MMD based Discriminative Learning for Face Forgery Detection
Jian Han (University of Amsterdam)*, Theo Gevers (University of Amsterdam)
Keywords: Face, Pose, Action, and Gesture
Abstract:
Face forensic detection is to distinguish manipulated from pristine face images. The main drawback of existing face forensics detection methods is their limited generalization ability due to differences in domains. Furthermore, artifacts such as imaging variations or face attributes do not persistently exist among all generated results for a single generation method.Therefore, in this paper, we propose a novel framework to address the domain gap induced by multiple deep fake datasets. To this end, the maximum mean discrepancy (MMD) loss is incorporated to align the different feature distributions. The center and triplet losses are added to enhance generalization. This addition ensures that the learned features are shared by multiple domains and provides better generalization abilities to unseen deep fake samples. Evaluations on various deep fake benchmarks (DFTIMIT, UADFV, Celeb-DF, and FaceForensics++) show that the proposed method achieves the best overall performance. An ablation study is performed to investigate the effect of the different components and style transfer losses.
SlidesLive
Similar Papers
Localin Reshuffle Net: Toward Naturally and Efficiently Facial Image Blending
Chengyao Zheng (Southeast Univeristy), Siyu Xia (Southeast University, China), Joseph Robinson (Northeastern University)*, Changsheng Lu (Shanghai Jiao Tong University), Wayne Wu (Tsinghua University), Chen Qian (SenseTime), Ming Shao (University of Massachusetts Dartmouth)
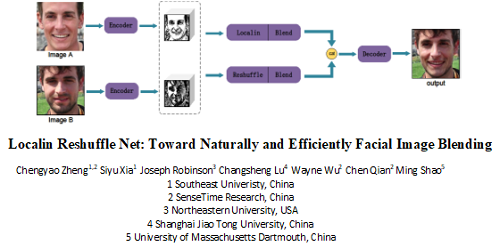
Adversarial Image Composition with Auxiliary Illumination
Fangneng Zhan (Nanyang Technological University), Shijian Lu (Nanyang Technological University)*, Changgong Zhang (Alibaba Group), Feiying Ma (Alibaba), Xuansong Xie (Alibaba)
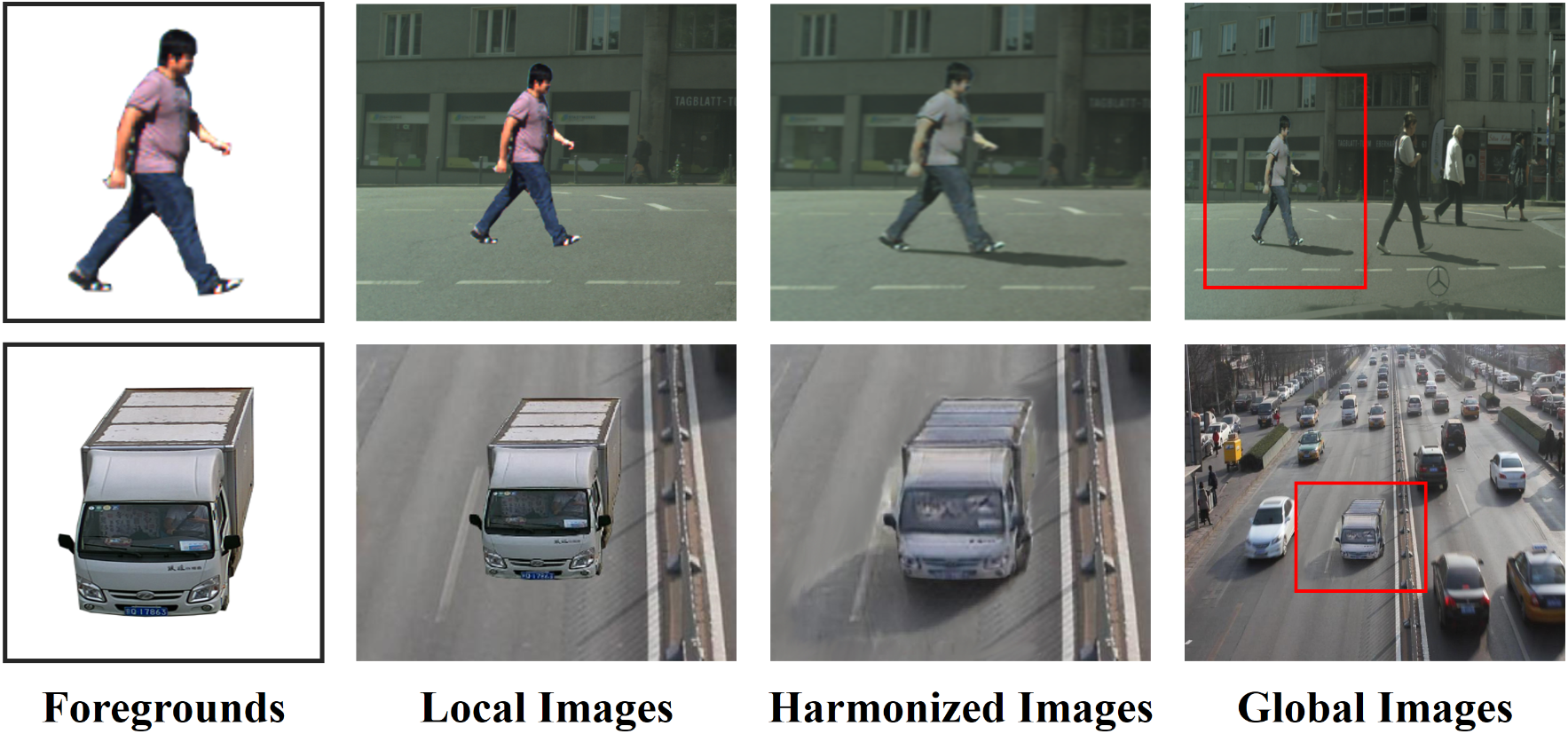
MLIFeat: Multi-level information fusion based deep local features
Yuyang Zhang (Institute of Automation, Chinese Academy of Sciences, University of Chinese Academy of Sciences), Jinge Wang (Megvii), Shibiao Xu (Institute of Automation, Chinese Academy of Sciences)*, Xiao Liu (Megvii Inc), Xiaopeng Zhang (Institute of Automation, Chinese Academy of Sciences)
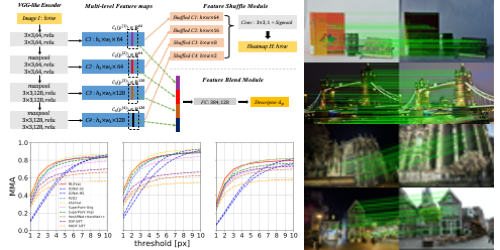