Trainable Structure Tensors for Autonomous Baggage Threat Detection Under Extreme Occlusion
Taimur Hassan (Khalifa University of Science and Technology)*, Naoufel Werghi (Khalifa University of Science and Technology)
Keywords: Applications of Computer Vision, Vision for X
Abstract:
Detecting baggage threats is one of the most difficult tasks, even for expert officers. Many researchers have developed computer-aided screening systems to recognize these threats from the baggage X-ray scans. However, all of these frameworks are limited in identifying the contraband items under extreme occlusion. This paper presents a novel instance segmentation framework that utilizes trainable structure tensors to highlight the contours of the occluded and cluttered contraband items (by scanning multiple predominant orientations), while simultaneously suppressing the irrelevant baggage content. The proposed framework has been extensively tested on four publicly available X-ray datasets where it outperforms the state-of-the-art frameworks in terms of mean average precision scores. Furthermore, to the best of our knowledge, it is the only framework that has been validated on combined grayscale and colored scans obtained from four different types of X-ray scanners.
SlidesLive
Similar Papers
Multi-label X-ray Imagery Classification via Bottom-up Attention and Meta Fusion
Benyi Hu (Xi'an Jiaotong University)*, Chi Zhang (Xi'an Jiaotong Univiersity), Le Wang (Xi'an Jiaotong University), Qilin Zhang (HERE Technologies), Yuehu Liu (Xi'an Jiaotong University)
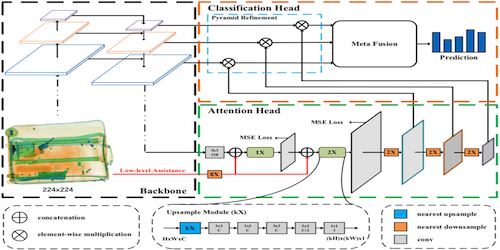
Unified Application of Style Transfer for Face Swapping and Reenactment
Le Minh Ngo (University of Amsterdam)*, Christian aan de Wiel (3DUniversum), Sezer Karaoglu (University of Amsterdam), Theo Gevers (University of Amsterdam)
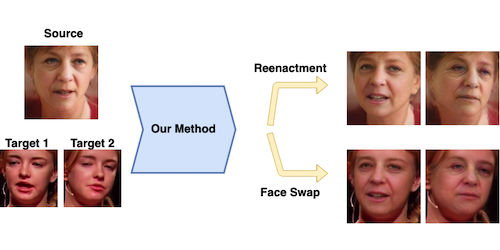
Vax-a-Net: Training-time Defence Against Adversarial Patch Attacks
Thomas Gittings (University of Surrey), Steve Schneider (University of Surrey), John Collomosse (Adobe Research)*
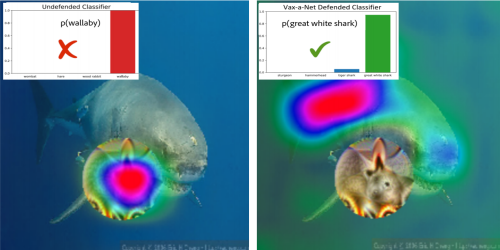