Unified Application of Style Transfer for Face Swapping and Reenactment
Le Minh Ngo (University of Amsterdam)*, Christian aan de Wiel (3DUniversum), Sezer Karaoglu (University of Amsterdam), Theo Gevers (University of Amsterdam)
Keywords: Face, Pose, Action, and Gesture
Abstract:
Face reenactment and face swap have gained a lot of attention due to their broad range of applications in computer vision. Although both tasks share similar objectives (e.g. manipulating expression and pose), existing methods do not explore the benefits of combining these two tasks.In this paper, we introduce a unified end-to-end pipeline for face swapping and reenactment. We propose a novel approach to isolated disentangled representation learning of specific visual attributes in an unsupervised manner. A combination of the proposed training losses allows us to synthesize results in a one-shot manner. The proposed method does not require subject-specific training.We compare our method against state-of-the-art methods for multiple public datasets of different complexities. The proposed method outperforms other SOTA methods in terms of realistic-looking face images.
SlidesLive
Similar Papers
DoFNet: Depth of Field Difference Learning for Detecting Image Forgery
Yonghyun Jeong (Samsung SDS)*, Jongwon Choi (Chung-Ang University), Doyeon Kim (SamsungSDS), Sehyeon Park (Samsung SDS), Minki Hong (Samsung SDS), Changhyun Park (Samsung SDS), Seungjai Min (Samsung SDS), Youngjune Gwon (Samsung SDS)
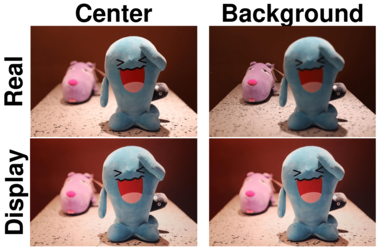
Transforming Multi-Concept Attention into Video Summarization
Yen-Ting Liu (National Taiwan University)*, Yu-Jhe Li (Carnegie Mellon University), Yu-Chiang Frank Wang (National Taiwan University)
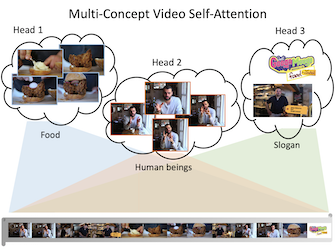
Channel Recurrent Attention Networks for Video Pedestrian Retrieval
Pengfei Fang (The Australian National University)*, Pan Ji (OPPO US Research Center), Jieming Zhou (The Australian National University), Lars Petersson (Data61/CSIRO), Mehrtash Harandi (Monash University)
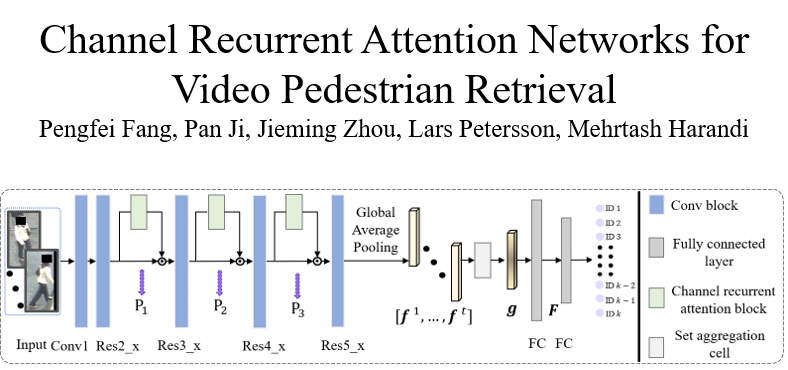