Adversarially Robust Deep Image Super-Resolution using Entropy Regularization
Jun-Ho Choi (Yonsei University), Huan Zhang (UCLA), Jun-Hyuk Kim (Yonsei University), Cho-Jui Hsieh (UCLA), Jong-Seok Lee ("Yonsei University, Korea")*
Keywords: Deep Learning for Computer Vision
Abstract:
Image super-resolution has been widely employed in various applications with boosted performance thanks to the deep learning techniques. However, many deep learning-based models are highly vulnerable to adversarial attacks, which is also applied to super-resolution models in recent studies. In this paper, we propose a defense method that is formulated as an entropy regularization loss for model training, which can be augmented to the original training loss of super-resolution models. We show that various state-of-the-art super-resolution models trained with our defense method are more robust against adversarial attacks than their original versions. To the best of our knowledge, this is the first attempt of adversarial defense for deep super-resolution models.
SlidesLive
Similar Papers
Towards Fast and Robust Adversarial Training for Image Classification
Erh-Chung Chen (National Tsing Hua University)*, Che-Rung Lee (National Tsing Hua University )
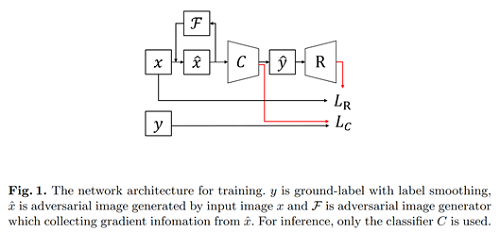
Learning Local Feature Descriptors for Multiple Object Tracking
Dmytro Borysenko (Samsung R&D Institute Ukraine), Dmytro Mykheievskyi (Samsung R&D Institute Ukraine), Viktor Porokhonskyy (Samsung Research&Development Institute Ukraine (SRK))*
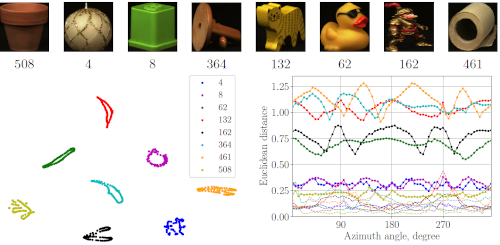
RAF-AU Database: In-the-Wild Facial Expressions with Subjective Emotion Judgement and Objective AU Annotations
Wen-Jing Yan (JD Digits)*, Shan Li (Beijing University of Posts and Telecommunications), Chengtao Que (JD Digits), Jiquan Pei (JD Digits), Weihong Deng (Beijing University of Posts and Telecommunications)
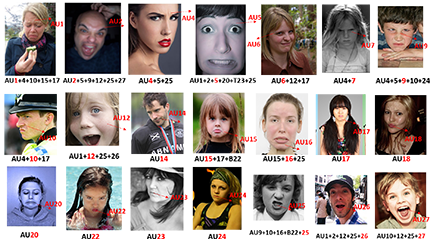