Leveraging Tacit Information Embedded in CNN Layers for Visual Tracking
Kourosh Meshgi (RIKEN AIP)*, Maryam Sadat Mirzaei (Riken AIP / Kyoto University), Shigeyuki Oba (Kyoto University)
Keywords: Motion and Tracking
Abstract:
Different layers in CNNs provide not only different levels of abstraction for describing the objects in the input but also encode various implicit information about them. The activation patterns of different features contain valuable information about the stream of incoming images: spatial relations, temporal patterns, and co-occurrence of spatial and spatiotemporal (ST) features. The studies in visual tracking literature, so far, utilized only one of the CNN layers, a pre-fixed combination of them, or an ensemble of trackers built upon individual layers. In this study, we employ an adaptive combination of several CNN layers in a single DCF tracker to address variations of the target appearances and propose the use of style statistics on both spatial and temporal properties of the target, directly extracted from CNN layers for visual tracking.Experiments demonstrate that using the additional implicit data of CNNs significantly improves the performance of the tracker. Results demonstrate the effectiveness of using style similarity and activation consistency regularization in improving its localization and scale accuracy.
SlidesLive
Similar Papers
Depth-Adapted CNN for RGB-D cameras
Zongwei WU (Univ. Bourgogne Franche-Comte, France)*, Guillaume Allibert (Université Côte d’Azur, CNRS, I3S, France ), Christophe Stolz (Univ. Bourgogne Franche-Comte, France), Cedric Demonceaux (Univ. Bourgogne Franche-Comte, France)
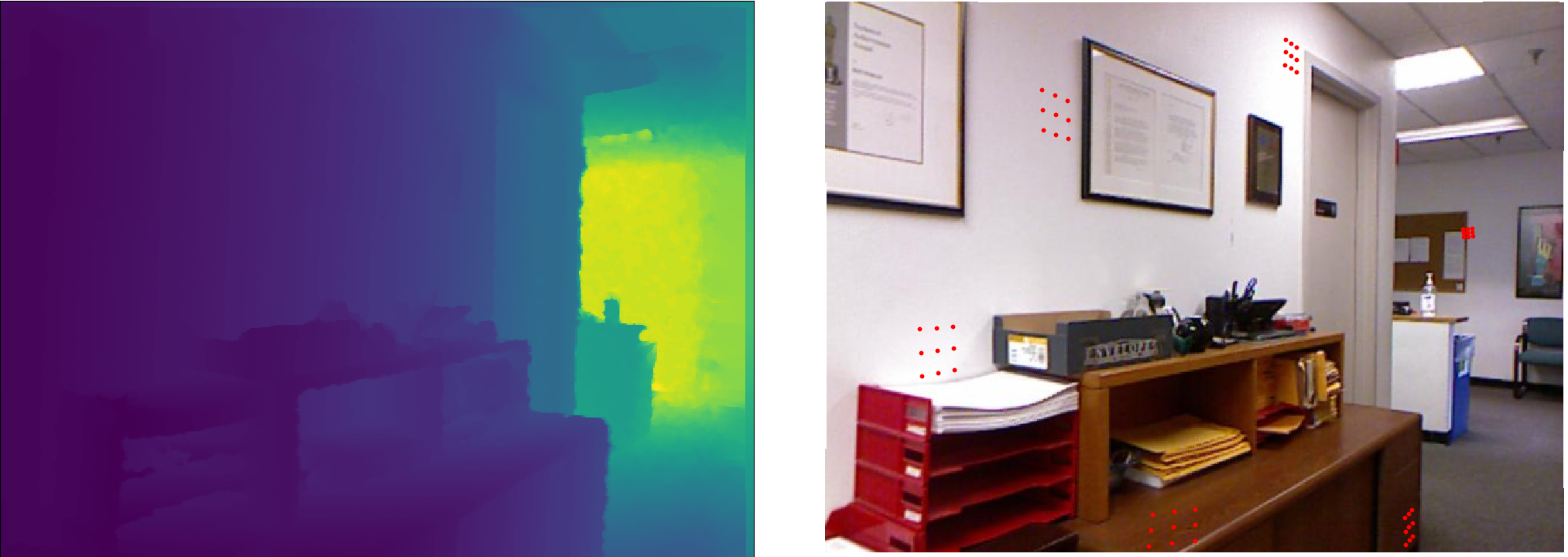
Frequency Attention Network: Blind Noise Removal for Real Images
Hongcheng Mo (Shanghai Jiao Tong University), Jianfei Jiang (Shanghai Jiao Tong University), Qin Wang (Shanghai Jiao Tong University)*, Dong Yin (Fullhan), Pengyu Dong (Fullhan), Jingjun Tian (Fullhan)
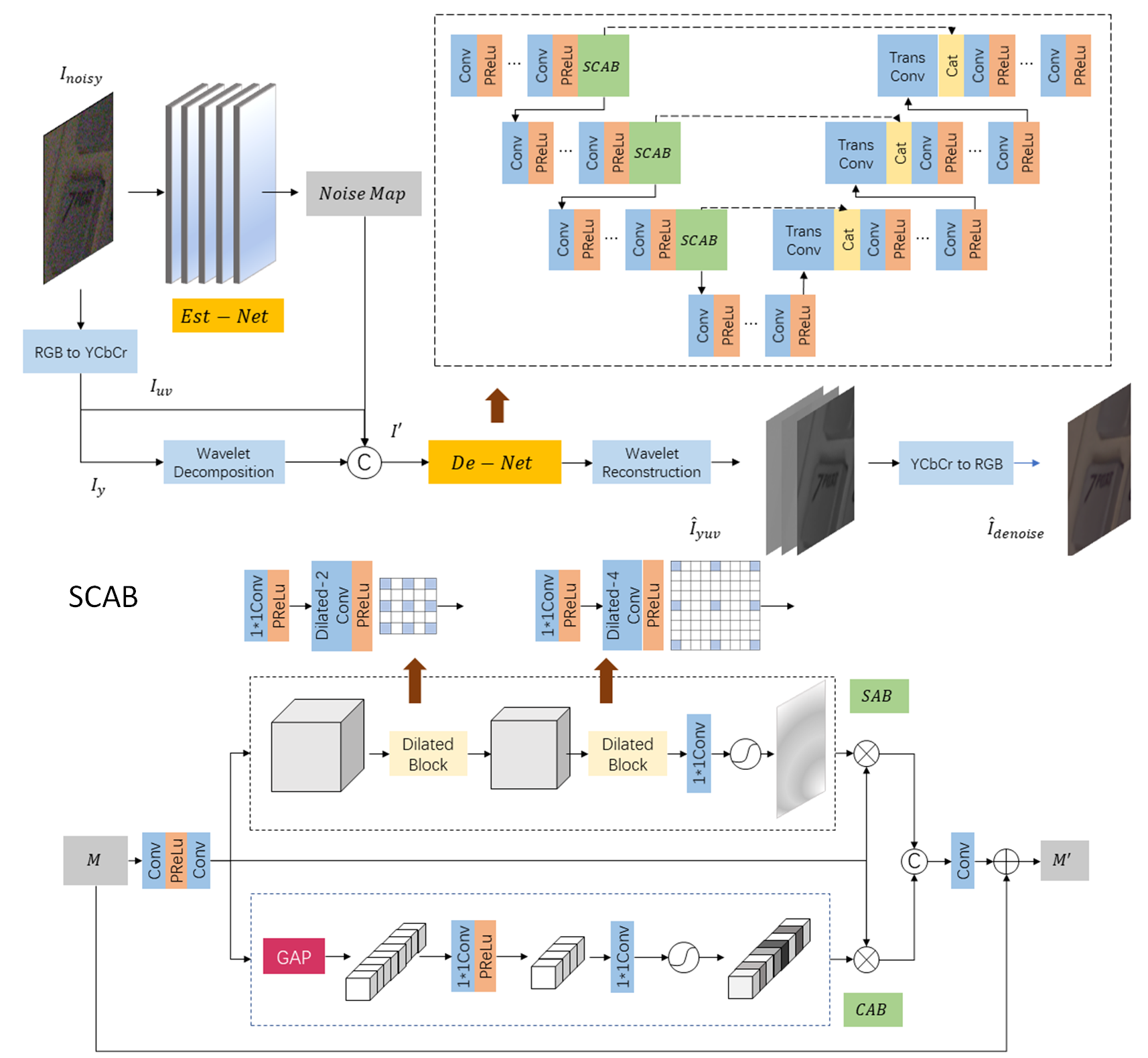
Color Enhancement using Global Parameters and Local Features Learning
Enyu Liu (Tencent)*, Songnan Li (Tencent), Shan Liu (Tencent America)
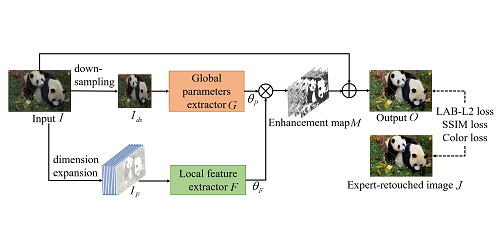