Cascaded Transposed Long-range Convolutions for Monocular Depth Estimation
Go Irie (NTT Corporation)*, Daiki Ikami (NTT Corporation), Takahito Kawanishi (NTT Corporation), Kunio Kashino (NTT Communication Science Laboratories)
Keywords: RGBD and Depth Image Processing
Abstract:
We study the shape of the convolution kernels in the upsampling block for deep monocular depth estimation. First, our empirical analysis shows that the depth estimation accuracy can be improved consistently by only changing the shape of the two consecutive convolution layers with square kernels, e.g., (5 x 5) -> (5 x 5), to two "long-range" kernels, one having the transposed shape of the other, e.g., (1 x 25) -> (25 x 1). Second, based on this observation, we propose a new upsampling block called Cascaded Transposed Long-range Convolutions (CTLC) that uses parallel sequences of two long-range convolutions with different kernel shapes. Experiments with NYU Depth V2 and KITTI show that our CTLC offers higher accuracy with fewer parameters and FLOPs than state-of-the-art methods.
SlidesLive
Similar Papers
Best Buddies Registration for Point Clouds
Amnon Drory (Tel-Aviv University)*, Tal Shomer (Tel-Aviv University), Shai Avidan (Tel Aviv University), Raja Giryes (Tel Aviv University)
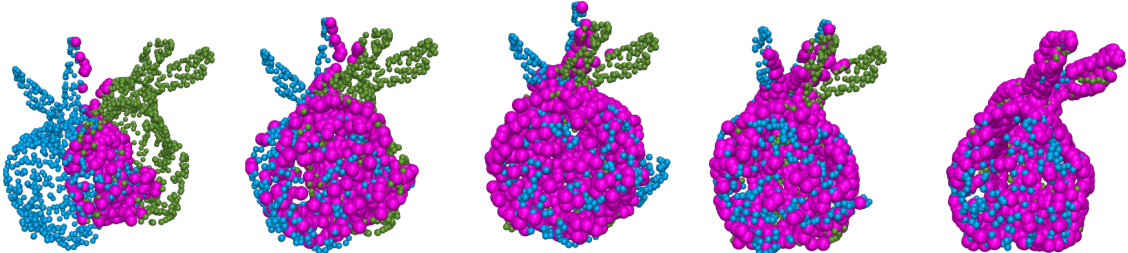
RGB-D Co-attention Network for Semantic Segmentation
Hao Zhou (Harbin Engineering University)*, Lu Qi (The Chinese University of Hong Kong), Zhaoliang Wan (Harbin Engineering University), Hai Huang (Harbin Engineering University), Xu Yang (Chinese Academy of Sciences)
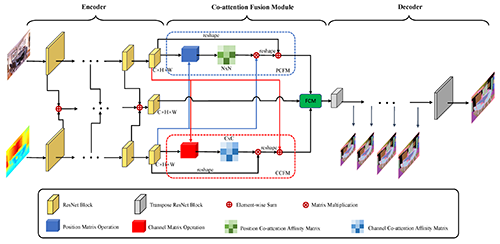
Dense-Scale Feature Learning in Person Re-Identification
Li Wang (Inspur), Baoyu Fan (Inspur Electronic Information Industry Co.,Ltd.)*, Zhenhua Guo (Inspur Electronic Information Industry Co.,Ltd.), Yaqian Zhao (Inspur), Runze Zhang (Inspur Electronic Information Industry Co.,Ltd.), Rengang Li (Inspur), Weifeng Gong ( Inspur Electronic Information Industry Co.,Ltd.)
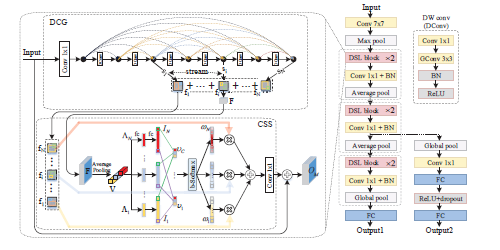