Lossless Image Compression Using a Multi-Scale Progressive Statistical Model
Honglei Zhang (Nokia Technologies)*, Francesco Cricri (Nokia Technologies), Hamed R. Tavakoli (Nokia Technologies), Nannan Zou (Tampere University), Emre Aksu (Nokia Technologies), Miska M. Hannuksela (Nokia Technologies)
Keywords: Statistical Methods and Learning
Abstract:
Lossless image compression is an important technique for im-age storage and transmission when information loss is not allowed. Withthe fast development of deep learning techniques, deep neural networkshave been used in this field to achieve a higher compression rate. Meth-ods based on pixel-wise autoregressive statistical models have showngood performance. However, the sequential processing way prevents thesemethods to be used in practice. Recently, multi-scale autoregressive mod-els have been proposed to address this limitation. Multi-scale approachescan use parallel computing systems efficiently and build practical sys-tems. Nevertheless, these approaches sacrifice compression performancein exchange for speed. In this paper, we propose a multi-scale progressivestatistical model that takes advantage of the pixel-wise approach and themulti-scale approach. We developed a flexible mechanism where the pro-cessing order of the pixels can be adjusted easily. Our proposed methodoutperforms the state-of-the-art lossless image compression methods ontwo large benchmark datasets by a significant margin without degradingthe inference speed dramatically.
SlidesLive
Similar Papers
CS-MCNet:A Video Compressive Sensing Reconstruction Network with Interpretable Motion Compensation
Bowen Huang (Fudan University)*, Jinjia Zhou (Hosei University), Xiao Yan (Fudan University), Ming'e Jing (Fudan University), Rentao Wan (Fudan University), Yibo Fan (Fudan University)
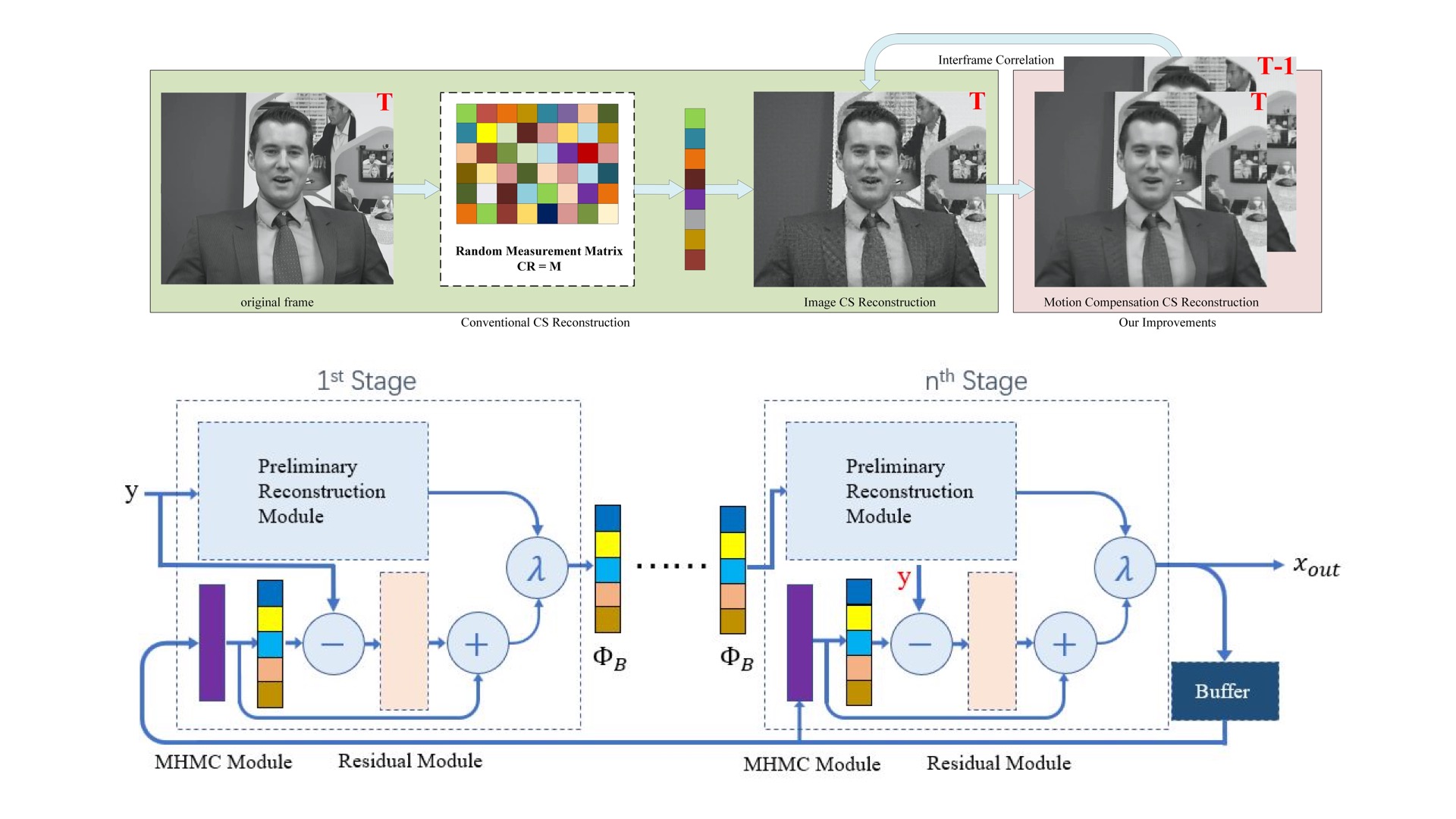
HPGCNN: Hierarchical Parallel Group Convolutional Neural Networks for Point Clouds Processing
Jisheng Dang (Lanzhou Jiaotong University), Jun Yang (School of Electronic and Information Engineering, Lanzhou Jiaotong University)*
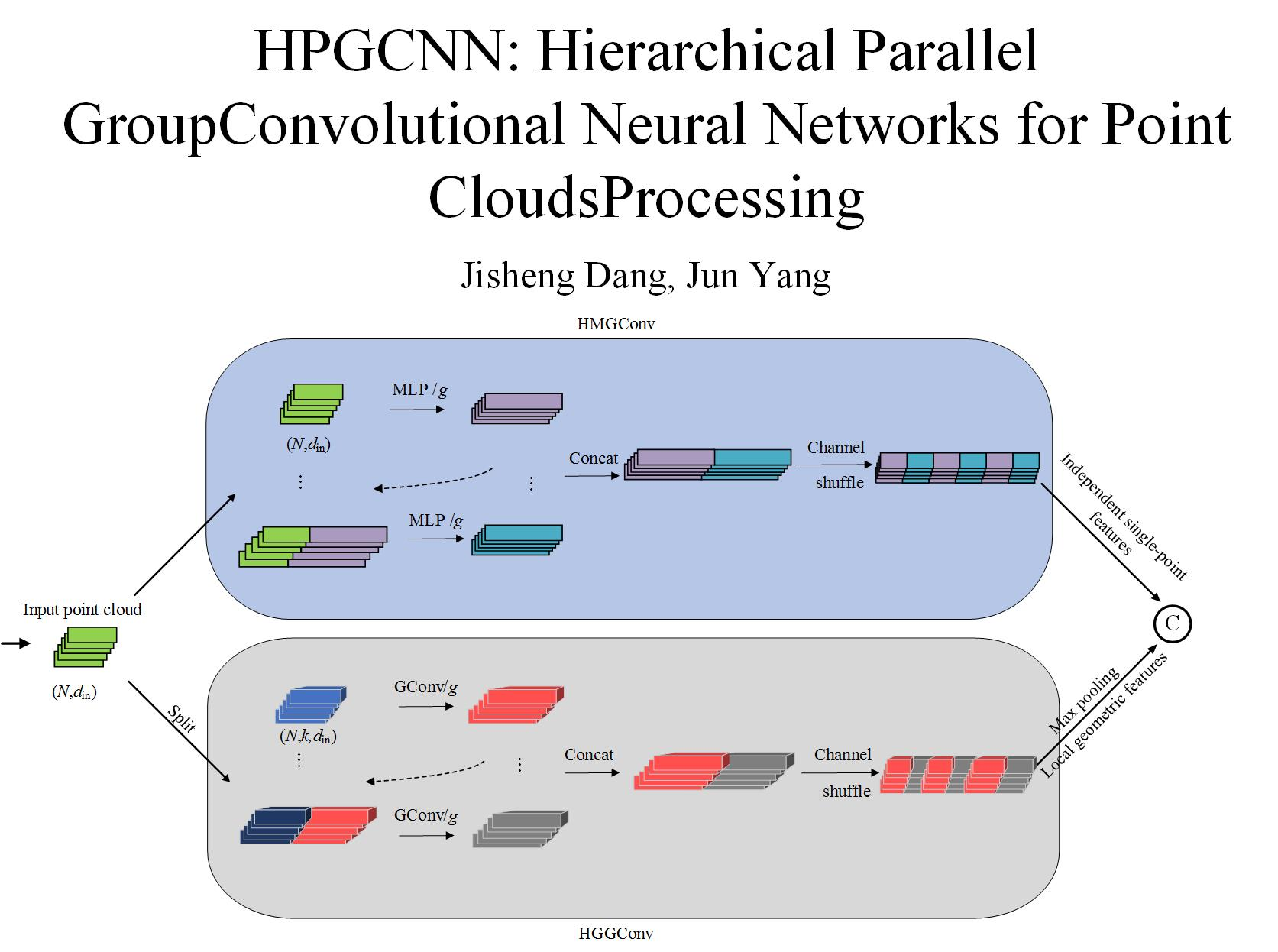
Fast and Differentiable Message Passing on Pairwise Markov Random Fields
Zhiwei Xu (Australian National University)*, Thalaiyasingam Ajanthan (ANU), RICHARD HARTLEY (Australian National University, Australia)
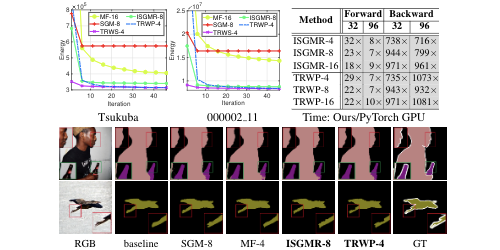