MagGAN: High-Resolution Face Attribute Editing with Mask-Guided Generative Adversarial Network
Yi Wei (University at Albany - SUNY)*, Zhe Gan (Microsoft), Wenbo Li (Samsung Research America), Siwei Lyu (University at Albany), Ming-Ching Chang (University at Albany - SUNY), Lei Zhang (Microsoft), Jianfeng Gao (Microsoft Research), Pengchuan Zhang (Microsoft Research AI)
Keywords: Generative models for computer vision
Abstract:
We present Mask-guided Generative Adversarial Network (MagGAN) for high-resolution face attribute editing, in which semantic facial masks from a pre-trained face parser are used to guide the finegrained image editing process. With the introduction of a mask-guided reconstruction loss, MagGAN learns to only edit the facial parts that are relevant to the desired attribute changes, while preserving the attributeirrelevant regions (e.g., hat, scarf for modification ‘To Bald�_. Further, a novel mask-guided conditioning strategy is introduced to incorporate the influence region of each attribute change into the generator. In addition, a multi-level patch-wise discriminator structure is proposed to scale our model for high-resolution (1024 �_1024) face editing. Experiments on the CelebA benchmark show that the proposed method significantly outperforms prior state-of-the-art approaches in terms of both image quality and editing performance.
SlidesLive
Similar Papers
End-to-end Model-based Gait Recognition
Xiang Li (Nanjing University of Science and Technology)*, Yasushi Makihara ("""Osaka University, Japan"""), Chi Xu (Nanjing University of Science and Technology), Yasushi Yagi (Osaka University), Shiqi Yu (Southern University of Science and Technology, China), Mingwu Ren (Nanjing University of Science and Technology)
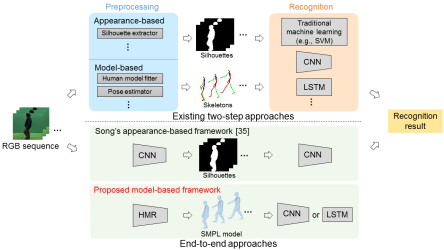
Domain-transferred Face Augmentation Network
Hao-Chiang Shao (Fu Jen Catholic University), Kang-Yu Liu (National Tsing Hua University), Chia-Wen Lin (National Tsing Hua University)*, Jiwen Lu (Tsinghua University)
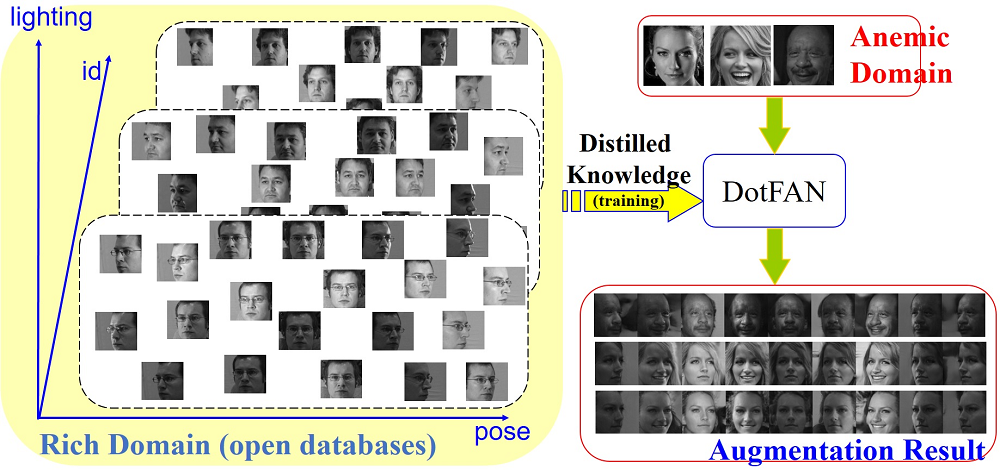
CPTNet: Cascade Pose Transform Network for Single Image Talking Head Animation
Jiale Zhang (Huazhong University of Science and Technology), Ke Xian (Huazhong University of Science and Technology), Chengxin Liu (Huazhong University of Science and Technology)*, Yinpeng Chen (Huazhong University of Science and Technology), Zhiguo Cao (Huazhong Univ. of Sci.&Tech.), Weicai Zhong (Huawei CBG Consumer Cloud Service Big Data Platform Dept.)
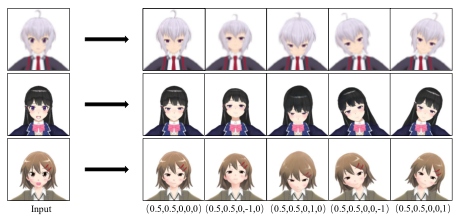