A Global to Local Double Embedding Method for Multi-person Pose Estimation
Yiming Xu (UESTC)*, Jiaxin Li (Beijing Institute of Technology), Yan Ding (Beijing Institute of Technology), Hua-Liang Wei (University of Sheffield)
Keywords: Face, Pose, Action, and Gesture
Abstract:
Multi-person pose estimation is a fundamental and challeng-ing problem to many computer vision tasks. Most existing methods canbe broadly categorized into two classes: top-down and bottom-up meth-ods. Both of the two types of methods involve two stages, namely, persondetection and joints detection. Conventionally, the two stages are imple-mented separately without considering their interactions between them,and this may inevitably cause some issue intrinsically. In this paper, wepresent a novel method to simplify the pipeline by implementing per-son detection and joints detection simultaneously. We propose a DoubleEmbedding (DE) method to complete the multi-person pose estimationtask in a global-to-local way. DE consists of Global Embedding (GE)and Local Embedding (LE). GE encodes different person instances andprocesses information covering the whole image and LE encodes the lo-cal limbs information. GE functions for the person detection in top-downstrategy while LE connects the rest joints sequentially which functionsfor joint grouping and information processing in A bottom-up strategy.Based on LE, we design the Mutual Refine Machine (MRM) to reducethe prediction difficulty in complex scenarios. MRM can effectively re-alize the information communicating between keypoints and further im-prove the accuracy. We achieve state-of-the-art results on benchmarksMSCOCO, MPII and CrowdPose, demonstrating the effectiveness andgeneralization ability of our method.
SlidesLive
Similar Papers
Domain-transferred Face Augmentation Network
Hao-Chiang Shao (Fu Jen Catholic University), Kang-Yu Liu (National Tsing Hua University), Chia-Wen Lin (National Tsing Hua University)*, Jiwen Lu (Tsinghua University)
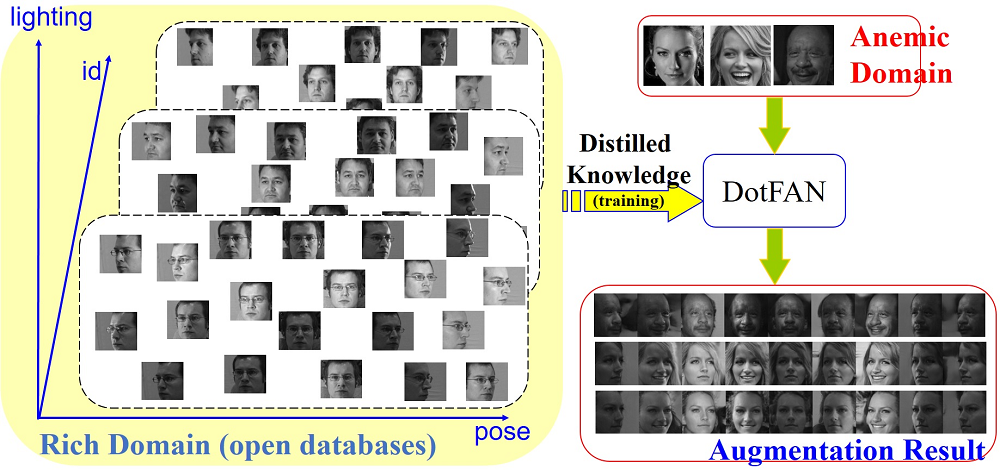
RealSmileNet: A Deep End-To-End Network for Spontaneous and Posed Smile Recognition
Yan Yang (Australian National University)*, Md Zakir Hossain (The Australian National University ), Tom Gedeon (The Australian National University), Shafin Rahman (North South University)
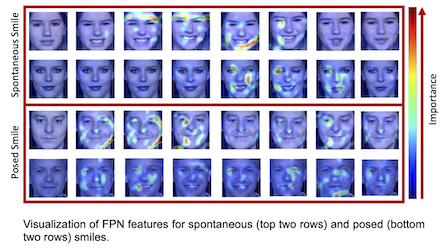
Learning Global Pose Features in Graph Convolutional Networks for 3D Human Pose Estimation
Kenkun Liu ( University of Illinois at Chicago), Zhiming Zou (University of Illinois at Chicago), Wei Tang (University of Illinois at Chicago)*
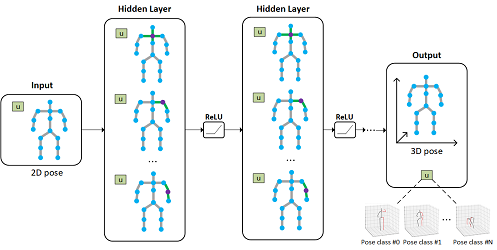