Interpreting Video Features: A Comparison of 3D Convolutional Networks and Convolutional LSTM Networks
Joonatan Mänttäri (KTH Royal Institute of Technology), Sofia Broomé (KTH Royal Institute of Technology)*, John Folkesson (KTH Royal Institute of Technology), Hedvig Kjellström (KTH Royal Institute of Technology)
Keywords: Video Analysis and Event Recognition
Abstract:
A number of techniques for interpretability have been presented for deep learning in computer vision, typically with the goal of understanding what the networks have based their classification on. However, interpretability for deep video architectures is still in its infancy and we do not yet have a clear concept of how to decode spatiotemporal features. In this paper, we present a study comparing how 3D convolutional networks and convolutional LSTM networks learn features across temporally dependent frames. This is the first comparison of two video models that both convolve to learn spatial features but have principally different methods of modeling time. Additionally, we extend the concept of meaningful perturbation introduced by Fong & Vedaldi to the temporal dimension, to identify the temporal part of a sequence most meaningful to the network for a classification decision. Our findings indicate that the 3D convolutional model concentrates on shorter events in the input sequence, and places its spatial focus on fewer, contiguous areas.
SlidesLive
Similar Papers
Adversarial Semi-Supervised Multi-Domain Tracking
Kourosh Meshgi (RIKEN AIP)*, Maryam Sadat Mirzaei (Riken AIP / Kyoto University)
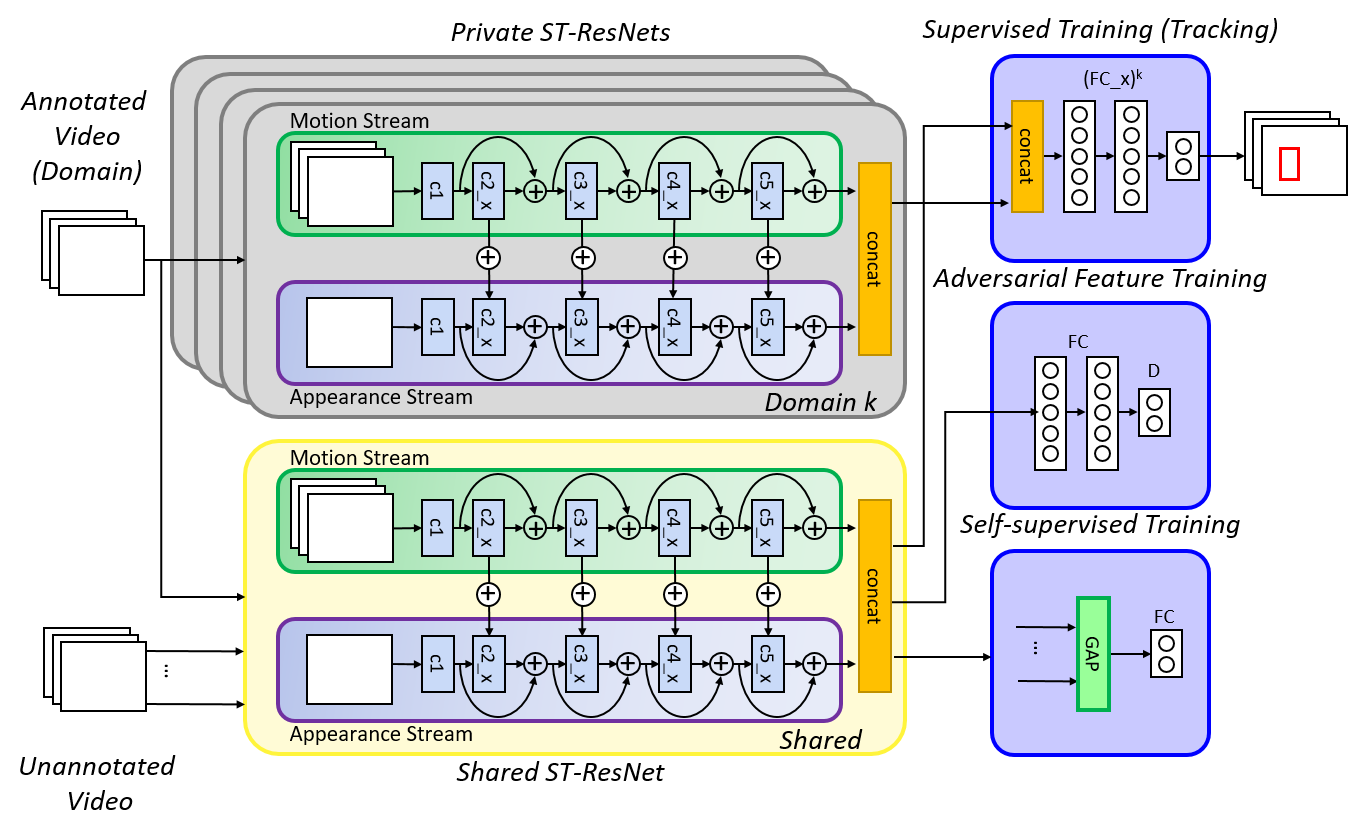
Contextual Semantic Interpretability
Diego Marcos (Wageningen University)*, Ruth Fong (University of Oxford), Sylvain Lobry (Wageningen University and Research), Rémi Flamary (Université Côte d’Azur), Nicolas Courty (UBS), Devis Tuia (Wageningen University and Research)
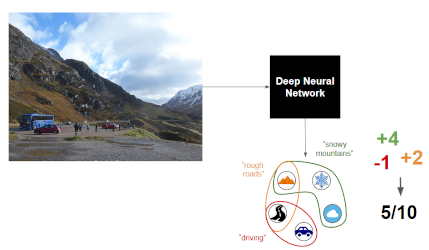
ERIC: Extracting Relations Inferred from Convolutions
Joe Townsend (Fujitsu Laboratories of Europe LTD)*, Theodoros Kasioumis (Fujitsu Laboratories of Europe LTD), Hiroya Inakoshi (Fujitsu Laboratories of Europe)
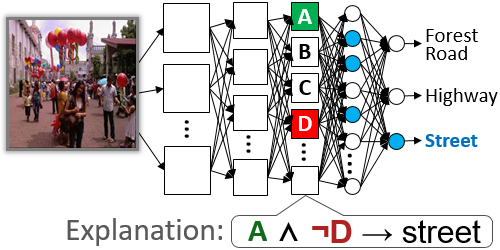