Multi-label X-ray Imagery Classification via Bottom-up Attention and Meta Fusion
Benyi Hu (Xi'an Jiaotong University)*, Chi Zhang (Xi'an Jiaotong Univiersity), Le Wang (Xi'an Jiaotong University), Qilin Zhang (HERE Technologies), Yuehu Liu (Xi'an Jiaotong University)
Keywords: Applications of Computer Vision, Vision for X
Abstract:
Automatic security inspection has received increasing interests in recent years. Due to the fixed top-down perspective of X-ray scanning of often tightly packed luggages, such images typically suffer from penetration-induced occlusions, severe object overlapping and violent changes in appearance. For this particular application, few research efforts have been made. To deal with the overlapping in X-ray images classification, we propose a novel Security X-ray Multi-label Classification Network (SXMNet). Our hypothesis is that different overlapping levels and scale variations are the primary challenges in the multi-label classification problem of prohibited items. To address these challenges, we propose to incorporate 1) spatial attention to locate prohibited items despite shape, color and texture variations; and 2) anisotropic fusion of per-stage predictions to dynamically fuse hierarchical visual information under violent variations. Motivated by these, our SXMNet is boosted by bottom-up attention and neural-guided Meta Fusion. Raw input image is exploited to generate high-quality attention masks in a bottom-up way for pyramid feature refinement. Subsequently, the per-stage predictions according to the refined features are automatically re-weighted and fused via a soft selection guided by neural knowledge. Comprehensive experiments on the Security Inspection X-ray (SIXray) and Occluded Prohibited Items X-ray (OPIXray) datasets demonstrate the superiority of the proposed method.
SlidesLive
Similar Papers
Trainable Structure Tensors for Autonomous Baggage Threat Detection Under Extreme Occlusion
Taimur Hassan (Khalifa University of Science and Technology)*, Naoufel Werghi (Khalifa University of Science and Technology)
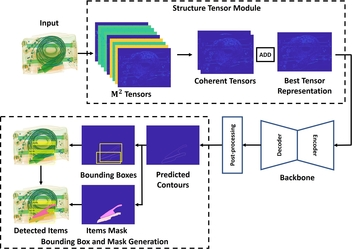
Unified Application of Style Transfer for Face Swapping and Reenactment
Le Minh Ngo (University of Amsterdam)*, Christian aan de Wiel (3DUniversum), Sezer Karaoglu (University of Amsterdam), Theo Gevers (University of Amsterdam)
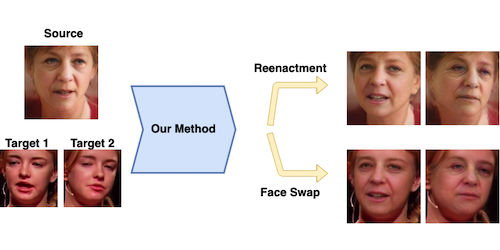
Vax-a-Net: Training-time Defence Against Adversarial Patch Attacks
Thomas Gittings (University of Surrey), Steve Schneider (University of Surrey), John Collomosse (Adobe Research)*
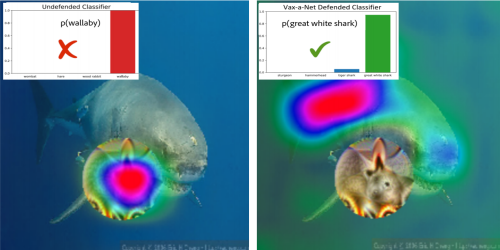