Adversarial Semi-Supervised Multi-Domain Tracking
Kourosh Meshgi (RIKEN AIP)*, Maryam Sadat Mirzaei (Riken AIP / Kyoto University)
Keywords: Motion and Tracking
Abstract:
Neural networks for multi-domain learning empowers an effective combination of information from different domains by sharing and co-learning the parameters. In visual tracking, the emerging features in shared layers of a multi-domain tracker, trained on various sequences, are crucial for tracking the target in unseen videos. Yet, in a fully shared architecture, some of the emerging features are useful only in a specific domain, reducing the generalization of the learned feature representation. We propose a semi-supervised learning scheme to separate domain-invariant and domain-specific features using adversarial learning, to encourage mutual exclusion between them, and to leverage self-supervised learning for enhancing the shared features using the unlabeled reservoir. By employing these features and training dedicated layers for each sequence, we build a tracker that performs exceptionally on different types of videos.
SlidesLive
Similar Papers
Interpreting Video Features: A Comparison of 3D Convolutional Networks and Convolutional LSTM Networks
Joonatan Mänttäri (KTH Royal Institute of Technology), Sofia Broomé (KTH Royal Institute of Technology)*, John Folkesson (KTH Royal Institute of Technology), Hedvig Kjellström (KTH Royal Institute of Technology)
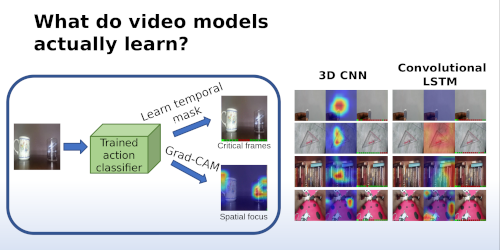
Contextual Semantic Interpretability
Diego Marcos (Wageningen University)*, Ruth Fong (University of Oxford), Sylvain Lobry (Wageningen University and Research), Rémi Flamary (Université Côte d’Azur), Nicolas Courty (UBS), Devis Tuia (Wageningen University and Research)
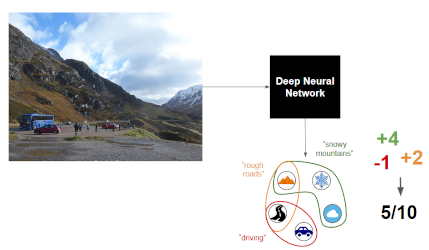
ERIC: Extracting Relations Inferred from Convolutions
Joe Townsend (Fujitsu Laboratories of Europe LTD)*, Theodoros Kasioumis (Fujitsu Laboratories of Europe LTD), Hiroya Inakoshi (Fujitsu Laboratories of Europe)
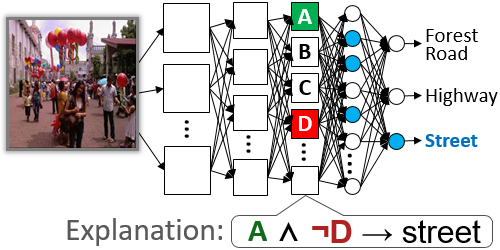