Goal-GAN: Multimodal Trajectory Prediction Based on Goal Position Estimation
Patrick Dendorfer (TUM)*, Aljosa Osep (TUM Munich), Laura Leal-Taixé (TUM)
Keywords: Motion and Tracking
Abstract:
In this paper, we present Goal-GAN, an interpretable and end-to-end trainable model for human trajectory prediction. Inspired by human navigation, we model the task of trajectory prediction as an intuitive two-stage process: (i) goal estimation, which predicts the most likely target positions of the agent, followed by a (ii) routing module which estimates a set of plausible trajectories that route towards the estimated goal. We leverage information about the past trajectory and visual context of the scene to estimate a multi-modal probability distribution over the possible goal positions, which is used to sample a potential goal during the inference. The routing is governed by a recurrent neural network that reacts to physical constraints in the nearby surroundings and generates feasible paths that route towards the sampled goal. Our extensive experimental evaluation shows that our method establishes a new state-of-the-art on several benchmarks while being able to generate a realistic and diverse set of trajectories that conform to physical constraints.
SlidesLive
Similar Papers
OpenTraj: Assessing Prediction Complexity in Human Trajectories Datasets
Javad Amirian (Inria, Rennes, France)*, Bingqing Zhang (UCL), Francisco Valente Castro (Cimat), Juan Jose Baldelomar (Cimat), Jean-Bernard Hayet (CIMAT), Julien Pettré (INRIA Rennes - Bretagne Atlantique)
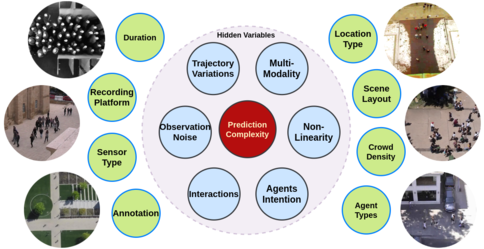
Second Order enhanced Multi-glimpse Attention in Visual Question Answering
Qiang Sun (Fudan University)*, Binghui Xie (Fudan University), Yanwei Fu (Fudan University)
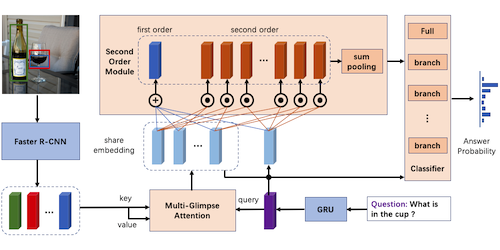
Sequential View Synthesis with Transformer
Phong Nguyen-Ha (University of Oulu)*, Lam Huynh ( University of Oulu), Esa Rahtu (Tampere University), Janne Heikkila (University of Oulu, Finland)
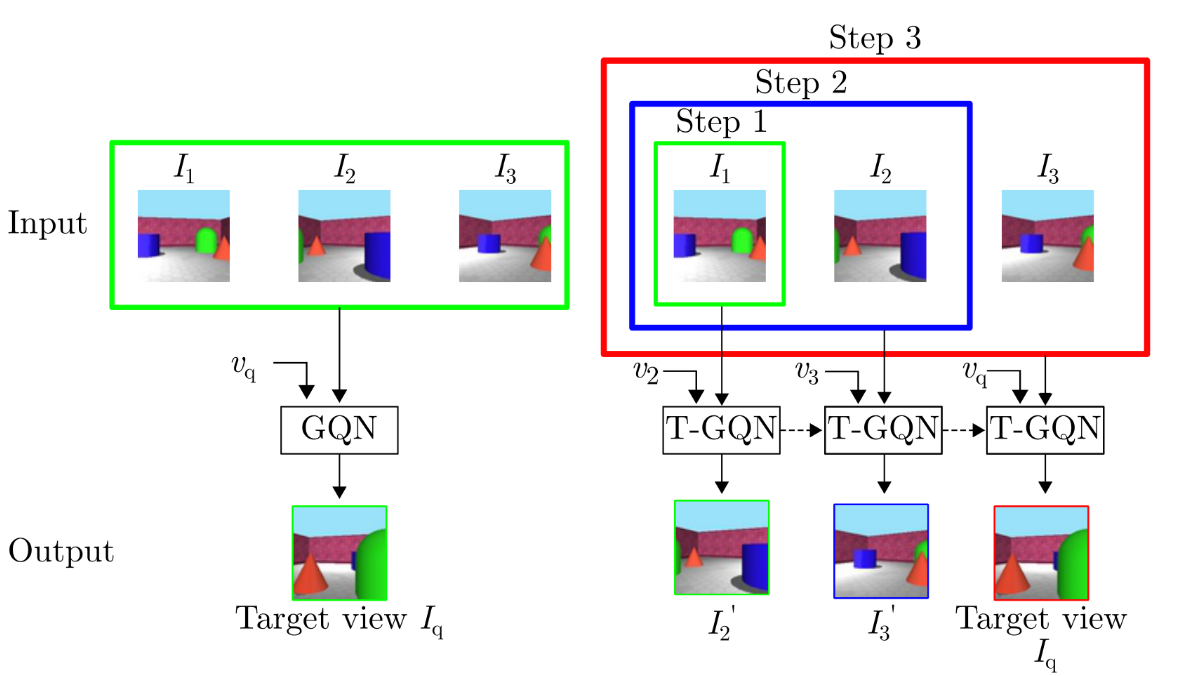