Learning Global Pose Features in Graph Convolutional Networks for 3D Human Pose Estimation
Kenkun Liu ( University of Illinois at Chicago), Zhiming Zou (University of Illinois at Chicago), Wei Tang (University of Illinois at Chicago)*
Keywords: 3D Computer Vision
Abstract:
As the human body skeleton can be represented as a sparse graph, it is natural to exploit graph convolutional networks (GCNs) to model the articulated body structure for 3D human pose estimation (HPE). However, a vanilla graph convolutional layer, the building block of a GCN, only models the local relationships between each body joint and their neighbors on the skeleton graph. Some global attributes, e.g., the action of the person, can be critical to 3D HPE, especially in the case of occlusion or depth ambiguity. To address this issue, this paper introduces a new 3D HPE framework by learning global pose features in GCNs. Specifically, we add a global node to the graph and connect it to all the body joint nodes. On one hand, global features are updated by aggregating all body joint features to model the global attributes. On the other hand, the feature update of each body joint depends on not only their neighbors but also the global node. Furthermore, we propose a heterogeneous multi-task learning framework to learn the local and global features. While each local node regresses the 3D coordinate of the corresponding body joint, we force the global node to classify an action category or learn a low-dimensional pose embedding. Experimental results demonstrate the effectiveness of the proposed approach.
SlidesLive
Similar Papers
Self-Supervised Multi-View Synchronization Learning for 3D Pose Estimation
Simon Jenni (Universität Bern)*, Paolo Favaro (University of Bern)
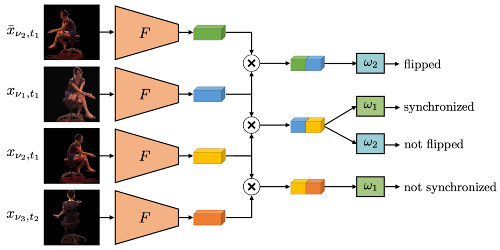
Speech2Video Synthesis with 3D Skeleton Regularization and Expressive Body Poses
Miao Liao (Baidu)*, Sibo Zhang (Baidu), Peng Wang (Baidu USA LLC.), Hao Zhu (Nanjing University), Xinxin Zuo (University of Kentucky), Ruigang Yang (University of Kentucky, USA)
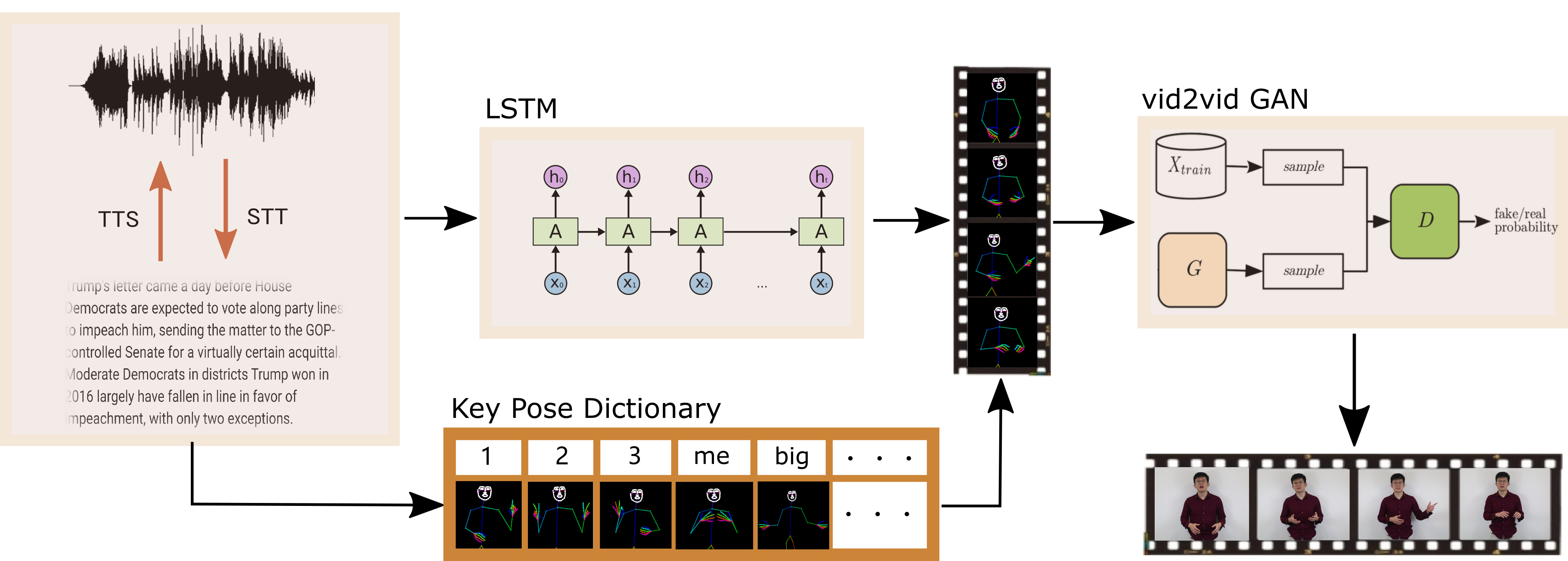
Decoupled Spatial-Temporal Attention Network for Skeleton-Based Action-Gesture Recognition
Lei Shi (Institute of Automation_�Chinese Academy of Sciences )*, Yifan Zhang (Institute of Automation, Chinese Academy of Sciences), Jian Cheng ("Chinese Academy of Sciences, China"), Hanqing Lu (NLPR, Institute of Automation, CAS)
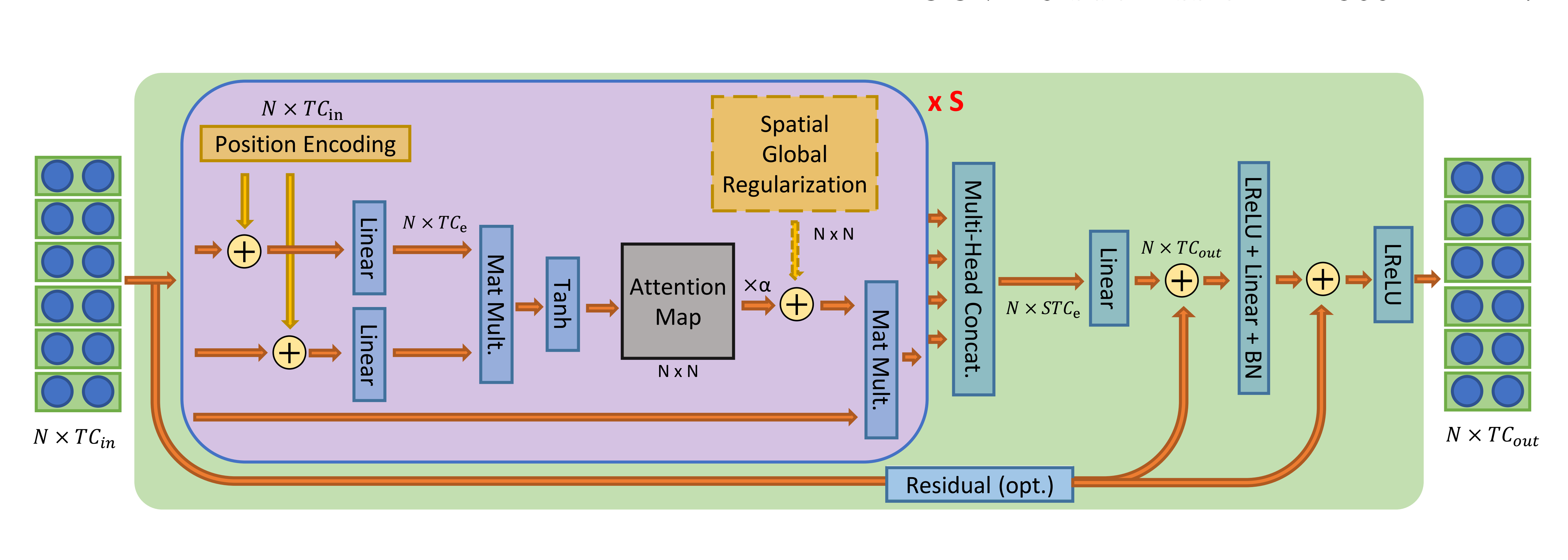