A Day on Campus - An Anomaly Detection Dataset for Events in a Single Camera
Mantini Pranav (University of Houston)*, Li Zhenggang (University of Houston), Shah Shishir K (University of Houston)
Keywords: Datasets and Performance Analysis
Abstract:
Detecting anomalies in videos is a complex problem with a myriad of applications in video surveillance. However, large and complex datasets that are representative of real-world deployment of surveillance cameras are unavailable. Anomalies in surveillance videos are not well defined and the standard and existing metrics for evaluation do not quantify the performance of algorithms accurately. We provide a large scale dataset, A Day on Campus (ADOC), with 25 event types, spanning over 721 instances and occurring over a period of 24 hours. This is the largest dataset with localized bounding box annotations that is available to perform anomaly detection. We design a novel metric to evaluate the performance of methods and we perform an evaluation of the state-of-the-art methods to ascertain their readiness to transition into real-world surveillance scenarios.
SlidesLive
Similar Papers
Video-Based Crowd Counting Using a Multi-Scale Optical Flow Pyramid Network
Mohammad Asiful Hossain (HUAWEI Technologies Co, LTD.)*, Kevin Cannons (Huawei Technologies Canada Co., Ltd ), Daesik Jang (Personal Research), Fabio Cuzzolin (Oxford Brookes University), Zhan Xu (Huawei Canada)
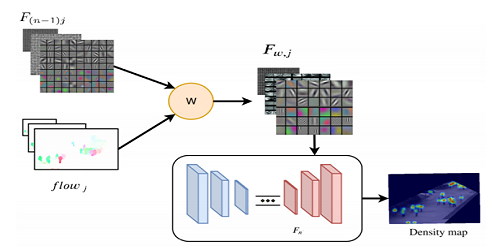
DoFNet: Depth of Field Difference Learning for Detecting Image Forgery
Yonghyun Jeong (Samsung SDS)*, Jongwon Choi (Chung-Ang University), Doyeon Kim (SamsungSDS), Sehyeon Park (Samsung SDS), Minki Hong (Samsung SDS), Changhyun Park (Samsung SDS), Seungjai Min (Samsung SDS), Youngjune Gwon (Samsung SDS)
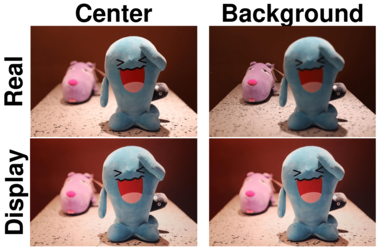
Channel Recurrent Attention Networks for Video Pedestrian Retrieval
Pengfei Fang (The Australian National University)*, Pan Ji (OPPO US Research Center), Jieming Zhou (The Australian National University), Lars Petersson (Data61/CSIRO), Mehrtash Harandi (Monash University)
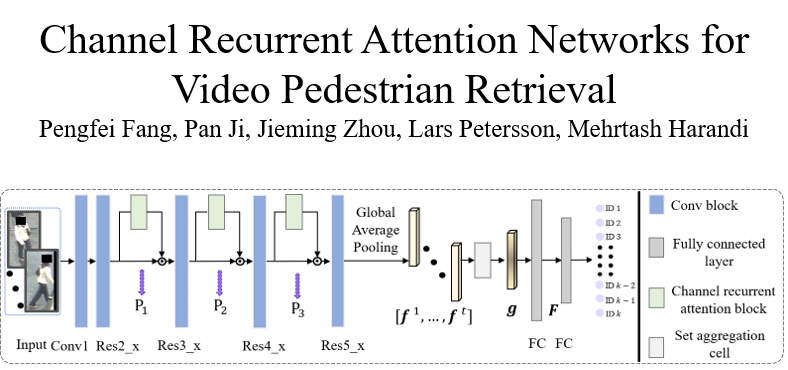