3D Human Motion Estimation via Motion Compression and Refinement
Zhengyi Luo (Carnegie Mellon University)*, S. Alireza Golestaneh (Carnegie Mellon University), Kris M. Kitani (Carnegie Mellon University)
Keywords: Face, Pose, Action, and Gesture
Abstract:
We develop a technique for generating smooth and accurate 3D human pose and motion estimates from RGB video sequences. Our technique, which we call Motion Estimation via Variational Autoencoder (MEVA), decomposes a temporal sequence of human motion into a smooth motion representation using auto-encoder-based motion compression and a residual representation learned through motion refinement. This two-step encoding process of human motion can represent a wide variety of general human motions while also retaining person-specific motion details. Experiments show that our method produces both smooth and accurate 3D human pose and motion estimates.
SlidesLive
Similar Papers
Visually Guided Sound Source Separation using Cascaded Opponent Filter Network
Lingyu Zhu (Tampere University)*, Esa Rahtu (Tampere University)
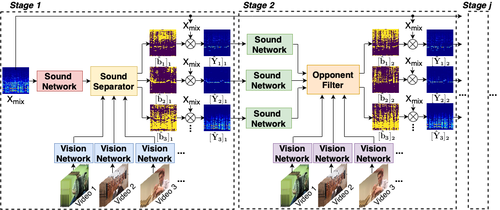
Recursive Bayesian Filtering for Multiple Human Pose Tracking from Multiple Cameras
Oh-Hun Kwon (University of Bonn), Julian Tanke (University of Bonn)*, Jürgen Gall (University of Bonn)
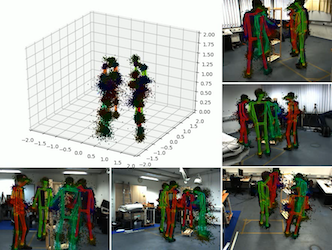
Learning Multi-Instance Sub-pixel Point Localization
Julien Schroeter (Cardiff University)*, Tinne Tuytelaars (KU Leuven), Kirill Sidorov (Cardiff University), David Marshall (Cardiff University)
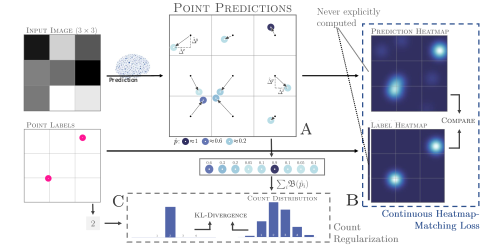