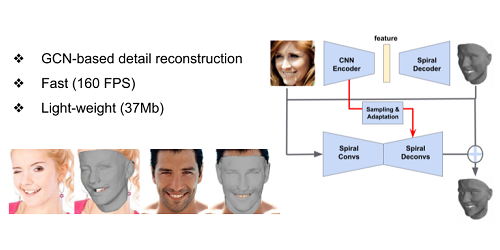
Reconstructing Creative Lego Models
George Tattersall (University of York)*, Dizhong Zhu (University of York), William A. P. Smith (University of York), Sebastian Deterding (University of York), Patrik Huber (University of York)
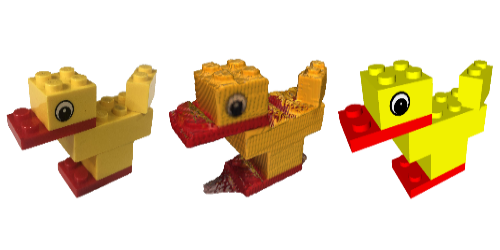
3D Object Detection and Pose Estimation of Unseen Objects in Color Images with Local Surface Embeddings
Giorgia Pitteri (Université de Bordeaux, LaBRI)*, Aureélie Bugeau (University of Bordeaux), Slobodan Ilic (Siemens AG), Vincent Lepetit (Ecole des Ponts ParisTech)
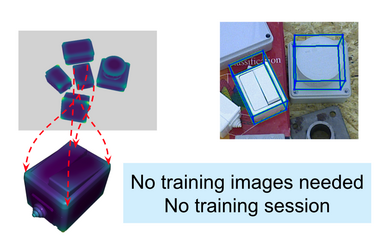