Speech2Video Synthesis with 3D Skeleton Regularization and Expressive Body Poses
Miao Liao (Baidu)*, Sibo Zhang (Baidu), Peng Wang (Baidu USA LLC.), Hao Zhu (Nanjing University), Xinxin Zuo (University of Kentucky), Ruigang Yang (University of Kentucky, USA)
Keywords: Face, Pose, Action, and Gesture
Abstract:
In this paper, we propose a novel approach to convert a given speech audio to a photo-realistic speaking video of a specific person, where the output video has synchronized, realistic and expressive rich body dynamics. We achieve this by first generating 3D skeleton movements from the audio sequence using a recurrent neural network (RNN), and then synthesizing the output video via a conditional generative adversarial network (GAN). To make the skeleton movement realistic and expressive, we embed the knowledge of an articulated 3D human skeleton and a learned dictionary of personal speech iconic gestures into the generation process in both learning and testing pipelines. The former prevents the generation of unreasonable body distortion, while the later helps our model quickly learn meaningful body movement through a few recorded videos. To produce photo-realistic and high-resolution video with motion details, we propose to insert part attention mechanisms in the conditional GAN, where each detailed part, e.g. head and hand, is automatically zoomed in to have their own discriminators. To validate our approach, we collect a dataset with 20 high-quality videos from 1 male and 1 female model reading various documents under different topics. Compared with previous SoTA pipelines handling similar tasks, our approach achieves better results by a user study.
SlidesLive
Similar Papers
Self-Supervised Multi-View Synchronization Learning for 3D Pose Estimation
Simon Jenni (Universität Bern)*, Paolo Favaro (University of Bern)
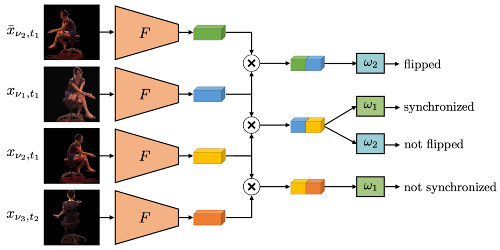
Learning Global Pose Features in Graph Convolutional Networks for 3D Human Pose Estimation
Kenkun Liu ( University of Illinois at Chicago), Zhiming Zou (University of Illinois at Chicago), Wei Tang (University of Illinois at Chicago)*
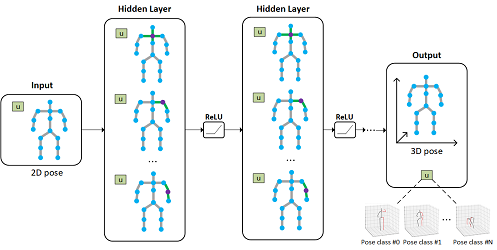
FootNet: An efficient convolutional network for multiview 3D foot reconstruction
Felix Kok (Cambridge University)*, James Charles (Cambridge University), Roberto Cipolla (University of Cambridge)
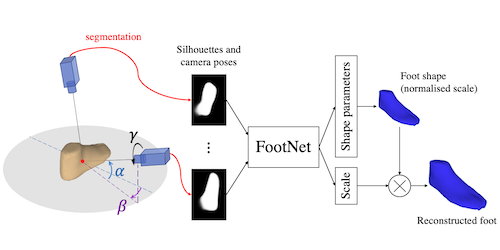