Localin Reshuffle Net: Toward Naturally and Efficiently Facial Image Blending
Chengyao Zheng (Southeast Univeristy), Siyu Xia (Southeast University, China), Joseph Robinson (Northeastern University)*, Changsheng Lu (Shanghai Jiao Tong University), Wayne Wu (Tsinghua University), Chen Qian (SenseTime), Ming Shao (University of Massachusetts Dartmouth)
Keywords: Face, Pose, Action, and Gesture
Abstract:
The blending of facial images is an effective way to fuse attributes such that the synthesis is robust to the finer details (e.g., periocular-region, nostrils, hairlines). Specifically, facial blending aims to transfer the style of a source image to a target such that violations in the natural appearance are minimized. Despite the many practical applications, facial image blending remains mostly unexplored with the reasons being two-fold: 1) the lack of quality paired data for supervision and 2) facial synthesizers (i.e., the models) are sensitive to small variations in lighting, texture, resolution and age. We address the reasons for the bottleneck by first building Facial Pairs to Blend (FPB) dataset, which was generated through our facial attribute optimization algorithm. Then, we propose an effective normalization scheme to capture local statistical information during blending: namely, Local Instance Normalization (LAN). Lastly, a novel local-reshuffle-layer is designed to map local patches in the feature space, which can be learned in an end-to-end fashion with dedicated loss. This new layer is essential for the proposed Localin Reshuffle Network (LRNet). Extensive experiments, and both quantitative and qualitative results, demonstrate that our approach outperforms existing methods.
SlidesLive
Similar Papers
MMD based Discriminative Learning for Face Forgery Detection
Jian Han (University of Amsterdam)*, Theo Gevers (University of Amsterdam)
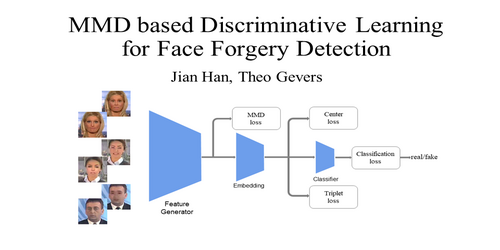
Adversarial Image Composition with Auxiliary Illumination
Fangneng Zhan (Nanyang Technological University), Shijian Lu (Nanyang Technological University)*, Changgong Zhang (Alibaba Group), Feiying Ma (Alibaba), Xuansong Xie (Alibaba)
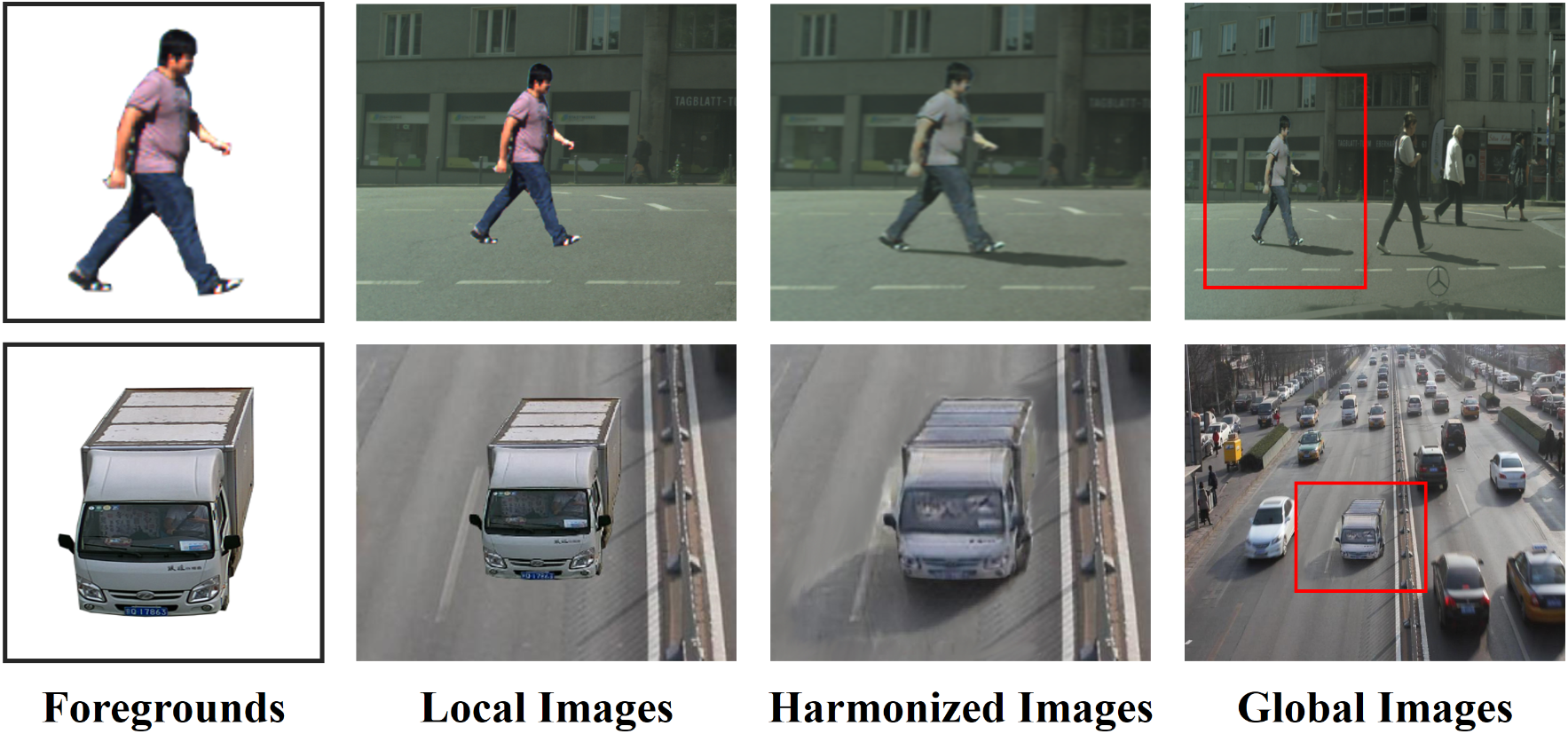
Low-light Color Imaging via Dual Camera Acquisition
Peiyao Guo (Nanjing University), Zhan Ma (Nanjing University)*
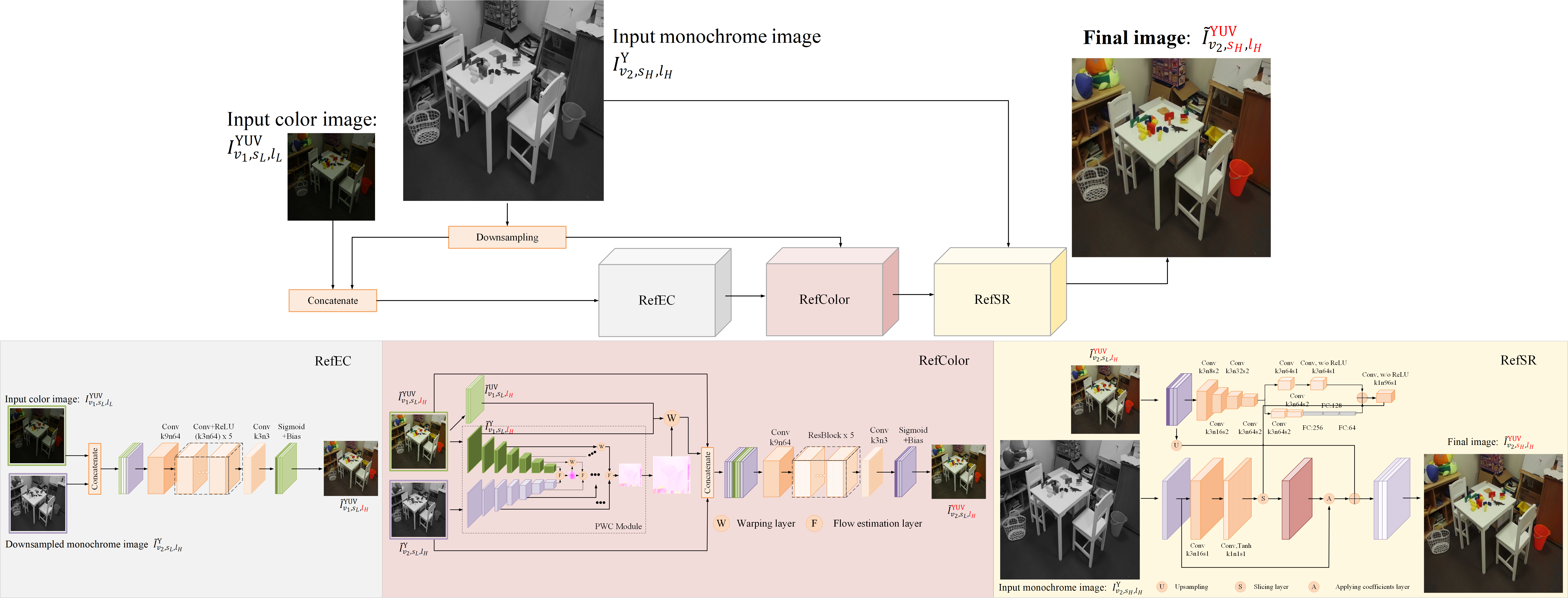