HPGCNN: Hierarchical Parallel Group Convolutional Neural Networks for Point Clouds Processing
Jisheng Dang (Lanzhou Jiaotong University), Jun Yang (School of Electronic and Information Engineering, Lanzhou Jiaotong University)*
Keywords: 3D Computer Vision
Abstract:
To achieve high performance but less complexity for point clouds processing, we introduce HPGCNN, an efficient and lightweight neural architecture. The key component in our approach is the Hierarchical Parallel Group Convolution(HPGConv) operation. It can capture both the discriminative independent single-point features and local geometric features of point clouds at the same time to enhance the richness of the features with less redundant information by designing two hierarchical parallel group convolutions, which is helpful to recognize elusive shapes. To significantly further reduce complexity and natively prevent overfitting, we use global average pooling and a full connected layer instead of the traditional three full connected layers for classification. Moreover, to further capture the contextual fine-grained features with higher-level semantics, we introduce a novel multi-semantic scale strategy to progressively increase the receptive field of each local area through the information communication of local areas of different scales. Extensive experiments show that our HPGCNN clearly surpasses state-of-the-art approaches for point clouds classification dataset ModelNet40 and large scale semantic segmentation datasets ShapeNet Parts, S3DIS, vKITTI and SemanticKITTI in terms of accuracy and complexity.
SlidesLive
Similar Papers
Lossless Image Compression Using a Multi-Scale Progressive Statistical Model
Honglei Zhang (Nokia Technologies)*, Francesco Cricri (Nokia Technologies), Hamed R. Tavakoli (Nokia Technologies), Nannan Zou (Tampere University), Emre Aksu (Nokia Technologies), Miska M. Hannuksela (Nokia Technologies)

Bridging Adversarial and Statistical Domain Transfer via Spectral Adaptation Networks
Christoph Raab (FHWS)*, Philipp Väth (FHWS), Peter Meier (FHWS), Frank-Michael Schleif (FHWS)
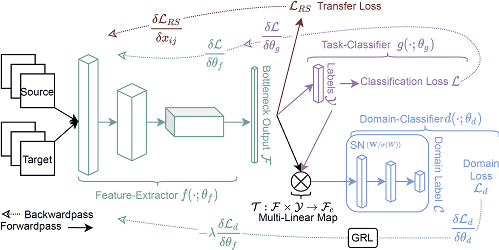
To filter prune, or to layer prune, that is the question
Sara Elkerdawy (University of Alberta)*, Mostafa Elhoushi (Huawei Technologies), Abhineet Singh (University of Alberta), Hong Zhang (University of Alberta), Nilanjan Ray (University of Alberta)
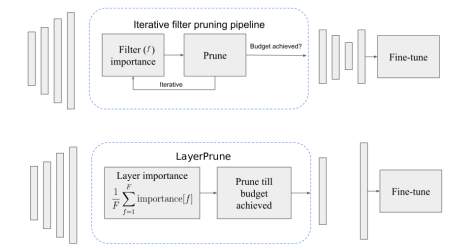