Learning Multi-Instance Sub-pixel Point Localization
Julien Schroeter (Cardiff University)*, Tinne Tuytelaars (KU Leuven), Kirill Sidorov (Cardiff University), David Marshall (Cardiff University)
Keywords: Biomedical Image Analysis
Abstract:
In this work, we propose a novel approach that allows for the end-to-end learning of multi-instance point detection with inherent sub-pixel precision capabilities. To infer unambiguous localization estimates, our model relies on three components: the continuous prediction capabilities of offset-regression-based models, the finer-grained spatial learning ability of a novel continuous heatmap matching loss function introduced to that effect, and the prediction sparsity ability of count-based regularization. We demonstrate strong sub-pixel localization accuracy on single molecule localization microscopy and checkerboard corner detection, and improved sub-frame event detection performance in sport videos.
SlidesLive
Similar Papers
Visually Guided Sound Source Separation using Cascaded Opponent Filter Network
Lingyu Zhu (Tampere University)*, Esa Rahtu (Tampere University)
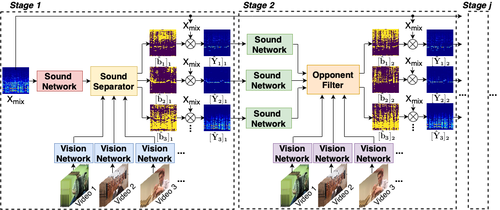
Dense Pixel-wise Micro-motion Estimation of Object Surface by using Low Dimensional Embedding of Laser Speckle Pattern
Ryusuke Sagawa ("AIST, Japan")*, Yusuke Higuchi (Kyushu University), Hiroshi Kawasaki (Kyushu univ.), Ryo Furukawa (Hiroshima city univ.), Takahiro Ito (AIST)
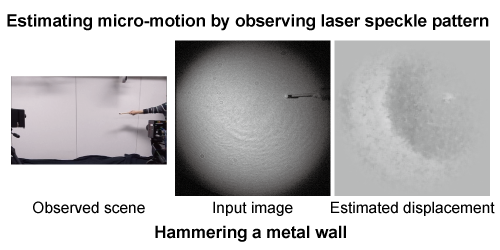
Explaining an image classifier's decisions using generative models
Chirag Agarwal (UIC), Anh Nguyen (Auburn University)*
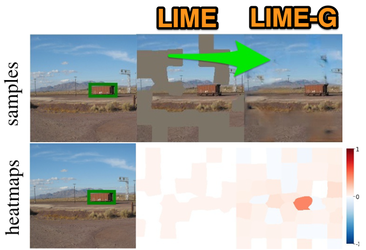